Accelerate Tumor & Lesion Annotation With Labellerr
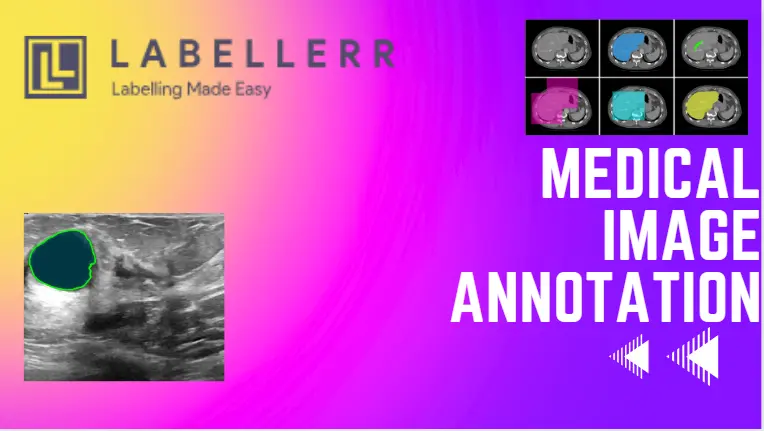
Table of Contents
- Introduction
- Challenge
- Solution: Auto Labeling in Tumor and Lesion Detection
- Conclusion
- Frequently Asked Questions
Introduction
Identifying tumors and lesions is a critical task in medical diagnosis and treatment planning.
The advancements in machine learning and medical imaging technology, automated systems can assist healthcare professionals in accurately detecting and diagnosing tumors and lesions from medical images such as MRI scans, and CT scans.
However, training machine learning models for tumor and lesion identification requires large datasets of annotated medical images.
Labellerr, an annotation platform, plays a pivotal role in annotating medical imaging datasets to create labeled datasets for training accurate tumor and lesion identification models.
Challenge
Identifying tumors and lesions presents a challenge in medical imaging data.
The variability in tumor and lesion characteristics, including size, shape, texture, and location within the body, presents a challenge.
Moreover, the high dimensionality of medical imaging data, such as MRI and CT scans, increases the complexity of annotation tasks as it requires lots of time and labor resources, especially when annotating large volumes of images.
Additionally, class imbalance within medical imaging datasets, where tumor and lesion cases are outnumbered by normal cases, poses a significant problem, necessitating the creation of balanced datasets for effective model training.
Furthermore, privacy and ethical considerations surrounding patient data confidentiality mandate strict adherence to regulatory standards.
Overcoming these challenges requires advanced annotation methodologies and tools to ensure accuracy, consistency, and compliance while generating high-quality labeled datasets essential for training precise tumor and lesion identification models.
Solution: Auto Labeling in Tumor and Lesion Detection
Intuitive Interface
Labellerr provides an intuitive user interface designed to simplify the annotation process for annotators working on tumor and lesion identification tasks.
Its user-friendly design and navigation tools empower annotators to label abnormalities within medical images with ease, reducing the learning curve and enhancing productivity.
Now annotators can focus on annotation tasks without being hindered by the complexity of medical imaging data.
Robust Segmentation Features
Labellerr incorporates robust segmentation features, including the Segment Anything Model (SAM), which enables precise and accurate identification of tumors and lesions within medical images.
SAM's advanced algorithms can effectively segment abnormalities, regardless of their size, shape, or location within the body.
SAM's segmentation capabilities ensures the accuracy and reliability of annotated datasets, enhancing the performance of tumor and lesion identification models.
Cost Savings
Labellerr offers substantial cost savings for tumor and lesion identification tasks by optimizing the annotation process and reducing manual annotation costs.
Its advanced automation features streamline the annotation workflow, minimizing the need for extensive human intervention.
Automating repetitive tasks and leveraging efficient annotation algorithms enables healthcare professionals to annotate large volumes of medical imaging data efficiently and cost-effectively, ultimately saving time and resources.
Custom Workflows
Labellerr supports customizable workflows tailored to the specific requirements of tumor and lesion identification tasks.
Healthcare professionals can define custom annotation protocols, designate abnormality categories, and customize labeling criteria to suit their unique needs.
This flexibility ensures that annotations are consistent, standardized, and aligned with the objectives of the project, ultimately leading to more reliable and accurate labeled datasets for training tumor and lesion identification models.
Active Learning Based Labeling
Labellerr employs active learning techniques to optimize the tumor and lesion identification process.
Intelligently selecting the most informative samples for annotation, Labellerr maximizes the efficiency of data labeling, reducing manual effort while improving the performance of trained models.
This active learning-based approach enables healthcare professionals to prioritize labeling efforts on data points that are most beneficial for model training, ultimately leading to more accurate and effective tumor and lesion identification models.
Automated Import and Export of Data
Labellerr streamlines the process of importing and exporting data for tumor and lesion identification tasks with its automated functionalities.
Labellerr allows various export of data including YOLO (You Only Look Once) models for object detection, healthcare professionals can seamlessly upload medical imaging data into the platform.
This integration with YOLO models enables efficient object detection and annotation, ensuring accurate labeling of tumors and lesions within medical images.
Collaborative Annotation Pipeline
Labellerr facilitates collaboration among healthcare professionals with its collaborative annotation pipeline.
Multiple users can work simultaneously on annotating medical images for tumor and lesion identification, allowing for distributed workflows and real-time collaboration.
This collaborative approach enhances productivity and ensures consistency and accuracy in the labeled dataset, ultimately improving the performance of tumor and lesion identification models.
Automated QA (Quality Assurance):
Labellerr integrates automated quality assurance mechanisms to ensure the accuracy and reliability of annotated tumor and lesion identification data.
Advanced algorithms analyze annotations in real-time, flagging inconsistencies or errors for review by healthcare professionals.
This automated QA process maintains the quality of the labeled dataset, reducing the risk of errors and ensuring the high performance of trained models in tumor and lesion identification tasks.
Conclusion
In conclusion, Labellerr emerges as an indispensable tool in the realm of tumor and lesion identification, offering a comprehensive solution that addresses the complex challenges inherent in medical imaging annotation.
By leveraging advanced features such as active learning-based labeling, integration with YOLO models for object detection, collaborative annotation pipelines, and automated quality assurance.
Labellerr allows healthcare professionals to annotate medical imaging data efficiently and accurately.
As a result, Labellerr helps in the development of precise and reliable tumor and lesion identification models, ultimately contributing to improved diagnostic accuracy, treatment planning, and patient care in the field of healthcare.
Frequently Asked Questions
Q1) What is tumor and lesion identification, and why is it important in healthcare?
Tumor and lesion identification involve the detection and characterization of abnormal growths or lesions within the body using medical imaging techniques such as MRI, CT, and ultrasound.
It is crucial for early diagnosis, treatment planning, and monitoring of various medical conditions, including cancer.
Q2) How does Labellerr assist in annotating medical imaging data for tumor and lesion identification?
Labellerr provides advanced annotation tools and features specifically designed for annotating medical imaging data for tumor and lesion identification tasks.
Its intuitive interface, integration with object detection models such as YOLO, and automation capabilities streamline the annotation process, enabling healthcare professionals to annotate large volumes of data efficiently and accurately.
Book our demo with one of our product specialist
Book a Demo