These 10 datasets you won't find on Kaggle-Part 2
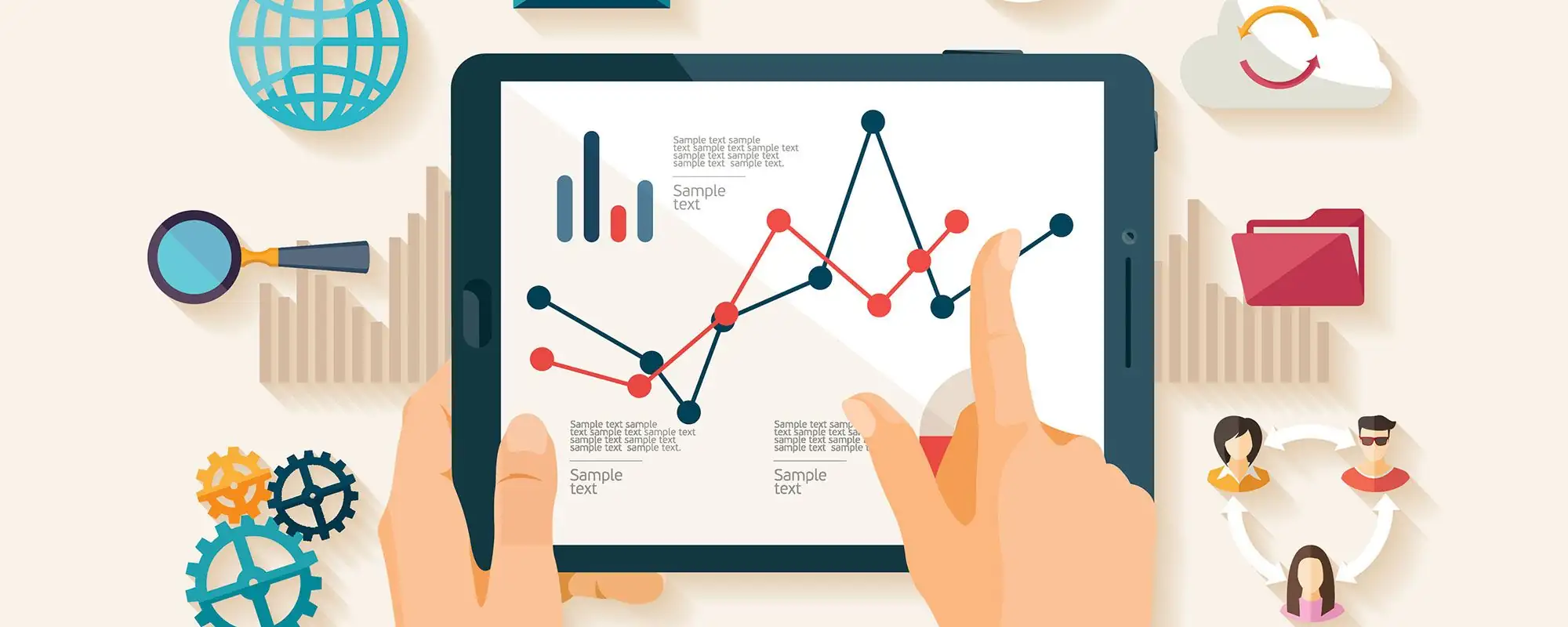
Continuing to our last blog https://www.labellerr.com/blog/these-10-datasets-you-wont-find-on-kaggle-part1/
Are you someone who deals with datasets every new day and are always looking for a new variety of datasets? If you are a data enthusiast, then CVPR ( IEEE/CVF Computer Vision and Pattern Recognition Conference (CVPR)) is something that is extremely important for you. Recently, they have published a list of 10 Datasets you might not find on Kaggle that might be of your interest if you are related to the Computer Vision industry.
Kaggle is for those interested in machine learning and data science. Users of Kaggle can work together, access and share datasets, use notebooks with GPU integration, and compete with several other data professionals to tackle data science problems.
Here we have listed 10 Datasets you might not find on Kaggle that might be of use to you. But first, let’s understand CVPR in detail.
6. NeRFReN: Neural Radiance Fields With Reflections
Author: Yuan-Chen Guo, Di Kang, Linchao Bao, Yu He, Song-Hai Zhang
Utilizing neural scene representations that are based on coordinates, Neural Radiance Fields (NeRF) has attained previously unheard-of vision synthesis quality. However, NeRF's view dependence only handles straightforward reflections, such as highlights, and is unable to handle more complicated reflections, including those off glass and mirrors. In these cases, NeRF simulates the digital representation as real geometry, which causes inaccurate depth estimates and fuzzy renderings whenever the multi-view consistency is broken because the reflected objects might only be visible from select viewpoints. They have presented NeRFReN, which is built atop NeRF to model scenes with reflections, in order to address these problems. To be more precise, they suggest that you divide a scene into reflected and transmitted components and model each component using a different neural radiance field.
