How Foresight Health Accelerates Its Medical Transcript Tagging With Labellerr
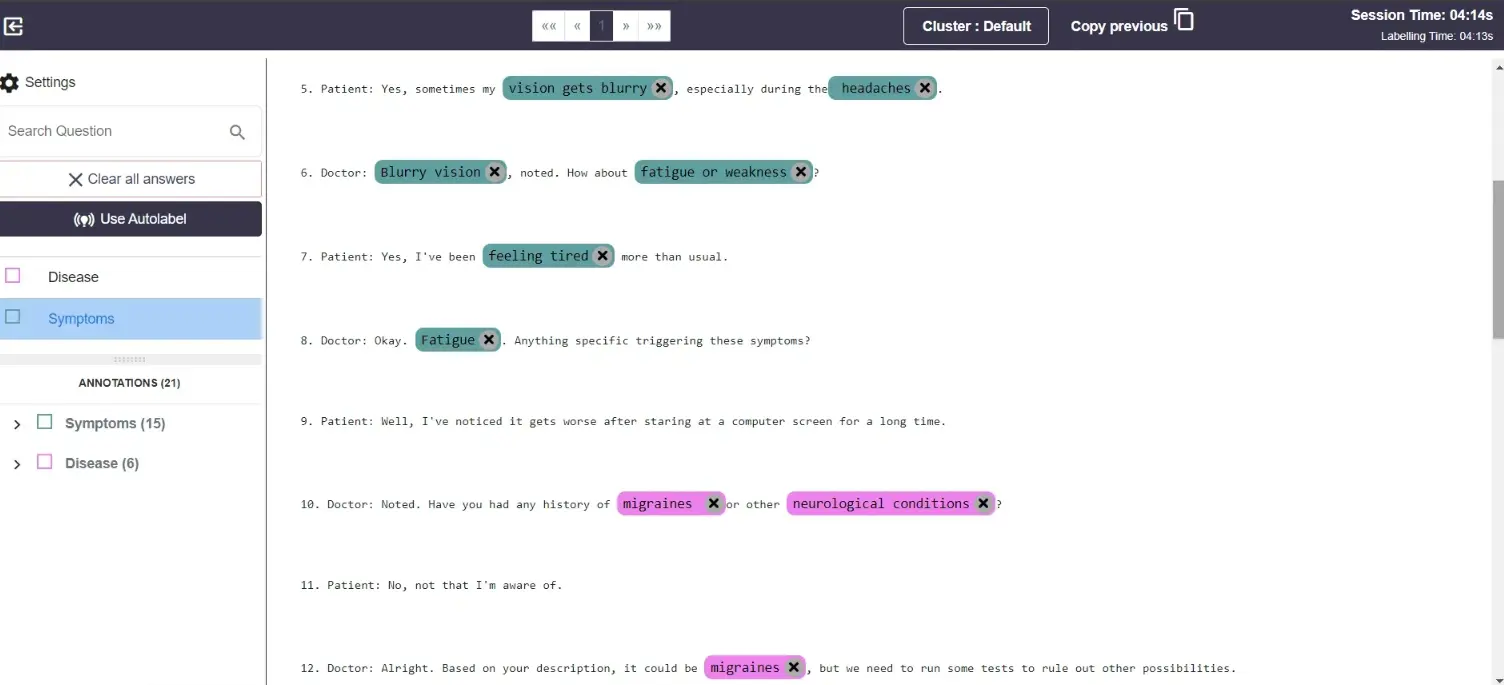
Introduction
In the fast-changing world of healthcare data, Foresight Health Solutions faced a tough challenge which was the need to analyze and mark more than 10,000 patient reports accurately in just one month, aiming for a super-high accuracy of 99%.
Imagine going through piles of important documents in such a short time which is like finding a needle in a haystack!
That's when Labellerr stepped in and helped like a guiding light.
Labellerr brought in a fresh way of working which made the process of handling healthcare data more organized and precise to deal with important medical information.
Let's dive and explore in this blog how this collaboration transformed the way Foresight Health Solutions handled its critical task.
Our Customer
Foresight Health Solutions is a healthcare analytics platform, that stands as a vanguard in leveraging data for enhanced patient care and outcomes.
Their dedication to cutting-edge solutions and data-driven insights positions them at the forefront of innovation in the healthcare industry.
Foresight Health Solutions uses smart technology to make sure patients get the best care possible.
They're leaders in using data (which is like smart information) to improve how doctors and nurses help people feel better.
