Learn How This Research Organization Automated Quality Control With Labellerr
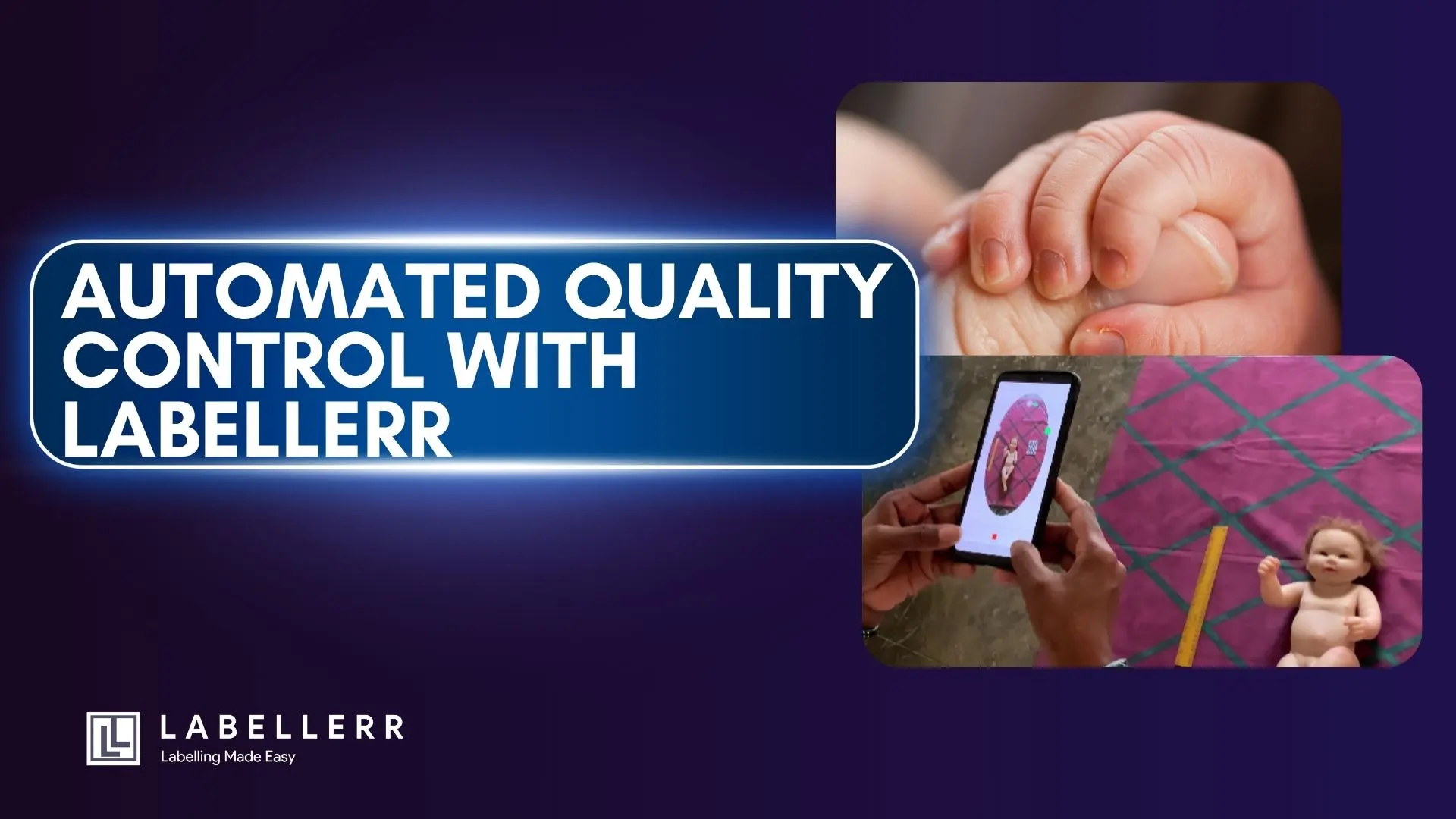
Accurately identifying low birth weight (LBW) babies is a critical challenge in regions with limited healthcare infrastructure.
