How Human-in-the-Loop is used in Data Annotation?
Human-in-the-Loop (HITL) annotation blends AI automation with human expertise to improve data labeling accuracy. It ensures AI models receive high-quality training data, reduces errors, and enhances real-world performance, making it essential for AI development across various industries.
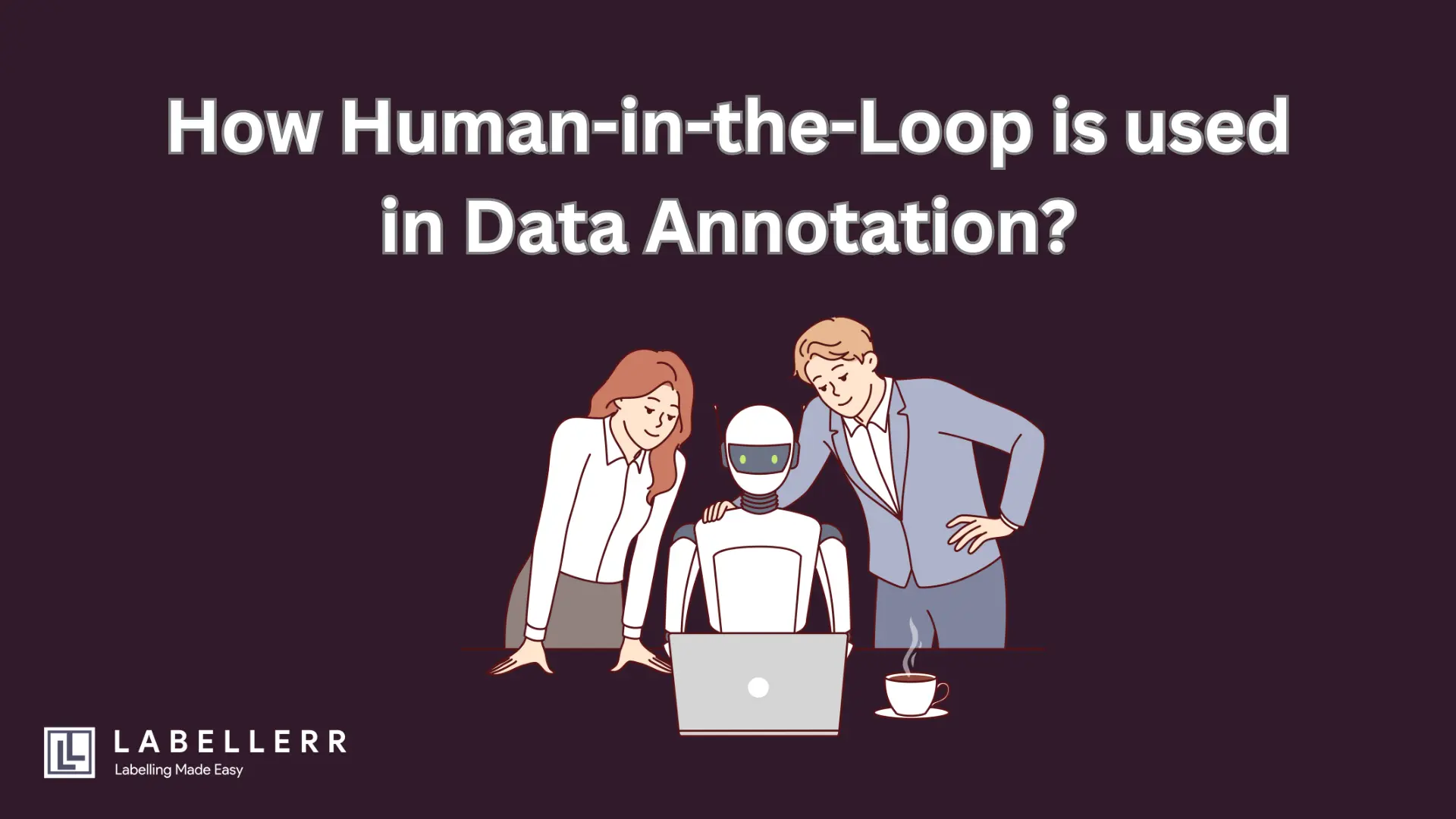
Artificial intelligence (AI) and machine learning (ML) models rely on high-quality labeled data to function effectively. Annotation software plays a key role in labeling images, text, video, and audio, making AI training possible.
While automated softwares have significantly improved efficiency, human involvement remains essential in maintaining accuracy, handling complex cases, and reducing bias in AI models.
The approach that combines automation with human expertise is called human-in-the-loop (HITL) annotation. It ensures that AI models receive precisely labeled data, leading to better decision-making and higher accuracy.
This article explores the importance of human-in-the-loop annotation, how it works, and why it remains a crucial step in AI development.
Table of Contents
- What Is Human-in-the-Loop Annotation?
- Why Is Human-in-the-Loop Annotation Important?
- How Human-in-the-Loop Annotation Works?
- When Is Human-in-the-Loop Annotation Needed?
- Human-in-the-Loop vs. Fully Automated Annotation
- The Future of Human-in-the-Loop Annotation
- Conclusion
- FAQs
What Is Human-in-the-Loop Annotation?
Human-in-the-loop (HITL) annotation is a process where AI-assisted labeling is combined with human review and correction.
Instead of relying entirely on automation, human annotators verify, refine, and correct AI-generated labels to ensure high-quality training data.