Foresight Health Solutions is always looking for new and smart ways to make a difference in the healthcare world.
Data Volume and Accuracy Challenge
Foresight Health Solutions had a massive collection of patient reports with tons of information.
They had this tricky job of marking more than 20 different things in each report, and each of these things had many details.
It's like trying to highlight specific words in a giant book.
Here's the catch: they had to do all this super accurately, aiming for 99% accuracy, and they had only one day!
This made the task not just tough but like a big challenge for their tech skills.
Let's see how they tackled this tricky situation with their technological know-how.
How Labellerr Stepped In
1. Easy Data Import and Connectivity
Labellerr's seamless integration with cloud platforms became the most important thing of Foresight Health Solutions data handling strategy.
The platform's ability to effortlessly import vast amounts of patient data at regular intervals ensured a continuous and uninterrupted flow, addressing the volume challenge with unparalleled efficiency.
2. Intuitive UI for Faster Annotation
Labellerr's user-friendly interface emerged as a force multiplier in expediting the annotation process. The platform's intuitive tools for entity tagging not only accelerated the annotation workflow but also upheld the highest standards of accuracy, aligning seamlessly with Foresight Health Solutions stringent requirements.
3. Efficient Workflow Management
Managing the annotation of multiple entities and attributes necessitated a meticulously organized workflow.
Labellerr's robust workflow management capabilities empowered Foresight Health Solutions to seamlessly onboard multiple domain experts for annotation, ensuring a thorough verification process and contributing significantly to achieving the ambitious 99% accuracy goal.
4. Complete Automation for Minimal Intervention
Labellerr's automation prowess drastically reduced the manual intervention required in the annotation process.
What once demanded hours of weekly effort from Foresight Health Solutions team was streamlined to mere minutes, allowing them to focus their expertise on refining their NER model.
5. Seamless Collaboration for Guideline Clarity
Effective collaboration became the hallmark of success in Foresight Health Solutions partnership with Labellerr.
The platform facilitated swift updates to annotation guidelines, serving as a central communication hub.
This ensured a collective understanding of guidelines among all stakeholders, fostering consistency and elevating the overall accuracy of the annotation process.
Conclusion
Foresight Health Solutions experience stands as a testament to the transformative power of advanced annotation tools in the healthcare sector.
Labellerr's pivotal role in optimizing the annotation process showcases the potential of technology in addressing complex challenges, contributing not only to advancements in patient care but also in reshaping the landscape of healthcare analytics.
In terms of precision and innovation, Labellerr is best, for providing solutions that propel healthcare analytics initiatives toward success.
Frequently Asked Questions
1. Are medical NER methods based on pre-trained language models?
Yes, many medical Named Entity Recognition (NER) methods leverage pre-trained language models as a foundational component of their approach.
Pre-trained language models are general-purpose models trained on vast amounts of diverse text data to learn the patterns and structures of language.
These models, like BERT (Bidirectional Encoder Representations from Transformers) and others, capture semantic relationships and contextual information in a language-agnostic manner.
When applied to the medical domain, researchers and practitioners often fine-tune these pre-trained models on specialized medical datasets to adapt them to the nuances and specific language structures of medical texts.
The fine-tuning process allows the model to learn domain-specific terminology, relationships, and context that are crucial for accurate Named Entity Recognition in healthcare.
The advantage of using pre-trained language models lies in their ability to capture intricate linguistic features and context, even when dealing with complex medical language.
This approach tends to outperform traditional rule-based or shallow machine learning methods, as pre-trained models can generalize well across various tasks and domains.
2. What is medical named entity recognition?
Medical Named Entity Recognition (NER) is a specialized natural language processing (NLP) task focused on identifying and classifying specific entities within medical texts.
The primary goal of NER in the medical domain is to automatically extract and categorize relevant information, such as diseases, symptoms, medications, procedures, and other medical entities, from unstructured textual data like clinical notes, research articles, or electronic health records (EHRs).
This process is crucial for unlocking valuable insights from the vast amount of medical information available, enabling more efficient data analysis, information retrieval, and ultimately improving patient care.
In practice, medical NER involves developing machine learning models or utilizing pre-trained language models that can recognize and classify entities based on learned patterns and context within the medical text.
These models are trained on labeled datasets that contain examples of various medical entities, allowing them to generalize and accurately identify similar entities in unseen texts.
The accuracy and efficiency of medical NER have significant implications for tasks such as clinical decision support, information extraction from medical literature, and enhancing the overall efficiency of healthcare information systems.
Book our demo with one of our product specialist
Book a Demo