These obstacles to automated data labeling can harm you! Remove them now!!!
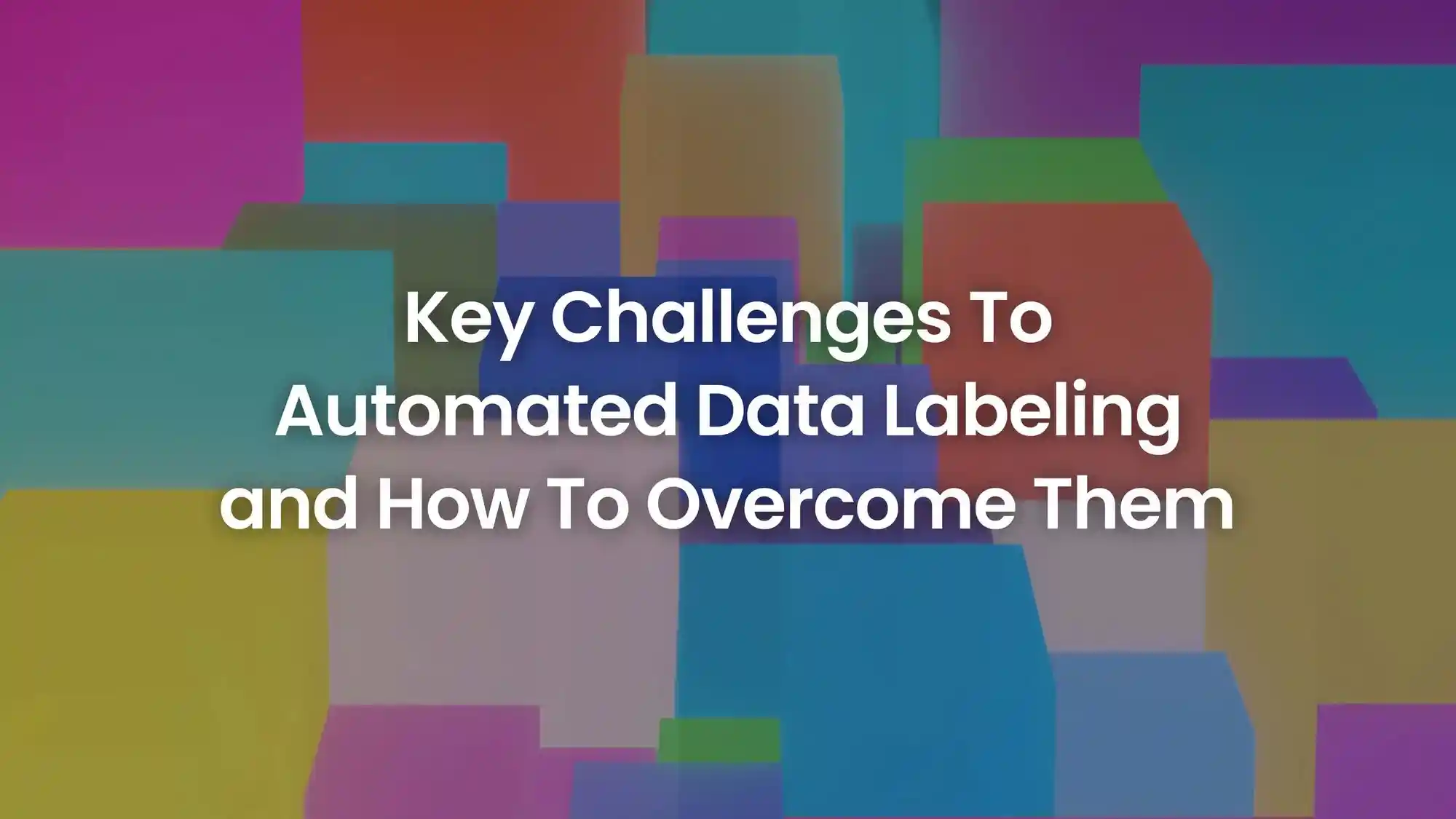
Data labeling is not a simple operation; it takes a great deal of expertise, knowledge, and work to identify the data used for machine learning training.