This approach helps AI models learn from mistakes and continuously improve. It is particularly useful for complex datasets, such as medical images, financial documents, and self-driving car data, where errors in annotation can lead to critical failures in AI performance.
Why Is Human-in-the-Loop Annotation Important?
- Ensuring High Annotation Accuracy
AI-powered annotation tools can make errors, especially in ambiguous or complex cases. Human review corrects these mistakes, ensuring that AI models receive precise training data. - Handling Complex and Subjective Data
Some types of data require human judgment to interpret correctly. For example:- Medical images need human expertise to correctly label tumors, fractures, or organ structures.
- Sentiment analysis in NLP requires understanding of tone and context.
- Self-driving datasets must accurately distinguish between pedestrians, cyclists, and vehicles.
- Reducing Bias in AI Models
AI models trained on biased data can reinforce discrimination in real-world applications. Human annotators can identify and correct biased labels, ensuring that AI makes fairer and more ethical decisions. - Training AI for Better Performance
AI learns from human corrections, improving its ability to label data more accurately over time. This iterative learning process makes AI models smarter and more efficient, reducing future reliance on manual corrections.
How Human-in-the-Loop Annotation Works?
The HITL process involves three main steps:
1. AI Generates Initial Annotations
- AI-powered software automatically labels data based on pre-trained models.
- The model applies bounding boxes, segmentation, or text tagging to datasets.
2. Human Review and Corrections
- Human annotators review AI-generated labels and fix errors to ensure accuracy.
- Annotators also provide feedback to improve AI predictions in future labeling tasks.
3. AI Model Learns and Improves
- AI incorporates human feedback into its learning process.
- Over time, the model reduces errors and becomes more independent, requiring less manual intervention.
This approach makes AI annotation systems faster and more accurate while maintaining high data quality.
When Is Human-in-the-Loop Annotation Needed?
HITL annotation is crucial in industries where high accuracy and precision are essential. Some key applications include:
- Healthcare – Labeling X-rays, MRIs, and CT scans for disease detection.
- Autonomous Vehicles – Verifying object detection in self-driving datasets.
- Retail & E-Commerce – Improving product recommendation systems through accurate tagging.
- Finance & Banking – Reviewing fraud detection models and transaction analysis.
- NLP & Chatbots – Refining text-based AI models for sentiment analysis and language translation.
Human-in-the-Loop vs. Fully Automated Annotation
Accuracy: Ensuring Data Quality
- Human-in-the-Loop Annotation: AI labels the data, and humans review, correct, and refine it for higher accuracy.
- Fully Automated Annotation: AI labels data without human intervention, but it may mislabel complex or ambiguous data.
- HITL annotation is crucial for critical applications like healthcare and autonomous driving.
- Fully automated annotation works well for structured, repetitive datasets with minimal complexity.
Efficiency: Speed vs. Quality
- Human-in-the-Loop Annotation: Slower than automation but ensures quality and reduces rework.
- Fully Automated Annotation: Faster and scalable but may require human correction later.
- HITL annotation is essential for AI models where errors can cause significant problems.
- Fully automated annotation is useful for high-volume, low-risk labeling tasks.
When to Use HITL or Fully Automated Annotation
- Human-in-the-Loop Annotation is best for:
- Healthcare: Medical imaging and diagnosis.
- Autonomous vehicles: Object detection and pedestrian tracking.
- Financial services: Fraud detection and regulatory compliance.
- Legal and compliance: Reviewing AI-generated legal document summaries.
- Fully Automated Annotation is best for:
- E-commerce: Product categorization and recommendations.
- Social media and marketing: Sentiment analysis and content tagging.
- Retail and inventory management: Automated stock tracking and labeling.
- Basic image recognition: Identifying simple objects in structured datasets.
Finding the Right Balance
- Fully automated annotation is ideal for large-scale, structured datasets where minor errors are acceptable.
- Human-in-the-loop annotation is necessary for complex tasks that require high accuracy.
- A hybrid approach combining both methods is often the best solution, balancing speed and quality.
The Future of Human-in-the-Loop Annotation
With advancements in AI, annotation software will become smarter and more efficient, but human oversight will remain essential. Future trends include:
- Smarter AI Models – AI will learn faster from human corrections, requiring less intervention over time.
- Real-Time HITL Annotation – AI-assisted human review will become faster and more seamless, allowing real-time data labeling.
- AI-Powered Quality Control – AI will proactively highlight mislabeled data for human review, streamlining the annotation workflow.
Labellerr integrates human-in-the-loop workflows, ensuring high-quality AI training data for various industries.
Conclusion
Human-in-the-loop annotation combines AI efficiency with human expertise, making AI models more accurate, reliable, and unbiased.
While automation speeds up the annotation process, human validation remains essential for handling complex data, reducing biases, and ensuring high-quality AI training.
As AI technology evolves, businesses must balance automation and human oversight to build trustworthy AI systems. Investing in the right software with human-in-the-loop capabilities will be key to developing ethical and high-performance AI applications.
FAQs
What is Human-in-the-Loop (HITL) annotation in AI?
Human-in-the-Loop annotation combines human expertise with AI automation to improve data labeling accuracy, ensuring better model performance.
Why is Human-in-the-Loop annotation important for AI development?
HITL helps refine AI models by correcting errors, handling complex cases, and ensuring high-quality labeled data for training machine learning algorithms.
How does Human-in-the-Loop annotation improve AI accuracy?
By allowing human reviewers to validate and refine AI-generated labels, HITL reduces errors and enhances the reliability of AI models in real-world applications.
Book our demo with one of our product specialist
Book a Demo