7 Best Image Segmentation Tools
Explore the 7 best image segmentation tools in 2024, offering powerful features like semantic segmentation, object detection, and AI-assisted annotation. Platforms like Labellerr, Labelbox, and CVAT simplify the process with intuitive interfaces, enhancing computer vision projects.
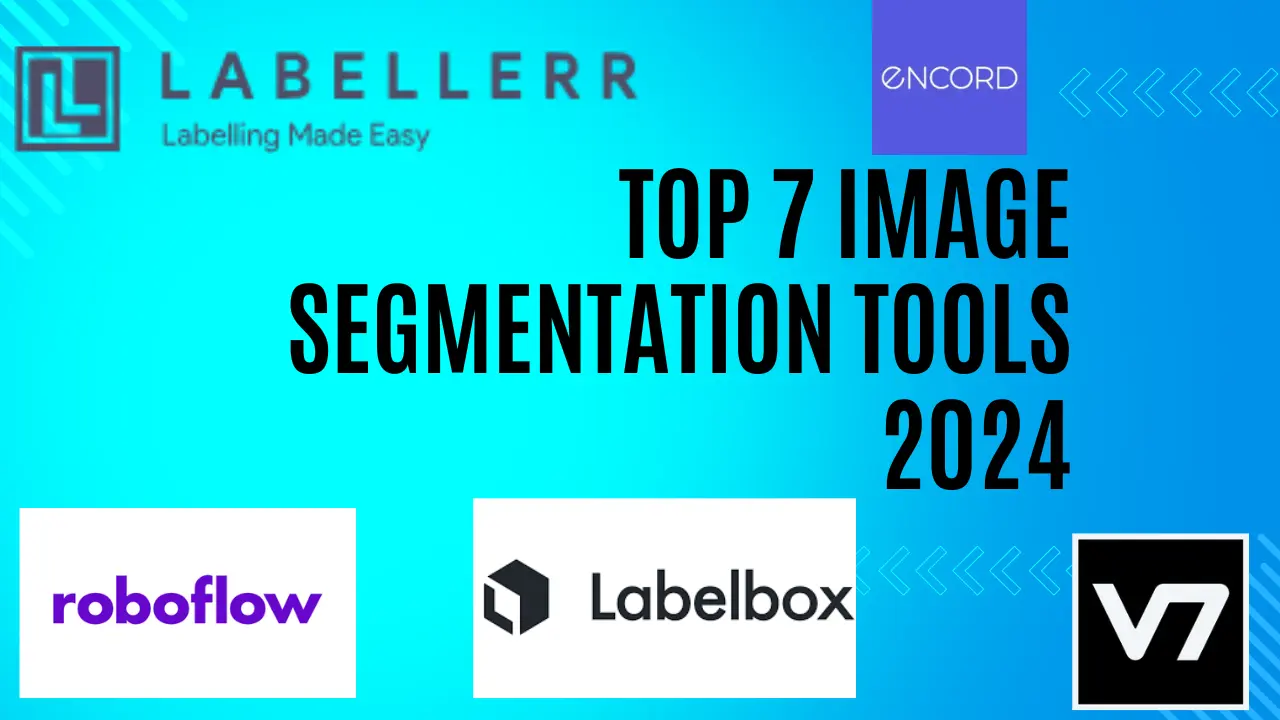
Are you struggling with the complexities of image segmentation in your computer vision projects?
You're not alone.
Many professionals face the challenge of efficiently dividing images into meaningful segments, whether for object detection, semantic segmentation, or AI-assisted annotation.
The process can be time-consuming, intricate, and difficult to master, especially without the right tools.
But here's the good news: in 2024, advanced image segmentation platforms are making this process easier and more accessible than ever.
These tools are revolutionizing the way we approach image segmentation with intuitive interfaces and powerful features that streamline workflows.
In this post, we'll explore the top 7 image segmentation tools that are shaping the future of computer vision. These platforms are designed to solve the very problems you're facing by simplifying complex tasks, enhancing productivity, and ensuring high-quality results.
