The Ultimate Guide To Data Labeling Services in 2025
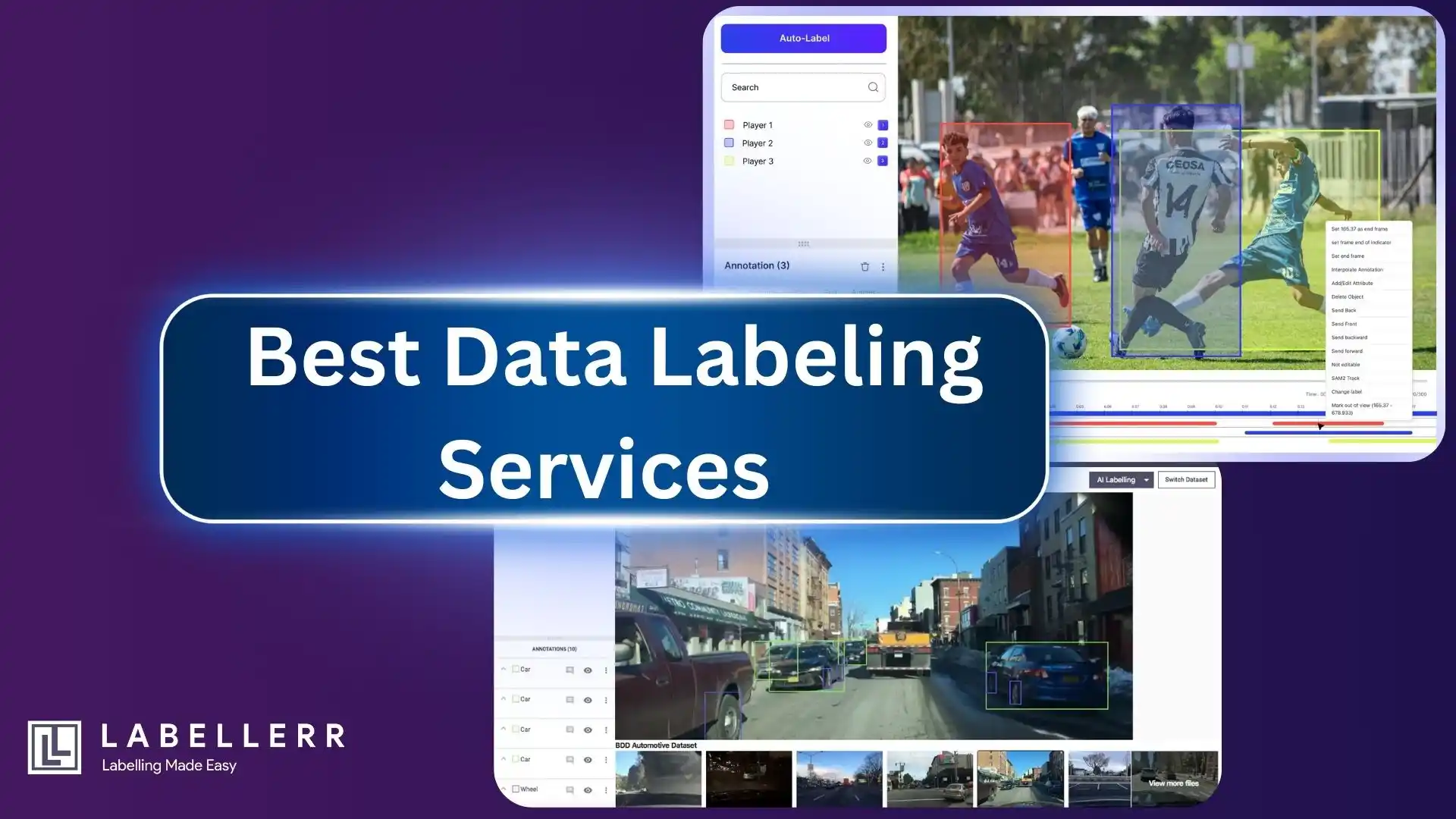
Imagine a self-driving car that can’t differentiate between a pedestrian and a street sign. Scary, right? This is the reality businesses and AI developers face when their models are fed with poor-quality or unlabeled data.
It’s like trying to solve a puzzle without knowing what the picture looks like.
According to a report by MarketsandMarkets, the global data labeling market is projected to reach $5.5 billion by 2027, growing at a compound annual rate of 29%. This shows how critical labeled data is for businesses aiming to deploy AI-powered solutions effectively.