They have used geometric priors and implemented well-planned training procedures to take advantage of this decomposition's significant under-constrained ness in order to provide acceptable decomposition results. Experiments on diverse self-shot scenes demonstrate that our approach yields physically sound depth estimate results and high-quality novel view synthesis while supporting scene editing applications.
7. YouMVOS: An Actor-Centric Multi-Shot Video Object Segmentation Dataset
Author: Donglai Wei, Siddhant Kharbanda, Sarthak Arora, Roshan Roy, Nishant Jain, Akash Palrecha, Tanav Shah, Shray Mathur, Ritik Mathur, Abhijay Kemkar, Anirudh Chakravarthy, Zudi Lin, Won-Dong Jang, Yansong Tang, Song Bai, James Tompkin, Philip H.S. Torr, Hanspeter Pfister
Multi-shot movies must be analyzed for many video understanding tasks, but the existing datasets for video object segmentation (VOS) only take into account single-shot videos. To solve this problem, we created a brand-new dataset called YouMVOS, which consists of 200 well-liked YouTube videos from ten different genres, each of which has an average runtime of five minutes and 75 shots. By identifying recurring actors and annotating 431K segmentation masks at a frame rate of six, we outperformed prior datasets in terms of average video time, object variation, and complexity of the narrative structure. To create competitive baseline approaches, we added best practices for memory management, multi-shot tracking, and model architecture design to an existing video segmentation method.
These baselines continue to struggle to account for cross-shot appearance variance on our YouMVOS dataset, according to error analysis. Our dataset thus presents fresh difficulties in multi-shot segmentation for more accurate video analysis.
The dataset:
The VGGFace2 dataset is made of around 3.31 million images divided into 9131 classes, each representing a different person's identity. The dataset is divided into two splits, one for the training and one for the test. The latter contains around 170000 images divided into 500 identities while all the other images belong to the remaining 8631 classes available for training. While constructing the datasets, the authors focused their efforts on reaching a very low label noise and a high pose and age diversity thus, making the VGGFace2 dataset a suitable choice to train state-of-the-art deep learning models on face-related tasks. The images of the training set have an average resolution of 137x180 pixels, with less than 1% at a resolution below 32 pixels (considering the shortest side).
The CASIA-WebFace dataset is used for face verification and face identification tasks. The dataset contains 494,414 face images of 10,575 real identities collected from the web.
YouTubeVIS is a new dataset tailored for tasks like simultaneous detection, segmentation and tracking of object instances in videos and is collected based on the current largest video object segmentation dataset YouTubeVOS.
You can download from here
Related Research Paper for your reference
Photo-Realistic Single Image Super-Resolution Using a Generative Adversarial Network
On CVPR 2017 by Christian Ledig, Lucas Theis, Ferenc Huszar, Jose Caballero, Andrew Cunningham, Alejandro Acosta, Andrew Aitken, Alykhan Tejani, Johannes Totz, Zehan Wang, Wenzhe Shi
VGGFace2: A dataset for recognising faces across pose and age published by Qiong Cao, Li Shen, Weidi Xie, Omkar M. Parkhi, Andrew Zisserman
MobileFaceNets: Efficient CNNs for Accurate Real-Time Face Verification on Mobile Devices published by Sheng Chen, Yang Liu, Xiang Gao, Zhen Han
YolactEdge: Real-time Instance Segmentation on the Edge published by Haotian Liu, Rafael A. Rivera Soto, Fanyi Xiao, Yong Jae Lee
8. Wnet: Audio-Guided Video Object Segmentation via Wavelet-Based Cross-Modal Denoising Networks
Author: Wenwen Pan, Haonan Shi, Zhou Zhao, Jieming Zhu, Xiuqiang He, Zhigeng Pan, Lianli Gao, Jun Yu, Fei Wu, Qi Tian
In visual analysis and editing, the difficult challenge of audio-guided video semantic segmentation involves autonomously separating foreground items from a background in a video sequence in accordance with the relevant audio expressions. Due to the lack of modeling the semantic representation of audio-video interaction contents, the existing referring video semantic segmentation studies, however, primarily concentrate on the guiding of text-based referring expressions. In this article, we approach the issue of audio-guided video semantic segmentation from the perspective of end-to-end denoised encoder-decoder network learning. We suggest using an encoder network built on wavelets to learn cross modal representations of video content using audio-form questions. We use a multi-head cross-modal attention strategy in particular to investigate any potential relationships between the video and the query contents.
The audio-video features are divided using a 2-dimension discrete wavelet transform. To filter out noise and outliers, we measure the high-frequency coefficient thresholds. After that, frequency domain transforms are used to create the target masks using a self-attention-free decoder network. In addition, to improve audio guiding, we optimise mutual information between the encoded features and multi-modal features. We also created the first extensive dataset for audio-guided video semantic segmentation. Extensive testing demonstrates the efficiency of our approach.
