Computer Vision and the Buyer's Journey
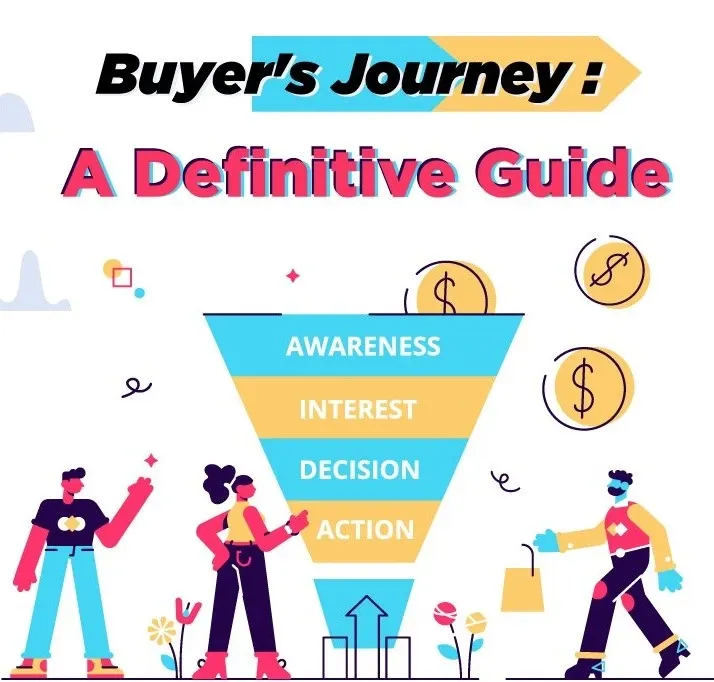
Table of Contents
Introduction
The buyer's journey is an important part of this advanced type of marketing since it allows firms to assist their clients by delivering educational content that helps them understand their problem and the possibilities for fixing it.
One big issue with traditional marketing is that it was not intended to benefit people.
Outbound marketing employs attention-grabbing strategies that inform people of their needs.
It does not add value by teaching people, like inbound marketing does.Here presenting you some stats!
71% of buyers and sellers begin with a basic search instead of searching for a specific brand.
65% of visitors spent a minimum of 16 minutes comparing products ahead of a decision-stage buy.
According to a DemandGen research, 61% of respondents picked vendors who provide material that fits every phase of the sales process.
52% participants watched at least eight pieces of material from the best provider.
86% of senior-level marketers believe it is vital to build a cohesive buyer journey.
Visitors guided by customised content boost sales opportunities by more than 20%.
According to Forrester.com, by 2020, 80% of the buying process will take place without direct human interaction.
Lets discuss each part in depth!
Buyer’s Journey
The buyer's journey is a conceptual framework that describes the system people go through when making a purchase decision.
It outlines the various stages that clients normally develop through the preliminary awareness of a need or problem to the final decision to buy a product or service.
The buyer's journey usually consists of three main stages:
Awareness Stage
- At this stage, clients end up being aware of what they want or problems they've.
- They begin to recognize signs and symptoms or troubles that prompt them to seek data or answers.
- During this segment, clients can also conduct research, collect information, and discover numerous options to better recognize their problem or want.
Consideration Stage:
- In the consideration stage, clients have recognized and defined their trouble or need.
- They begin to compare special alternatives and solutions available to them.
- This stage includes evaluating products or services, weighing the pros and cons, and assessing which options pleasantly cope with their specific necessities or alternatives.
Decision Stage:
- The choice stage is where customers make their final choice and take motion to buy a product or services.
- At this point, customers have narrowed down their options and are geared up to choose.
- Factors including pricing, functions, evaluations, and brand popularity can also have an effect on their final purchase decision.
Why Computer Vision?
Imagine Bob a retailer facing a challenge that seemed to grow in size by the day.
Bob detected a concerning trend among his clients.
They frequently looked through his varied collection of things from one shelf to the next.
The issue was the lack of clarity, which confused the customer experience.
Although Bob was proud to have a variety of goods, customers often had difficulties limiting down their choices to the specific item they were searching for.
The absence of personalised suggestion left many feeling confused about where to go for help or suggestions.
Here comes the computer vision, a sign of hope for Bob.
Bob saw the integration of computer vision technology within the store as a solution that would enhance the consumer experience.
Customers could just take a picture of an item they wanted, and the computer vision system will instantly locate similar things in Bob's inventory.
As users began their path of research, AI algorithms would analyse their browsing patterns and interests in real time, providing personalised suggestions specific to their desires.
Customers would feel at ease to traverse Bob's store with trust and clarity due to computer vision.
Furthermore, augmented reality applications will take buyers in a world of limitless possibilities, allowing them to see how things would look in their homes before purchasing.
Whether they were considering a new piece of furniture or playing with a bold new style, the immersive experiences made possible by computer vision would provide pleasure and ignite ideas.
With computer vision at his fingertips, Bob's Shop evolved into a place of curiosity and fulfilment.