If you're an AI enthusiast, business leader, or data scientist, you already know the challenges: messy data, inconsistent labeling, and the time it takes to clean and tag datasets.
These hurdles not only slow down your projects but also reduce the performance of your AI models.
This blog is your solution. It covers everything you need to know about data labeling services, from their types and features to the best practices and providers in 2024.
With these insights, you’ll be equipped to make informed decisions that can supercharge your AI projects. Let’s dive in!
Table of Contents
- What Are Data Labeling Services?
- Why Businesses Need Data Labeling Services
- Key Features to Look for
- Top Industries Benefiting
- Best Data Labeling Services in 2024
- How to Choose the Right Service
- How to Choose the Right Data Labeling Service for Your Business
- Case Studies
- Labellerr: Your Partner in Data Labeling
- Conclusion
- FAQs
What Are Data Labeling Services?
Data labeling is the process of tagging raw data—such as images, text, videos, or audio—with meaningful labels that help AI models learn and make accurate predictions. It’s like teaching a toddler to recognize objects by using flashcards.
Labeled data is the backbone of AI, enabling applications in healthcare, autonomous vehicles, retail, and more. Here’s a breakdown of the main types of data labeling, along with examples for each:
1. Image Labeling
Image labeling involves tagging objects, regions, or features in images. These labels help AI models identify objects, understand scenes, or perform tasks like object detection.
Example: In self-driving cars, image labeling is used to tag objects like pedestrians, vehicles, and traffic signs. For instance, labeling stop signs in various lighting conditions helps the car’s AI recognize and respond to them accurately.
2. Text Labeling
Text annotation focuses on tagging specific elements within textual data. This could include labeling entities, classifying sentiment, or identifying intent in sentences.
Example: In chatbots, text annotation tags customer queries to identify their intent (e.g., “Order status” or “Refund request”). This enables the bot to respond appropriately, improving the user experience. Annotating thousands of queries helps the bot learn and provide more accurate answers.
3. Video Labeling
Video labeling involves annotating objects, activities, or motion across video frames. This requires tracking objects as they move through different scenes.
Example: In sports analytics, video tagging is used to label player movements, ball trajectories, and game strategies. For instance, tagging a football player’s position in each frame helps analysts and coaches understand tactics and improve team performance.
4. Audio Transcription
Audio transcription converts spoken words or sounds into labeled text. It’s crucial for training speech recognition and audio analysis models.
Example: Voice assistants like Alexa or Google Assistant rely on audio transcription to interpret spoken commands.
For example, labeling audio clips with phrases like “Play music” or “Set an alarm” trains the assistant to recognize and respond to these commands accurately.
Manual vs. Automated Labeling
Manual labeling, done by humans, provides precision and handles edge cases effectively. For example, manually labeling medical images ensures that subtle anomalies are correctly tagged.
However, it is time-consuming and costly.Automated tools, on the other hand, are faster and scalable, using AI to pre-label data. They may struggle with complex or nuanced tasks but work well for repetitive tasks.
Combining both manual and automated approaches ensures the highest quality labeled data, delivering smarter and more reliable AI systems.
By understanding the specific requirements of your project, you can strike the right balance for optimal results.
Why Businesses Need Data Labeling Services
High-quality labeled data is the foundation of successful AI models. Without accurate labels, even the best algorithms fail to perform effectively.
Data labeling services provide businesses with the resources and expertise to overcome challenges like inconsistent data and time constraints.
1. Accelerate AI Development
Labeled data enables faster training of AI models, reducing the time it takes to deploy solutions. This helps businesses achieve results quickly while ensuring the accuracy and reliability of their AI systems.
2. Save Time and Costs
Outsourcing data labeling eliminates the need for businesses to create and manage in-house teams. Service providers are experienced in handling labeling tasks, reducing the overall cost and time spent on data preparation.
3. Ensure Scalability
Data labeling services are built to handle large volumes of data, allowing businesses to scale their AI projects without delays or inconsistencies. This ensures that as datasets grow, the quality remains consistent.
4. Improve Data Quality and Consistency
Professional data labeling services follow strict quality control processes, ensuring that the data used to train AI models is accurate and consistent. This is critical for models to deliver reliable results.
5. Focus on Core Goals
Outsourcing data labeling allows businesses to focus on developing AI models, improving algorithms, and scaling their operations. By leaving the data preparation to experts, businesses can prioritize innovation and growth.