The dataset:
JHMDB is an action recognition dataset that consists of 960 video sequences belonging to 21 actions. It is a subset of the larger HMDB51 dataset collected from digitized movies and YouTube videos. The dataset contains video and annotation for puppet flow per frame (approximated optimal flow on the person), puppet mask per frame, joint positions per frame, action label per clip and meta label per clip (camera motion, visible body parts, camera viewpoint, number of people, video quality).
A2D (Actor-Action Dataset) is a dataset for simultaneously inferring actors and actions in videos. A2D has seven actor classes (adult, baby, ball, bird, car, cat, and dog) and eight action classes (climb, crawl, eat, fly, jump, roll, run, and walk) not including the no-action class, which we also consider. The A2D has 3,782 videos with at least 99 instances per valid actor-action tuple and videos are labeled with both pixel-level actors and actions for sampled frames. The A2D dataset serves as a large-scale testbed for various vision problems: video-level single- and multiple-label actor-action recognition, instance-level object segmentation/co-segmentation, as well as pixel-level actor-action semantic segmentation, to name a few.
You can download it from here
Related Research Paper for your reference
AVA: A Video Dataset of Spatiotemporally Localized Atomic Visual Actions published on CVPR 2018 by Chunhui Gu, Chen Sun, David A. Ross, Carl Vondrick, Caroline Pantofaru, Yeqing Li, Sudheendra Vijayanarasimhan, George Toderici, Susanna Ricco, Rahul Sukthankar, Cordelia Schmid, Jitendra Malik ·
2D Human Pose Estimation: New Benchmark and State of the Art Analysis published on CVPR 2014 by Mykhaylo Andriluka, Leonid Pishchulin, Peter Gehler, Bernt Schiele
Actor and Action Video Segmentation from a Sentence published on CVPR 2018 · by Kirill Gavrilyuk, Amir Ghodrati, Zhenyang Li, Cees G. M. Snoek
A Survey on Deep Learning Technique for Video Segmentation by Wenguan Wang, Tianfei Zhou, Fatih Porikli, David Crandall, Luc van Gool
9. Dynamic EarthNet: Daily Multi-Spectral Satellite Dataset for Semantic Change Segmentation
Author: Aysim Toker, Lukas Kondmann, Mark Weber, Marvin Eisenberger, Andrés Camero, Jingliang Hu, Ariadna Pregel Hoderlein, Çağlar Şenaras, Timothy Davis, Daniel Cremers, Giovanni Marchisio, Xiao Xiang Zhu, Laura Leal-Taixé
The audio-video features are divided using a 2-dimension discrete wavelet transform. To filter out noise and outliers, we measure the high-frequency coefficient thresholds. After that, frequency domain transforms are used to create the target masks using a self-attention-free decoder network. In addition, to improve audio guiding, we optimize the similarity matrix between the encoded characteristics and multi-modal features. They also create the first extensive dataset for audio-guided video semantic segmentation. Extensive testing demonstrates the efficiency of our approach.
In their experiments, we contrast a number of well-established baselines that either uses the daily observations as extra training data (semi-supervised learning) or numerous observations simultaneously (Spatio-temporal learning) as a point of reference for future study. Finally, they suggest the SCS, a novel assessment metric that specifically addresses the difficulties of time-series semantic change segmentation.
The dataset:
DOTA is a large-scale dataset for object detection in aerial images. It can be used to develop and evaluate object detectors in aerial images. The images are collected from different sensors and platforms. Each image is of size in the range from 800 × 800 to 20,000 × 20,000 pixels and contains objects exhibiting a wide variety of scales, orientations, and shapes. The instances in DOTA images are annotated by experts in aerial image interpretation by arbitrary (8 d.o.f.) quadrilateral. We will continue to update DOTA, to grow in size and scope to reflect evolving real-world conditions. Now it has three versions:
DOTA-v1.0 contains 15 common categories, 2,806 images and 188, 282 instances. The proportions of the training set, validation set, and testing set in DOTA-v1.0 are 1/2, 1/6, and 1/3, respectively.
Functional Map of the World (fMoW) is a dataset that aims to inspire the development of machine learning models capable of predicting the functional purpose of buildings and land use from temporal sequences of satellite images and a rich set of metadata features.
iSAID contains 655,451 object instances for 15 categories across 2,806 high-resolution images. The images of iSAID are the same as the DOTA-v1.0 dataset, which is mainly collected from Google Earth, some are taken by satellite JL-1, and the others are taken by satellite GF-2 of the China Centre for Resources Satellite Data and Application.