Furthermore, in order to prepare the computer vision algorithms that help the visual perception model detect different things, annotated photographs are required.
However, businesses run into a number of issues when identifying the various data kinds, which makes the process time-consuming and ineffective. We need to comprehend these issues in order to improve the productivity and effectiveness of data labeling.
So, we'll talk about data labeling difficulties in this blog article and offer some solutions.
Table of Contents
- Erroneous Labeling
- Practice Period
- The Likelihood of Errors
- QA Flaws
- Choosing the Appropriate Tools & Methods
1. Erroneous labeling
The guidelines or guidance provided by a sample data set determine how effectively automation is used. The automated program is aware that the sample given by the engaged data scientists or researchers should be the basis for its labeling decisions.
If the test datasets are fallible, this will inevitably result in situations in which the automated processes are incorrect and need to be rectified to prevent future incidents or the possibility of inadequately annotated data that may be inappropriate for training without even being repurposed and under the manual supervision of human labelers.
Because it is ultimately unproductive and counterproductive, this is a severe difficulty for those using automated features and functionality when annotating data. Auto labeling must provide datasets that are at least as high-quality as those that are handled manually for it to be effective and to achieve its goal.
Solution
In automated labeling, you can reduce a large number of chances of erroneous labeling by including human-in-the-loop. Experts can really help you achieve the goal of getting supervised models.
Although, ML teams should be careful when producing sample datasets that are specifically designed for training auto-labeling systems to ensure that the algorithms accurately read and carry out labeling instructions—starting with the creation of a perfect ground truth data set, ideally by a short manual auditing procedure that can spot label errors and get them ready for training.
2. Practice period
Automated labeling typically ends up being a far more effective way to prepare datasets over time, although the models that are used to achieve this still need to be trained.
The main issue with this difficulty is rather simple: how much time is spent on training, whether it is worthwhile in each individual situation, and whether it meets project requirements, including deadlines for production.
This issue arises specifically when model-assisted labeling, also known as using existent ML models for labeling, is attempted. To confirm and ensure that the gentle labels they generate are accurate, this approach necessitates extensive human engagement in ongoing observation.
Additionally, that model can only be successful at categorizing things that it has already been appropriately trained on, thus in order to address any new special cases or use cases, you will have to go through the entire lifecycle of ML model training.
This means that no matter how similar the use cases or the target sector are, ML teams can anticipate applying incremental changes depending on inconsistent and erroneous results as they analyze datasets over the term, especially between projects.
Solution
The iterative training requirements of an auto-labeling model can, however, be satisfied with a customized solution. With just a short ground truth data, a few clicks, and no more than an hour or less of preparation time. With Labellerr’s workflow management system, you can achieve this.
Labellerr's unique workflow management system is completely flexible. This enables the annotators to quickly recognize and label items in datasets and is easily adaptable to the precise use cases of each team and project.
The entire workflow process is automated by the smart feedback loop, which increases the overall data pipeline's efficiency and makes it simple to handle without missing a beat. To automate the computer vision data pipeline, it records all the actions, notes, instructions, and pixel-level views of the images and movies.
By streamlining the generation of training data, which is made possible by automating data collection, curation, and annotation, the feedback loop is a system that ensures effective use of the AI/ML team's time.
To save labeling time, Labellerr even introduced the copy the label feature which reduces the time spent on each image by up to 70%.
This allows annotators to quickly and easily copy all the labels from their previous annotations. Our method sorts the annotated image based on similarity with the best likelihood of comparable labels for big and diverse datasets.
Additionally, because it will always be at the top, this saves annotators time from having to search for the appropriate image to copy.
3. The likelihood of errors
Auto labeling program can be tricked, just like a locomotive that has been taken off its tracks and redirected with the flick of a lever. This may have a continuing, long-term impact on how effective it is from the moment or period when the proper labeling criteria were abandoned.
The model's mechanical inclination to follow the outcomes or results it has been programmed to create, rather than to realign itself if those results are no longer accurate, accounts for this.