Key Features to Look for in a Data Labeling Service
Choosing the right data labeling service is critical to ensure your AI projects are successful. Here are seven essential features to consider, with examples to illustrate their importance:
1. Accuracy and Quality Control
Accuracy is the cornerstone of any data labeling service. Reliable providers implement robust quality checks to ensure error-free labeled data.
2. Scalability for Diverse Data Types
The ability to handle various data formats like images, videos, text, and audio ensures the service can meet growing and changing project requirements.
3. Seamless Integration with AI/ML Pipelines
Services that integrate smoothly with your existing AI/ML workflows save time and reduce technical challenges.
4. Data Security and Compliance
Protecting sensitive data is non-negotiable. Choose a provider that adheres to global standards like GDPR or ISO certifications.
5. Expertise in Complex Tasks and Edge Cases
A reliable service should handle intricate tasks like labeling ambiguous or rare data scenarios with precision.
6. Customization Options
Flexibility to tailor labeling tasks to your specific project requirements ensures better outcomes.
7. Cost-Effectiveness Without Compromising Quality
Affordability is key, but it shouldn’t come at the expense of data quality. A good service strikes the right balance.
Top Industries Benefiting from Data Labeling Services
Data labeling services are critical across industries where AI and machine learning play a transformative role. Here’s a detailed look at how different sectors benefit, with examples to highlight their real-world impact.
1. Healthcare
Accurate data labeling is essential for medical imaging, diagnostics, and predictive analytics. Labeled datasets train AI models to detect diseases, analyze medical images, and even predict patient outcomes.
Example: Annotating X-rays and MRIs helps AI systems detect fractures or tumors with high accuracy, assisting radiologists in making quicker and more reliable diagnoses.
2. Automotive
The automotive industry uses data labeling extensively for autonomous vehicles and advanced driver-assistance systems (ADAS). Labeled datasets help identify road signs, pedestrians, vehicles, and lane markings.
Example: Video labeling of traffic scenarios allows self-driving cars to recognize and react to obstacles, making roads safer.
3. Retail
In retail, data labeling supports product categorization, recommendation engines, and customer sentiment analysis. It enhances inventory management and personalizes the shopping experience.
Example: Labeling product images for an e-commerce platform ensures accurate search results, boosting customer satisfaction and sales.
4. Agriculture
In agriculture, labeled drone imagery helps monitor crop health, detect pest infestations, and optimize irrigation. AI models use this data to improve yield and reduce resource wastage.
Example: Annotating aerial images to highlight pest-affected areas helps farmers take timely action, preventing large-scale crop damage.
5. Security and Surveillance
Security systems rely on labeled video and image data to detect suspicious activities, recognize faces, and monitor crowded areas.
Example: Labeling video feeds helps AI-powered surveillance cameras identify unauthorized access or unusual behavior in real-time, enhancing safety.
6. Sports
AI in sports uses labeled data to analyze player movements, strategies, and game highlights. This improves coaching decisions and fan engagement.
Example: Video tagging in football allows teams to analyze player positioning and tactics, leading to improved performance on the field.
7. Manufacturing
In manufacturing, labeled datasets support quality control, defect detection, and predictive maintenance. AI systems trained on these datasets improve operational efficiency and reduce downtime.
Example: Annotating images of defective and non-defective products helps factories automate quality checks, ensuring consistent product standards.
Best Data Labeling Services in 2024
1. Labellerr
Labellerr stands out for its precision, scalability, and seamless integration capabilities. It supports a wide range of industries, including healthcare, retail, and manufacturing. Labellerr offers a user-friendly platform that balances manual and automated labeling, ensuring high-quality results at an affordable cost.
Top Features:
- Combines manual and automated labeling for flexibility.
- Scalable solutions for diverse industries.
- Strong focus on data security and compliance.
- Easy integration with AI/ML pipelines.
Pros:
- Suitable for both startups and enterprises.
- High-quality results at cost-effective rates.
- User-friendly interface.
Cons:
- Limited advanced collaboration tools.
- Slightly fewer pre-built models compared to competitors.
G2 Review:"Labellerr provides excellent value for money, offering both scalability and quality. Great for businesses of all sizes."
2. Clarifai
Clarifai is an AI-powered platform offering robust data labeling and automation features. It specializes in providing solutions for large-scale data processing with a strong focus on visual recognition tasks.
Its advanced AI tools help automate the labeling process, making it faster and more efficient. However, the pricing may be a challenge for smaller teams or startups.
Top Features:
- AI-powered labeling with pre-built models.
- Handles diverse data types like images, videos, and text.
- Scalable for large datasets.
- Offers API integrations for seamless workflows.