An open-source Multi-View Overhead Imagery dataset with 27 unique looks from a broad range of viewing angles (-32.5 degrees to 54.0 degrees). Each of these images covers the same 665 square km geographic extent and is annotated with 126,747 building footprint labels, enabling direct assessment of the impact of viewpoint perturbation on model performance.
You can download it from here
Related Research Paper for your reference
DOTA: A Large-scale Dataset for Object Detection in Aerial Images published on CVPR 2018 by Gui-Song Xia, Xiang Bai, Jian Ding, Zhen Zhu, Serge Belongie, Jiebo Luo, Mihai Datcu, Marcello Pelillo, Liangpei Zhang
DeepGlobe 2018: A Challenge to Parse the Earth through Satellite Images published by Demir, Krzysztof Koperski, David Lindenbaum, Guan Pang, Jing Huang, Saikat Basu, Forest Hughes, Devis Tuia, Ramesh Raskar ·
There is no data like more data -- current status of machine learning datasets in remote sensing published by Michael Schmitt, Seyed Ali Ahmadi, Ronny Hänsch ·
Path Aggregation Network for Instance Segmentation published on CVPR 2018 by Shu Liu, Lu Qi, Haifang Qin, Jianping Shi, Jiaya Jia ·
10. Big Dataset GAN: Synthesizing ImageNet With Pixel-Wise Annotations
Author: Daiqing Li, Huan Ling, Seung Wook Kim, Karsten Kreis, Adela Barriuso, Sanja Fidler, Antonio Torralba
Pixel-wise labelling of photographs is a time-consuming and expensive technique. Recently, Dataset GAN demonstrated a possible alternative: generating a limited group of manually labelled, GAN-generated images from a big labelled dataset using generative adversarial networks (GANs). Here, we scale the class diversity of Dataset GAN to that of ImageNet. In order to manually annotate 5 photos for each of the 1k classes, they have used image samples from the class-conditional generative model BigGAN that was trained on ImageNet. We transform BigGAN into a labeled dataset generator by training a powerful feature segmentation architecture on top of BigGAN.
They also demonstrated how VQGAN can generate datasets by using the already annotated data. By labeling an extra collection of 8k real photos, we establish a new ImageNet benchmark and assess segmentation performance under various conditions. They have demonstrated significant advances in using a large generated dataset to train several supervised and self-supervised backbone models on pixel-wise tasks through a thorough ablation study. Additionally, they have shown that pre-training with our synthetic datasets outperforms pre-training with regular ImageNet on a number of downstream datasets, including PASCAL-VOC, MS-COCO, Cityscapes, and chest X-ray, as well as tasks (detection, segmentation).
The dataset:
The MS COCO (Microsoft Common Objects in Context) dataset is a large-scale object detection, segmentation, key-point detection, and captioning dataset. The dataset consists of 328K images.
Splits: The first version of the MS COCO dataset was released in 2014. It contains 164K images split into training (83K), validation (41K) and test (41K) sets. In 2015 an additional test set of 81K images was released, including all the previous test images and 40K new images.
Based on community feedback, in 2017 the training/validation split was changed from 83K/41K to 118K/5K. The new split uses the same images and annotations. The 2017 test set is a subset of 41K images of the 2015 test set. Additionally, the 2017 release contains a new unannotated dataset of 123K images.
Cityscapes is a large-scale database which focuses on a semantic understanding of urban street scenes. It provides semantic, instance-wise, and dense pixel annotations for 30 classes grouped into 8 categories (flat surfaces, humans, vehicles, constructions, objects, nature, sky, and void). The dataset consists of around 5000 fine annotated images and 20000 coarse annotated ones. Data was captured in 50 cities during several months, daytime, and good weather conditions. It was originally recorded as video so the frames were manually selected to have the following features: a large number of dynamic objects, varying scene layouts, and varying backgrounds.
You can download it from here
Related Research Paper for your reference
MnasFPN: Learning Latency-aware Pyramid Architecture for Object Detection on Mobile Devices published on CVPR 2020 by Bo Chen, Golnaz Ghiasi, Hanxiao Liu, Tsung-Yi Lin, Dmitry Kalenichenko, Hartwig Adams, Quoc V. Le
Visual Representations for Semantic Target Driven Navigation published by Arsalan Mousavian, Alexander Toshev, Marek Fiser, Jana Kosecka, Ayzaan Wahid, James Davidson ·
MOSAIC: Mobile Segmentation via decoding Aggregated Information and encoded Context published by Weijun Wang, Andrew Howard ·
Searching for Efficient Multi-Scale Architectures for Dense Image Prediction published on NeurIPS 2018 by Liang-Chieh Chen, Maxwell D. Collins, Yukun Zhu, George Papandreou, Barret Zoph, Florian Schroff, Hartwig Adam, Jonathon Shlens
Book our demo with one of our product specialist
Book a Demo