Whether or not the right data points and annotation approaches have been assigned to and used in a model, it will still go down the path it has been set.
When a model commits a mistake or an error, it is more likely to keep doing so in the future, perhaps indefinitely. Because of this, it's critical for labellers and other AI/ML development members to have the tools necessary to identify and fix persistent errors before they contaminate or corrupt a sizable section of a dataset pool that appears to have been processed and adversely affect the performance and functionality of an ML model once trained.
Solution
The greatest possible solution would be to measure and assess the "trustability" of an auto-label AI's output in order to lower the rate or frequency of inaccuracy. Technology like uncertainty estimates can be useful in this situation.
A data team's ability to place their faith in the results of their models is statistically measured using this technique, to put it simply. They can then use that measurement to compute the proportionate probability of prediction mistakes and the probability of them.
We propose uncertainty estimation for labelers, which assesses the complexity of an automated labeling task. You can check the level of accuracy achieved by your model and even analyze it further.
At the stage of reviewing, you can only focus on the mismatched labeled data probabilities. This will further reduce the chances of overall mistakes and will ensure the timely processing of data.
The probability of model mistakes brought on by problems in the data set can be greatly reduced by concentrating on a few datasets.
Challenge 4: QA flaws
The primary benefits of using automation in data labeling procedures for ML teams are well known: they reduce the amount of time typically required for traditional data labeling techniques and eliminate the requirement to produce large, high-quality datasets in order to create ML models.
Even if it's a big task, auto-labeling can unquestionably complete it and even go above and above.
When labeling leads and/or project managers monitor the procedure from a high-level viewpoint, it is easiest to produce optimal and adequate training data.
The need for human interference and intervention is reduced compared to a fully manual labeling pipeline, including data collection and identification cleaning, accumulation, and of course, the real annotation tasks.
Instead, it is intended to be sparingly used, typically to make targeted adjustments to improve procedures based on inadequacies, auto-label mistakes, and programming misinterpretations.
Solution
Through a comprehensive labeling platform like the Labellerr platform, which includes team management and analytics capabilities, this top-level view can be easily set up and utilized to monitor labeling project data.
These technologies enable ML project managers to identify problem areas in a labeling workflow and make the necessary changes to improve automated labeling processes.
5. Choosing the appropriate tools & methods
For data annotation organizations, having the correct tools and well-trained employees is crucial for producing high-quality training datasets.
However, understanding is required for all automated machines, AI-assisted data labeling, manual data annotation, automation, and data administration.
Actually, multiple tools and approaches are utilized to label the information for deep machine learning depending on the sorts of data.
Software and solutions of various kinds that were created specifically for data labeling are readily accessible on the market. The three most popular picture annotation methods taken into account while labeling the data are bounding box labeling, semantic segmentation, and point cloud annotation.
However, producing such customized tools requires a significant amount of funding for in-house tooling.
Additionally, some businesses take a conservative approach to manual data labeling, which makes it challenging or impossible to meet the standards.
Actually, developing your tool impacts the level of the datasets and raises your costs as well. Therefore, you should think about if the tools you choose offer all the services you're seeking before purchasing them from a third party.
Here, it is crucial to pick a reliable data annotation system that can guarantee quality and is reasonably priced.
Solution
Go for Labellerr. Labellerr offers a smart feedback loop that automates the processes that help data science teams to simplify the manual mechanisms involved in the AI-ML product lifecycle.
We are highly skilled at providing training data for a variety of use cases with various domain authorities. By choosing us, you can reduce the dependency on industry experts as we provide advanced technology that helps to fasten processes with accurate results.
A variety of charts are available on Labellerr's platform for data analysis. The chart displays outliers if any labels are incorrect or to distinguish between advertisements.
We accurately extract the most relevant information possible from advertisements—more than 95% of the time—and present the data in an organized style. having the ability to validate organized data by looking over screens.
If you are looking for a place that can provide you with a unified platform to solve all these automated data labeling problems, then reach out to us at Labellerr.
Book our demo with one of our product specialist
Book a Demo