Table of Contents
- Features to Look in an Image Segmentation Tools
- Labellerr
- Labelbox
- V7 Labs
- Encord
- CVAT
- Dataturks
- Roboflow
- Conclusion
- Frequently Asked Questions
Features to Look in an Image Segmentation Tools
There are several important factors to consider when comparing image segmentation tools. The following are some crucial qualities to consider:
1. Accuracy and Quality
Ensure the tool has a solid track record for producing accurate and high-quality annotations. Check their history, client testimonials, and any credentials they might have.
2. Annotation Capabilities
Check to see if the supplier delivers the particular annotation you need. Examples include instance segmentation, bounding box annotation, semantic segmentation, object identification, picture classification, and keypoint annotation.
3. Scalability
Evaluate the supplier's capacity for managing significant annotation projects. To be sure they can handle your volume and deadlines, consider their infrastructure, labor capacity, and project management skills.
4. Annotation Tools and Technology
Consider the provider's utilization of these resources. Search for cutting-edge algorithms, machine learning methods, and automation features that can improve annotation precision and speed.
5. Data Security and Confidentiality
Verify that the supplier has effective security measures to safeguard your data and uphold confidentiality. Data Security and Confidentiality. Secure data transmission methods, encryption, access restrictions, and adherence to pertinent data protection laws are all included in this.
6. Customization and Flexibility
Assess the provider's flexibility and ability to customize their annotation services to your unique needs. Custom annotation rules, annotation formats, and other unique requirements you might have ought to be supported by them.
7. Turnaround Time
Consider the provider's capacity to supply annotated data within the periods you want. Keep an eye out for things like their typical turnaround time, capacity for handling rush orders, and communication responsiveness.
8. Pricing and cost-effectiveness
Evaluate the pricing plans of various suppliers to make sure they fit within your spending limit. Consider the trade-off between price and quality, and seek vendors that can deliver accurate information at a reasonable price.
9. Domain Knowledge
Look for suppliers with experience and knowledge in your particular business or domain, such as autonomous vehicles or medical imaging, if your annotation needs are specialized in that area. They'll be more knowledgeable about your particular needs.
10. Customer Support and Communication
Consider how well the supplier supported and communicated with customers during the annotating process. Make sure they are accessible, have a specific point of contact, and are eager to respond to any queries or problems you may have.
Considering these aspects, you can choose an image segmentation tool provider that best suits your unique demands and objectives.
7 Top Image Segmentation Tools in 2024
1. Labellerr
Labellerr is a powerful platform designed to streamline the image segmentation process. Using AI-assisted tools, it enables users to quickly label images with segmentation masks, offering both manual and automatic annotation options.
It is ideal for projects that need to label a wide variety of images, from objects to complex environments, and integrates smoothly into machine learning pipelines for scalable workflows.
Top Features:
- AI-assisted segmentation tools.
- Customizable project workflows for image annotation.
- Real-time collaboration features for teams.
- Integration with ML pipelines for automatic model training.
- Support for multiple data formats and annotation types.
Pros:
- Flexible and scalable for different project sizes.
- High-quality AI tools to speed up annotation.
- Seamless integration into ML pipelines.
Cons:
- Some advanced features may require setup time.
- Focused more on ML pipeline integration, so less suited for smaller-scale, non-ML projects.
Pricing:
- Custom pricing based on project needs and scale.
G2 Review:
Labellerr is highly rated for its ease of use, AI-driven accuracy, and its ability to integrate into existing workflows. Users especially appreciate the speed at which images are segmented and the platform's scalability for larger teams.
Rating: 4.5/5
2. Labelbox
Labelbox is a robust data labeling platform known for its powerful image segmentation tools. It allows users to annotate images with precise polygon, box, and mask annotations, ideal for machine learning models that require high-quality segmentation.
It supports a collaborative environment for teams and offers advanced features like quality control and integration with ML models for continuous improvement.
Top Features:
- Polygon, bounding box, and mask segmentation tools.
- Quality assurance features for annotation accuracy.
- Real-time collaboration and feedback options.
- Integration with popular ML frameworks.
- Customizable workflows for different types of annotation.
Pros:
- Comprehensive features for image segmentation.
- Efficient collaboration tools for large teams.
