Underwater Fish Annotation Using Labellerr
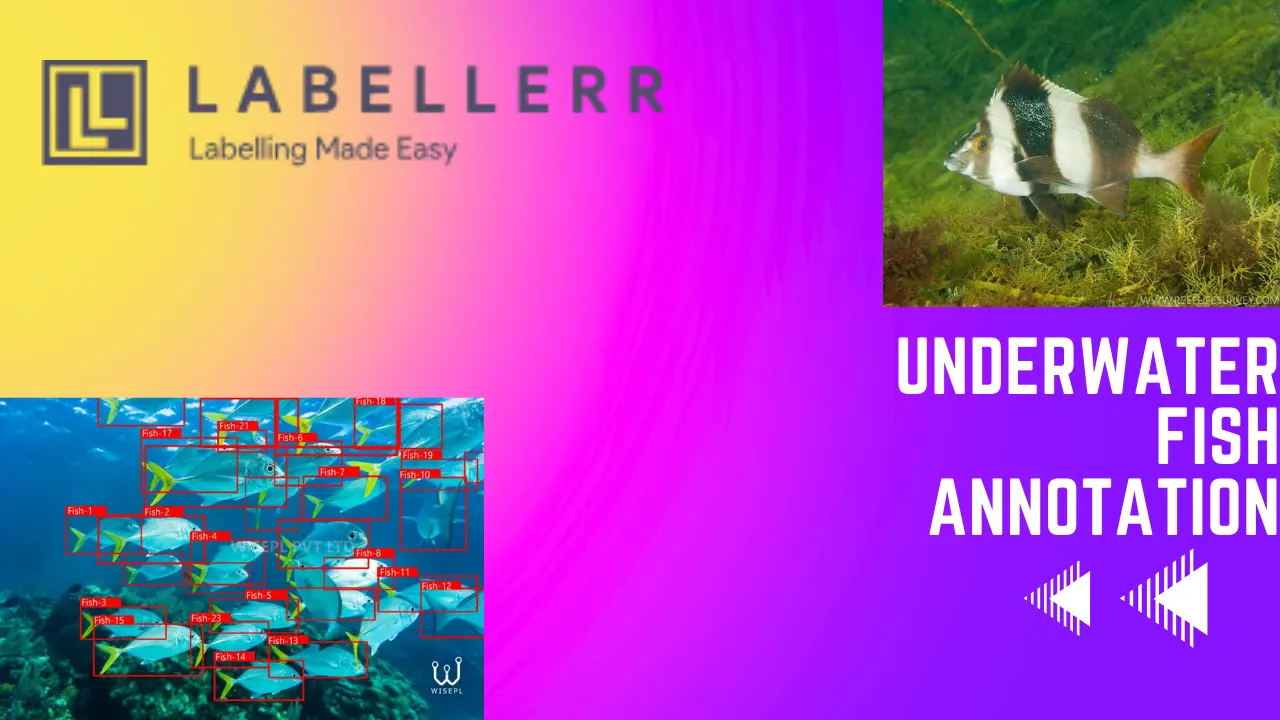
Detecting fish in oceans and seas is a critical task for marine biology, fisheries management, and environmental monitoring.
The size and diversity of the aquatic world makes it challenging to estimate the population a number of fish species. Scientists believe there are approximately 32,000 recognized fish species, with estimates indicating that thousands more have yet to be discovered in the ocean's unknown depths.
Computer vision has become a vital tool in this domain, allowing for automated identification and counting of fish species within large volumes of underwater imagery.
This technology can help researchers monitor fish populations, track migration patterns, and assess the health of marine ecosystems.
However, to train reliable computer vision models for fish detection - accurate and extensive data annotation is essential.
This is where Labellerr, a leading data annotation platform, plays a crucial role. By providing advanced annotation tools, intuitive workflows, and collaborative features, Labellerr facilitates the efficient and accurate annotation of underwater images and videos.
This enables researchers and marine biologists to create robust datasets for training computer vision models, ultimately contributing to more effective monitoring and management of marine environments.
Table of Contents
Challenge
Harsh surroundings: There are specific difficulties for precisely annotating in the underwater domain.
Diverse Lighting: According to the depth and water quality, light is absorbsed and dispersed in the water, resulting in noticeable differences in color and brightness. Fish may become harder to spot in the background or with important characteristics (like markings or fins) that are needed for classification.
Water Clarity: Plankton blooms and suspended particles, can significantly reduce clarity in water. Simply using visual cues to distinguish between species may be difficult for annotators who have trouble seeing fish well.
Dynamic Backgrounds: Fish may be obscured or difficult to track for accurate bounding box annotation in videos by migrating sediments, air bubbles, or swaying kelp.
Annotators may develop specialized pre-processing procedures for underwater photos and videos. These processes may include the following:
Color correction and normalization by adjusting color balance and brightness to adjust absorption and dispersion of light underwater. Also, they can use noise reduction methods for removing artifacts produced by plankton blooms or suspended particles.