Pros:
- High efficiency with automated workflows.
- Suitable for enterprise-scale projects.
- Powerful visual recognition tools.
Cons:
- Expensive for small teams.
- Limited manual labeling customization options.
G2 Review:"Clarifai is powerful for handling large datasets, but the pricing structure could be more flexible for smaller businesses."
3. Labelbox
Labelbox focuses on collaborative data labeling, making it ideal for teams working on large projects. The platform provides an intuitive interface, robust quality control features, and integrations with popular machine learning tools. It excels in scenarios requiring constant feedback and team collaboration.
Top Features:
- Collaborative tools for teamwork.
- Supports images, text, and video labeling.
- Quality assurance features to ensure accuracy.
- Seamless integrations with AI/ML workflows.
Pros:
- Easy-to-use interface.
- Excellent for collaborative projects.
- Strong quality control options.
Cons:
- Limited automation for repetitive tasks.
- Can be costly for smaller datasets.
G2 Review:"The platform is user-friendly and great for collaboration, but it could improve automation for repetitive labeling tasks."
4. SuperAnnotate
SuperAnnotate is known for its focus on annotation workflows, delivering consistent quality for complex labeling tasks. It supports images, videos, and text, with powerful collaboration features. The tool is a favorite among teams handling detailed projects like medical imaging or autonomous vehicles.
Top Features:
- Advanced workflow management for large projects.
- Customizable tools for detailed annotations.
- Collaboration features for team efficiency.
- Strong quality control mechanisms.
Pros:
- Excellent for complex and detailed labeling tasks.
- High-quality annotation tools.
- Strong focus on quality assurance.
Cons:
- Slightly steep learning curve for new users.
- Limited automation features.
G2 Review:"SuperAnnotate excels in handling detailed projects, but onboarding takes time due to the platform’s complexity."
5. Label Your Data
Label Your Data provides tailored data labeling services for businesses with specific needs. Its strength lies in offering customized solutions for niche industries like agriculture, finance, and healthcare. The platform is designed for companies that require a hands-on approach to meet unique project requirements.
Top Features:
- Customizable solutions for niche industries.
- Expert teams for manual labeling.
- High-quality annotations tailored to specific use cases.
- Focused on data security and compliance.
Pros:
- Excellent for niche and specialized industries.
- High customization flexibility.
- Ensures high data quality.
Cons:
- May not be cost-effective for smaller projects.
- Limited scalability for large datasets.
G2 Review:"Label Your Data is a go-to for custom solutions, but scalability could be better for larger datasets."
How to Choose the Right Data Labeling Service for Your Business
Selecting the right data labeling service is critical for building accurate and reliable AI models. Here’s a step-by-step guide to help you make an informed decision:
1. Define Your Data Requirements
- Identify the type of data you need labeled: images, text, video, or audio.
- Specify the level of detail required, such as bounding boxes for objects or sentiment in text.
2. Understand Your Project Goals
- Clarify how the labeled data will be used in your AI/ML project.
- Determine if you need high accuracy for sensitive use cases (e.g., healthcare) or speed for rapid prototyping.
3. Assess Scalability Needs
- Consider the volume of data you need labeled now and in the future.
- Choose a provider that can scale operations as your dataset grows.
4. Check Quality Assurance Processes
- Look for services that have strict quality control measures, such as multi-layer reviews.
- Ensure they can handle complex cases and maintain accuracy.
5. Evaluate Domain Expertise
- Choose a service familiar with your industry (e.g., medical labeling for healthcare or video annotation for autonomous vehicles).
- Domain-specific knowledge ensures better quality and relevance.
6. Consider Integration Capabilities
- Ensure the service integrates smoothly with your existing AI/ML tools and workflows.
- Look for APIs and automation features that save time and effort.
7. Prioritize Data Security and Compliance
- Verify that the provider complies with global standards like GDPR, HIPAA, or ISO.
- Ensure they have measures in place to protect sensitive data.
8. Compare Pricing and Value
- Understand the pricing structure: is it based on labels, hours, or volume?
- Balance cost with the quality and features offered. Avoid going for the cheapest option if it compromises results.
9. Request a Trial Run
- Ask for a small trial project to evaluate accuracy, turnaround time, and overall performance.
- Use the trial to test their ability to meet deadlines and handle edge cases.
10. Review Feedback and Client Testimonials
- Look for reviews, ratings, and case studies to understand how well they’ve performed for other clients.
- Check platforms like G2 or Capterra for unbiased feedback.