- Easy integration with ML pipelines.
Cons:
- Pricing can be expensive for smaller teams or startups.
- Requires setup time for custom workflows.
Pricing:
- Tiered pricing based on features and team size.
G2 Review:
Users find Labelbox’s segmentation tools accurate and the platform intuitive to use. However, some express concerns over pricing, particularly for small-scale projects. The integration with ML models is a highlight.
Rating: 4.6/5
3. V7 Labs
V7 Labs is a powerful data annotation platform with a strong focus on image segmentation. It leverages computer vision to automate some aspects of annotation, reducing the manual effort required.
It supports a wide range of annotation tasks, including pixel-perfect segmentation, and allows for rapid iterations, making it an excellent tool for large-scale machine learning projects.
Top Features:
- AI-assisted annotation tools for faster segmentation.
- Multiple annotation types, including segmentation masks.
- Real-time collaboration and quality control features.
- Integration with ML pipelines for model training.
- Customizable project templates for varied workflows.
Pros:
- Automation tools speed up the segmentation process.
- High precision in segmentation tasks.
- Great for large datasets and teams.
Cons:
- Some users find the interface challenging at first.
- Can be expensive for smaller-scale projects.
Pricing:
- Custom pricing depending on usage and features.
G2 Review:
V7 Labs is appreciated for its speed, accuracy, and seamless integration with ML workflows. Users love the AI-powered assistance, though it may take time to master for new users.
Rating: 4.7/5
4. Encord
Encord is a data labeling platform that provides a robust set of tools for image segmentation. It is designed for teams that need to label large datasets quickly and efficiently, with a strong focus on deep learning applications.
It’s platform allows for easy collaboration and integrates well with AI models to continuously improve annotation accuracy.
Top Features:
- Image segmentation tools with polygon and mask options.
- Customizable workflows for different annotation needs.
- AI-assisted annotation features for speed and accuracy.
- Integration with ML pipelines for model feedback.
- Collaborative tools for team-based annotation.
Pros:
- Easy to scale for large projects.
- Collaboration-focused for team efficiency.
- AI-powered features enhance annotation speed.
Cons:
- May be overkill for smaller, less complex projects.
- Pricing can be a concern for smaller teams.
Pricing:
- Custom pricing based on team size and requirements.
G2 Review:
Encord receives positive reviews for its ease of use, scalability, and AI-enhanced annotation capabilities. Some users note that it’s better suited for larger teams and complex projects, but it does not cater well to smaller scale users.
Rating: 4.6/5
5. CVAT
CVAT (Computer Vision Annotation Tool) is an open-source tool that provides a wide range of image segmentation features. It allows users to annotate images with pixel-perfect accuracy and includes support for polygon and mask-based annotations.
It is favored by many for its flexibility and is particularly popular in research and development environments.
Top Features:
- Support for polygon and mask segmentation.
- Real-time collaborative annotation for teams.
- Open-source, allowing full customization.
- Integrates with machine learning models for continuous feedback.
- Highly flexible for various annotation tasks.
Pros:
- Free and open-source, great for research and development.
- Highly customizable for different needs.
- Active community support.
Cons:
- Interface can be complex for beginners.
- Lacks the polished user experience of paid tools.
Pricing:
- Free (open-source).
G2 Review:
CVAT is praised for being a powerful and customizable tool, but users note its complexity for those new to image segmentation. It’s ideal for teams comfortable with open-source tools and looking for flexibility.
Rating: 4.3/5
6. Roboflow
Roboflow is a comprehensive data labeling platform that specializes in image segmentation tasks. It offers an intuitive interface and multiple annotation types, including masks and polygons.
It also provides advanced features like pre-trained models and automatic annotation suggestions to help speed up the labeling process.
Top Features:
- Polygon and mask-based segmentation.
- AI-assisted annotations and pre-trained models.
- Real-time collaboration features for teams.
- Easy integration with ML pipelines.
- Extensive dataset management and analytics tools.
Pros:
- AI-assisted tools accelerate the annotation process.
- Supports a variety of data formats.
- Integrates well with machine learning frameworks.