Fish Behavior: Underwater fish are always moving, in contrast to still images.
Quick Movement: Fish, especially smaller species, can move quickly, making it difficult to get good video frames and appropriately categorize them.
Overlapping Fish: Fish that are in shoal or hiding in cracks may group together, making it difficult to distinguish and classify each fish in every video frame.
Annotators can use multiple object tracking techniques detect and identify individual fish in schools or overlapping groupings.
Partial Occlusion: Fish may have parts of their bodies obscured by coral, rocks, or other underwater structures, making it challenging to identify them by their entire body shape or other essential characteristics.
Annotators may use instance segmentation by identifying and segmenting individual fish even when some of their body components are occluded, allowing for categorization.
Technological Restrictions: The annotation process for videos may encounter obstacles due to current technological advancements.
Image Quality: The inability to see fine details or distorted video frames due to underwater camera restrictions makes it more difficult to identify fish and their characteristics.
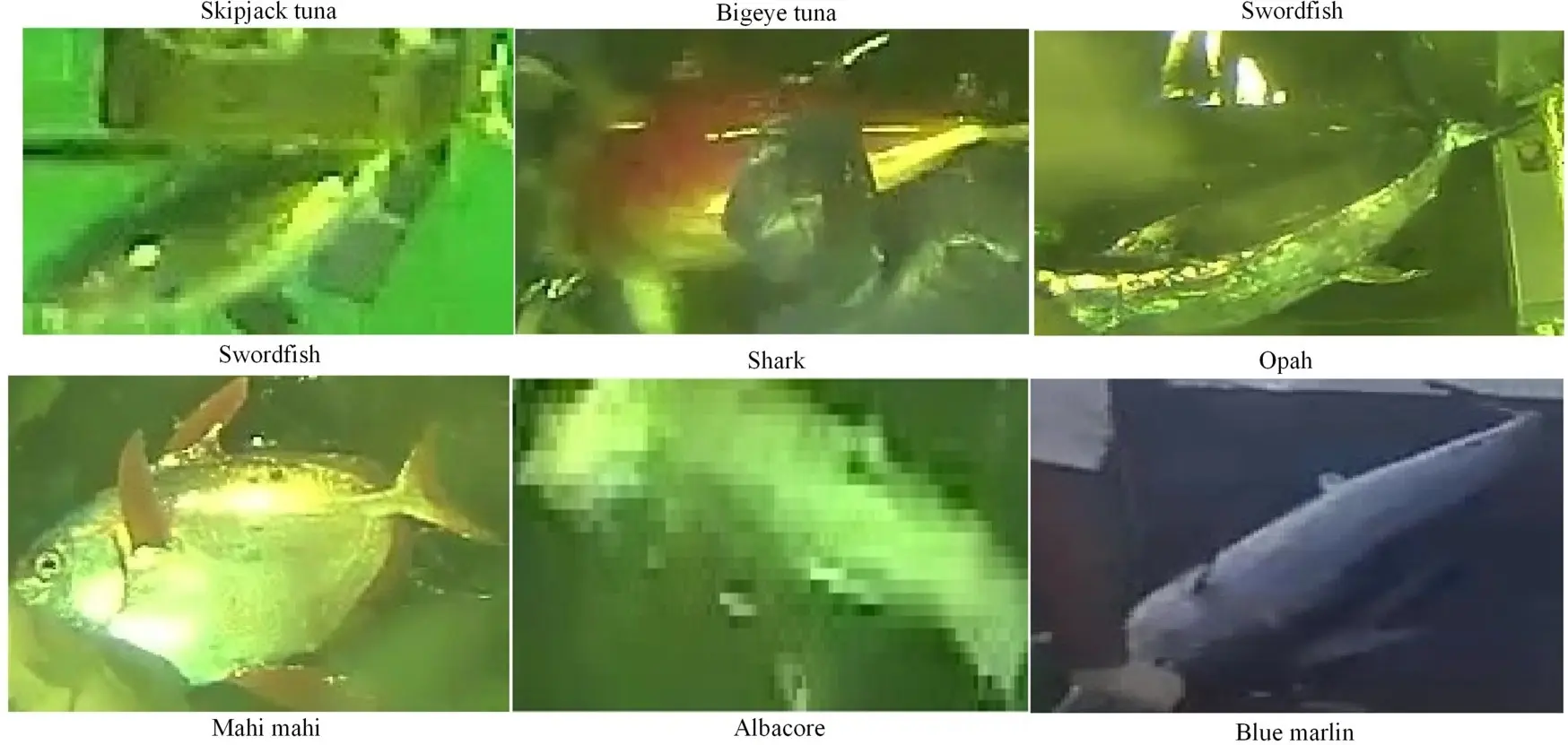
Annotation Tools: The difficulties of labeling moving things in underwater movies may be beyond the capabilities of conventional annotation tools created for static photos.