11. Evaluate Turnaround Time
- Assess their ability to meet deadlines, especially for time-sensitive projects.
- Ensure they can scale resources to avoid bottlenecks.
12. Check Customer Support and Communication
- Choose a provider with responsive customer support.
- Good communication ensures that issues are resolved quickly and that the service adapts to your evolving needs.
13. Confirm Flexibility and Customization
- Ensure the provider can customize workflows to fit your project’s unique requirements.
- Flexibility is essential for niche industries or specialized use cases.
14. Measure ROI Potential
- Assess how the service improves your AI model’s performance and reduces your internal workload.
- A reliable provider should deliver measurable value over time.
Case Studies
Data labeling services are crucial for training accurate AI models, directly impacting business performance.
Here are two detailed case studies showcasing how companies have leveraged these services to enhance their AI capabilities and achieve significant returns on investment (ROI).
1. Kinetic Business Solutions and a Leading Computer Vision Company
A prominent computer vision company faced challenges with the labor-intensive task of data labeling, which was diverting resources from core operations and hindering AI model development.
Solution: The company partnered with Kinetic Business Solutions to outsource their data labeling needs. Kinetic provided scalable solutions, strict quality control, and allowed the client to refocus on primary business functions.
Results:
- Increased Efficiency: Accelerated AI model training and deployment.
- Improved Quality: Enhanced performance of AI models due to high-quality labeled data.
Source: Kinetic Business Solutions Case Study
2. RTB House's Implementation of Deep Learning Algorithms
Problem: RTB House aimed to enhance its retargeting solutions by improving user modeling without manual intervention.
Solution: In 2017, RTB House integrated deep learning algorithms, specifically long short-term memory (LSTM) neural networks, to autonomously understand user behavior.
Results:
- Enhanced User Modeling: Achieved a more accurate understanding of users, leading to improved ad targeting.
- Business Growth: Expanded operations across Europe, Australia, Asia, and the U.S., with revenues reaching €99.89 million in 2018.
Source: RTB House Wikipedia
Labellerr: Your Partner in Data Labeling
At Labellerr, we specialize in solving your data labeling challenges—whether you’re working on medical imaging, retail optimization, autonomous vehicles, or more.
Facing messy datasets or tight deadlines? Labellerr offers precision, scalability, and seamless integration to get your projects back on track.
From startups to enterprises, we’ve empowered businesses with high-quality labeled data that enhances AI model performance and saves time.
What’s Next?
- Start Small: Test our platform with a trial project to see the difference Labellerr can make.
- Talk to Us: Share your specific challenges, and we’ll craft a tailored solution for your needs.
- Scale with Confidence: Let Labellerr handle your data so you can focus on building impactful AI solutions.
Take the Next Step
Start your free trial today or schedule a demo to experience how Labellerr can elevate your AI projects.
Conclusion
Choosing the right data labeling service is essential for building accurate AI models and achieving your goals. High-quality labeled data saves time, reduces costs, and improves the performance of your AI projects.
Stay ahead by embracing the latest trends like AI-assisted labeling and scalable solutions. Whether you’re a startup or an enterprise, outsourcing data labeling lets you focus on innovation while experts handle the data.
Take the next step today. Explore trusted providers like Labellerr to transform your raw data into actionable insights and elevate your AI projects to the next level!
FAQs
What are the common challenges in data labeling services?
Maintaining accuracy, handling large datasets, and managing edge cases can be tricky. Tools like Labellerr simplify these challenges by offering scalable, high-quality solutions.
How much do data labeling services typically cost?
Costs vary by task complexity and volume, ranging from a few cents per label to premium pricing for niche projects. Always compare providers to find the best value.
Can small businesses benefit from data labeling services?
Absolutely! Self-service tools and affordable providers make it easy for small businesses to access top-notch labeling. A small retail client we worked with improved search accuracy by 30% using tailored labeling services.

Simplify Your Data Annotation Workflow With Proven Strategies
Download the Free Guide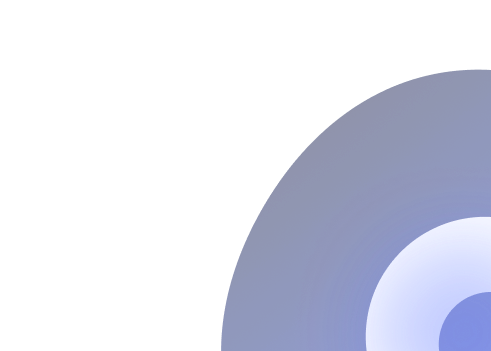