According to UNICEF, 50% of LBW babies, about 10 million annually go undetected, increasing risks of developmental disorders and neonatal mortality. Timely identification can significantly improve their quality of life through appropriate care.
To address this, a customer developed an AI-powered mobile app to estimate newborn weight from images. Labellerr streamlined the annotation process, achieving a 70% reduction in annotation time, enabling accurate 3D modeling and enhancing AI model efficiency for weight estimation.
Labellerr provided the essential tools and expertise to streamline their quality control process, enhancing the efficiency and accuracy of their AI model.
About the Customer
Wadhwani Institute for Artificial Intelligence is a nonprofit research organization dedicated to using AI to address critical global challenges. Their mission is to use AI technologies, such as computer vision, cognitive reasoning, and IoT, to improve healthcare, education, agriculture, and public infrastructure.
They collaborate with leading academic institutions, international organizations, and governments to develop innovative AI solutions that reflect meaningful change.
Problems and Challenges
They faced several challenges in developing their AI mobile app for estimating newborn weight:
- Data Volume: Annotating tens of thousands of newborn images was required to create a precise 3D model.
- Varied Conditions: Images were captured in diverse conditions, such as poor lighting, awkward angles, and inconsistent backgrounds.
- Time Constraints: Manual annotation was time-consuming, delaying the model development process.
- Accuracy: Precise annotations were critical for ensuring the AI model accurately correlated visual patterns with weight estimates.
How Labellerr Stepped In
Labellerr’s advanced annotation platform provided the ideal solution for Wadhwani’s needs. Here’s how Labellerr helped automate and enhance quality control:
- Efficient Object Classification:
- Applied over 25 specific tags for accurate object classification.
- Enabled detailed annotation necessary for 3D modeling of newborn images.
- Time-Saving Automation:
- Saved over 70% of annotation time by copying relevant tags based on image similarity.
- Reduced manual workload through automated tagging processes.
- Quality Control Mechanisms:
- Implemented a two-tier review process involving external annotation workers and internal teams.
- Provided a quick review mechanism to verify annotation accuracy and ensure consistency.
- Consensus-Based Tagging:
- Used inter-annotator agreement to improve accuracy and minimize errors.
- Enhanced reliability of annotations with automated quality checks.
- Seamless Integration:
- Connected Labellerr’s platform to the client’s cloud storage, ensuring smooth data transfer and project scalability.
Results and Impacts
With Labellerr's support, the Wadhwani Institute achieved:
- Enhanced Model Accuracy:
- Improved correlation between visual patterns and newborn weight estimation.
- Increased Efficiency:
- 70% reduction in annotation time, accelerating model development.
- Reliable 3D Model Creation:
- Accurate annotations enabled the development of a precise 3D model essential for the AI app.
- Scalable Quality Control:
- Efficient review and tagging processes ensured consistent, high-quality annotations.
This collaboration empowered Wadhwani to deliver an AI solution with superior performance and precision.
Conclusion
Labellerr’s automated annotation and quality control capabilities significantly enhanced Wadhwani’s ability to develop a reliable AI model for newborn weight estimation.
By streamlining annotation processes, improving accuracy, and saving time, Labellerr helped transform its vision into a successful AI-powered solution.
Need help automating your annotation and quality control processes? Contact Labellerr today to see how we can support your AI development journey!
FAQs
- What types of tags were used in the annotation process?
Labellerr applied over 25 specific tags to classify newborn images accurately, focusing on body parts, angles, and lighting conditions. - How did Labellerr reduce annotation time by 70%?
Labellerr’s platform automated repetitive tasks by copying relevant tags based on image similarity, significantly reducing manual effort. - How did Labellerr ensure annotation quality?
Labellerr implemented a two-tier review process and used consensus-based tagging to verify accuracy and maintain high-quality annotations.
Book our demo with one of our product specialist
Book a Demo