Annotators may use active learning by selectively annotating video frames that contain the most information for model training, lowering overall annotation time.
Length of Video: Annotating lengthy films can take a lot of time, particularly when trying to monitor certain fish for the duration of the recording.
Solution for Fish Annotation in Oceans with Labellerr
Intuitive Interface
Labellerr provides an intuitive and user-friendly interface designed to simplify the annotation process for fish detection in underwater imagery.
With a clear layout and easy-to-use tools, annotators can efficiently label fish, even in complex scenes.
This intuitive interface minimizes the learning curve, enabling users to quickly adapt to the platform and begin accurate annotation work.
It also reduces errors and increases productivity, making the process more seamless and efficient.
Cost Savings
Labellerr's cost-effective approach to data annotation helps reduce expenses associated with traditional manual annotation methods.
By streamlining workflows and automating repetitive tasks, Labellerr significantly reduces the time and resources needed to create high-quality annotated datasets.
This cost savings is particularly important in large-scale projects, where the annotation of extensive underwater videos and images can become costly if not managed efficiently.
Robust Segmentation Features
Labellerr's advanced segmentation capabilities, including state-of-the-art features like the Segment Anything Model (SAM), are ideally suited for annotating underwater imagery.
These robust segmentation tools allow annotators to precisely identify fish, even when they overlap or blend into the background.
Labellerr's powerful algorithms can handle the complex patterns and rapid movements characteristic of fish in the ocean, ensuring accurate segmentation and tracking across frames.
Custom Workflows
Labellerr offers customizable workflows, enabling teams to tailor the annotation process to their specific needs.
This flexibility is crucial for underwater annotation projects, where conditions and requirements can vary widely.
Custom workflows help streamline the annotation process, allowing teams to focus on key tasks and ensure consistent results.
Additionally, Labellerr's workflows support collaboration among multiple annotators, facilitating distributed work and promoting consistency across the dataset.
Active Learning Based Labeling
Labellerr incorporates active learning into its annotation process, making it ideal for fish detection in underwater videos.
This approach focuses annotators' efforts on the most uncertain or ambiguous parts of the data, allowing them to refine the model's accuracy with minimal manual input.
For fish detection, this is invaluable as it helps identify and label the most complex cases, where fish are overlapping, blending in with the environment, or moving rapidly.
Active learning speeds up the annotation process while enhancing the quality and accuracy of the final dataset.
Automated Import and Export of Data
Labellerr's automated import and export functionalities are designed to seamlessly integrate with various data sources and formats.
This capability is crucial for underwater projects where imagery and videos can come from diverse sources like underwater drones, submersibles, or remote cameras.
With automated import, annotators can quickly bring large datasets into the platform, while automated export ensures that the annotated data is easily transferred to other systems for analysis or further processing.
This automation streamlines workflow reduces manual effort, and ensures data compatibility.
Collaborative Annotation Pipeline
Labellerr's collaborative annotation pipeline allows multiple users to work on the same project simultaneously.
This feature is particularly useful for large-scale underwater projects, where multiple annotators may be required to handle extensive volumes of data.
The collaborative pipeline facilitates real-time communication and coordination among annotators, ensuring consistent labeling and reducing the chances of errors.
It also promotes teamwork and knowledge sharing, leading to more accurate and efficient annotation outcomes in complex fish detection tasks.
Conclusion
In conclusion, Labellerr's comprehensive platform offers a robust solution for fish detection in oceans and seas, effectively addressing the unique challenges associated with underwater annotation.
With powerful tools and features, Labellerr significantly improves the speed, accuracy, and reliability of data annotation for fish detection.
This leads to better training datasets for computer vision models, ultimately contributing to more effective monitoring, research, and conservation efforts in marine environments.
By leveraging Labellerr, researchers, marine biologists, and environmentalists can achieve greater insights into fish populations and their behaviors, advancing our understanding of ocean ecosystems.
Frequently Asked Questions
Q1)What is Labellerr, and how does it help with fish detection in oceans and seas?
Labellerr is a data annotation platform designed to streamline the process of annotating images and videos for computer vision models.
In the context of fish detection in oceans and seas, Labellerr helps annotate large datasets, allowing researchers to identify and track fish more accurately.
It offers robust segmentation tools, custom workflows, and collaborative features, which are crucial for this complex task.
Q2) Why is annotating underwater videos challenging?
Annotating underwater videos is challenging due to factors like changing lighting conditions, water clarity, dynamic backgrounds, and rapid movement of fish.
Fish can also overlap, blend into their environment, or move unpredictably, making it difficult to achieve consistent annotation.
These challenges require advanced tools and efficient workflows to ensure accurate labeling.
References
- Application of Deep Learning-Based Object Detection Techniques in Fish Aquaculture: A Review(Link)
- Research progress of computer vision technology in abnormal fish detection(Link)
- Fish-TViT: A novel fish species classification method in multi water areas based on transfer learning and vision transformer(Link)
Book our demo with one of our product specialist
Book a Demo