Customers no longer felt lost in the maze of options; rather, they were embraced by the comfort of personalised assistance and the promise of limitless possibilities.
Building the Model
1. Data Collection and Preprocessing
- Collect a diverse dataset of product images from various sources, including e-commerce websites, social media platforms, and in-house product catalogs.
- Label images with relevant metadata such as product category, color, style, and other attributes.
- One can use user friendly tools like Labellerr for annotation process.
- Resize images to a consistent resolution, normalize pixel values, and augment the dataset to increase diversity and robustness.
2. Model Architecture
- Design a CNN architecture for image feature extraction and representation learning.
- Utilize pre-trained CNN models such as VGG, ResNet, or EfficientNet as feature extractors to leverage learned representations for downstream tasks.
- Fine-tune the pre-trained CNN on the specific task of product recognition, recommendation, or augmented reality.
3. Integration into the Buyer's Journey
Awareness Stage
- Train a model for image similarity search using techniques like Siamese networks or triplet loss.
- Develop a visual search engine that computes similarity scores between query images and product images in the database.
Consideration Stage
- Build a recommendation system using collaborative filtering or content-based filtering techniques.
- Extract image embeddings from the CNN model and calculate similarity scores between products to generate personalized recommendations.
- Integrate the recommendation model into e-commerce platforms or mobile applications to provide real-time product suggestions based on user interactions.
Decision Stage
- Develop an AR application for visualizing products in real-world environments.
- Train models for object detection and pose estimation to accurately overlay virtual objects onto scenes.
- Deploy the AR application on mobile devices, allowing users to interactively preview furniture, apparel, or accessories before making purchase decisions.
Post-Purchase Stage
- Develop models for image and video analytics to extract insights from consumer-generated content.
- Perform sentiment analysis on visual content to understand consumer opinions and preferences.
- Apply clustering or trend detection algorithms to identify emerging patterns and market trends.
- Integrate visual analytics tools into business intelligence platforms for monitoring consumer engagement and market trends.
Evaluation and Optimization
- Evaluate model performance using metrics such as accuracy, precision, recall, and F1 score for classification tasks, and mean average precision (mAP) for object detection.
- Conduct A/B tests to measure the impact of computer vision integration on user engagement, conversion rates, and revenue.
- Continuously monitor model performance and collect feedback data to iteratively improve the accuracy and relevance of recommendations and visual experiences.
Deployment
When deploying a computer vision model for enhancing the buyer's journey, several key considerations come into play
- Infrastructure: Deploy scalable and reliable infrastructure to accommodate varying workloads and ensure optimal performance.
- Integration: Design APIs and data pipelines for seamless integration with existing systems and workflows.
- Security: Implement encryption, access controls, and model security measures to protect sensitive data and prevent unauthorized access.
- Monitoring and Maintenance: Set up monitoring systems to track performance metrics, detect anomalies, and automate remediation processes.
- User Experience: Provide comprehensive documentation, user support, and feedback mechanisms to enhance usability and user satisfaction.Continuous
- Improvement: Embrace iterative development and version control to continuously improve and enhance deployed models over time.
Challenges
- Data Quality and Diversity: Accessing diverse, high-quality datasets is essential for training robust computer vision models.
- Scalability: Deploying computer vision models at scale demands robust infrastructure and resource management to handle fluctuating workloads.
- Model Interpretability: Enhancing interpretability of computer vision models is crucial for building trust and understanding among users and stakeholders.
- Security and Privacy: Protecting sensitive data and ensuring compliance with privacy regulations are paramount when deploying computer vision applications.
- Bias and Fairness: Mitigating bias and promoting fairness in computer vision models requires proactive strategies to address biases in training data and algorithms.
- Maintenance and Updates: Continuous monitoring and maintenance are necessary to address performance degradation and evolving user needs in deployed models.
- User Experience and Adoption: Promoting user adoption relies on intuitive interfaces, comprehensive documentation, and responsive support channels to educate users about the benefits of computer vision technology.
Conclusion
Deploying computer vision models to improve the buyer's journey brings both opportunities and challenges to businesses.
Organisations may fully utilise computer vision technology by using high-quality data, scalable infrastructure, and proactive methods for model interpretability, security, fairness, maintenance, and user experience.
Addressing these difficulties necessitates a comprehensive approach that encourages collaboration, creativity, and continual development among many teams and stakeholders.
Finally, by overcoming these obstacles, businesses may provide transformative experiences that promote meaningful engagement, foster trust, and accelerate growth in today's dynamic economy.
Frequently Asked Questions
Q1. What is computer vision in shopping malls?
Computer Vision can track client movements and behaviours within a store while protecting their privacy. This not only gives retailers insight into which products are most popular, but it can also help them make inventory decisions and shop layout changes.
Q2.How does computer vision help in customer support?
Computer vision can use visual data to add important information to a customer's profile, such as the customer's face profile, home setting, car, purchased equipment, invoices, contracts, and so on. It can also assist predict difficulties before they occur, helping a customer service team to avoid discontent or attrition.
Book our demo with one of our product specialist
Book a Demo