Cons:
- Pricing can be expensive for large-scale teams.
- Limited customization compared to open-source platforms.
Pricing:
- Free tier available; paid plans for larger teams and features.
G2 Review:
Roboflow receives strong praise for its intuitive interface and the time-saving AI tools. It’s particularly loved for its ability to streamline workflows, though some users find it costly for large-scale projects.
Rating: 4.6/5
7. Dataturks
Dataturks is a versatile data annotation platform that offers easy-to-use tools for image segmentation, among other tasks like text annotation and audio labeling.
It provides powerful features for both manual and AI-assisted annotation of images, making it suitable for various machine learning and computer vision projects.
It is ideal for teams looking to annotate large datasets efficiently, with a focus on collaboration and ease of use.
Top Features:
- Supports mask and polygon segmentation for images.
- AI-assisted tools for faster annotation and labeling.
- Real-time collaboration and feedback tools for teams.
- Customizable workflows for different types of data.
- API integration with machine learning models for data processing.
Pros:
- Intuitive interface that is easy to navigate.
- AI-assisted features help speed up the annotation process.
- Suitable for both small and large-scale projects.
- Affordable pricing for startups and small teams.
Cons:
- Lacks some advanced features available in more specialized tools.
- Limited support for more complex segmentation tasks.
Pricing:
- Free tier available with limited features.
- Paid plans based on the scale and number of annotations required.
G2 Review:
Dataturks is highly regarded for its user-friendly interface and efficient team collaboration features. Users appreciate the ability to quickly label images using both manual and AI-assisted tools. However, some note that the platform could benefit from more advanced segmentation features and customization options for larger teams.
Rating: 4.4/5
Conclusion
The evolution of image segmentation tools has significantly transformed the area of computer vision, allowing users to create high-quality datasets with greater ease and accuracy.
Platforms like Labellerr, Encord, and Roboflow offer robust and intuitive solutions for various segmentation needs, from semantic and instance segmentation to more complex polygonal techniques.
These tools cater to a wide range of users, from individual developers to large teams, and support collaborative workflows that enhance productivity and consistency.
The integration of AI-assisted annotation, compatibility with popular machine learning frameworks, and comprehensive data management features make these platforms adaptable to different use cases.
Whether you're working on medical imaging, autonomous vehicles, or robotics, these tools provide the functionality and flexibility required to streamline your projects.
As image segmentation continues to play a crucial role in advancing computer vision and AI, these platforms are driving innovation and enabling a new era of automation and intelligent applications.
The choice will depend on your specific requirements, team dynamics, and desired level of automation. Still, each offers a robust set of features to help you achieve your segmentation goals.
Read our other popular listicle:
1. Top 10 Best Video Annotation & Labeling Tools In 2024
2.Top 10 Best Image Labeling & Annotation Tools in 2024
Frequently Asked Questions
Q1) What is image segmentation, and why is it important in computer vision?
Image segmentation is the process of dividing an image into distinct regions or segments to isolate objects, boundaries, or areas of interest.
It's crucial in computer vision because it allows for more detailed analysis, enabling tasks like object detection, medical imaging, facial recognition, and autonomous driving to be performed with greater accuracy.
Q2) What are some common types of image segmentation?
The common types of image segmentation include semantic segmentation, where each pixel is assigned a class label; instance segmentation, where each object instance is uniquely identified; and polygonal segmentation, which involves creating custom shapes around objects.
These techniques have diverse applications depending on the problem at hand.

Simplify Your Data Annotation Workflow With Proven Strategies
Download the Free Guide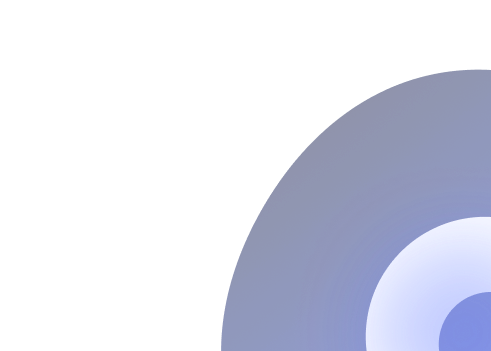