The next big thing in data annotation
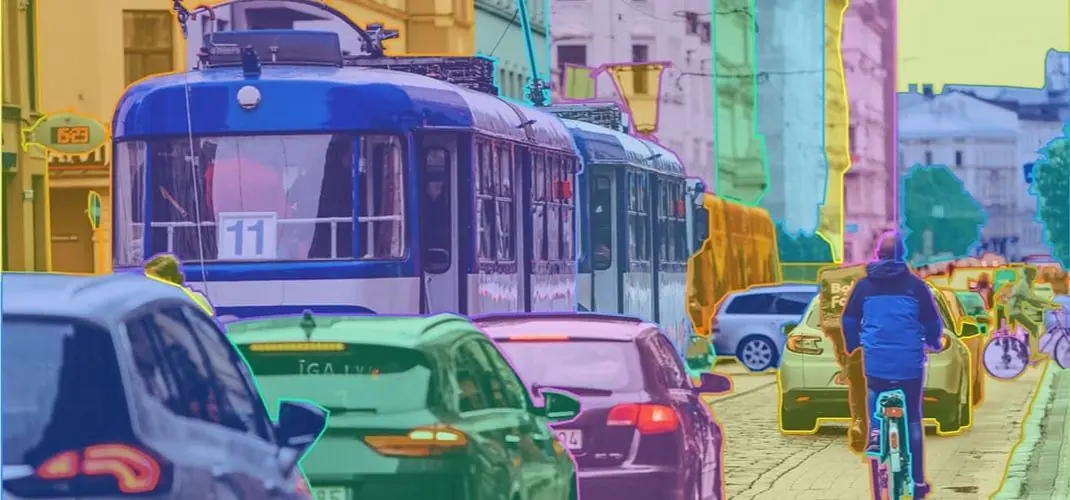
Data enthusiasts use a variety of datasets while creating ML models, carefully adjusting each one to the model's training needs. Robots can therefore recognize content that has been labelled in a wide range of understandable formats, including images, texts, and videos.
In order to train their algorithms to recognize recurring patterns and produce precise estimations and projections, AI and machine learning companies are searching for annotated data and annotation services to incorporate into their algorithms.
The rapid use of AI has impacted our current reality and the digital environment for contemporary businesses. And when we developed the ability to gather and analyze data at previously unheard-of rates, the function of data annotation was largely neglected. The time to make things right is now.
Today, we'll go even further into this subject and see what the AI community and data annotation experts have in store for us in the upcoming year.
About data annotation
Data annotation is the process of labeling information for usage by machines. In supervised learning, in which the system uses labelled datasets to interpret, comprehend, and understand input patterns to produce desired outputs, it is very helpful.
For a particular use case, training data should be correctly categorized and annotated. Business organizations may create and enhance AI solutions that include high-quality, human-powered data annotation.
Read here: How to super fast data annotations process with automation?
What variables affect current trends in data annotation?
Data annotation advances significantly in 2022 and further integrate into the current digital environment. The development of mobile computing platforms and digital image processing is the primary cause of these changes.
What's the point of data annotation in these applications, and how does it function?
- Customer experience improvement through digital commerce.
- Verification of documents and real-time consumer engagement in banking, finance, and insurance.
- Parsing a large number of gathered and unstructured datasets is research.
- Social media: identifying inappropriate content, content curation, and monitoring.
- The sector of agriculture: evaluation of the soil, crop monitoring, etc.
This is not a complete list of the variables influencing the developments in data annotation, though. The incredible expansion of digital content throughout all business platforms is another reality to take into account. It implies handling massive user data, such as photographs, videos, and text, over a variety of digital channels. Here, data annotation enables companies to maximize the advantages of online information, add value, and attract new clients.
A data-centric design is also being mandated by businesses this year. Data is the most important resource to implement and sustain an efficient enterprise architecture, according to data centricity, which is both a way of thinking and a technical design. To decrease the high time and expense of human annotation, this calls for a shift toward automated choices and a more intelligent workforce for data labeling.
Another factor influencing the growth of the data labeling market in 2022 is the explosion of picture annotation tools in the commercial, automotive, and healthcare sectors. The necessity to increase the value of data used in these businesses by applying labeling technology is the reason for the explosive growth in the demand for annotation tools.
Last but not least, in order for AI projects to be practicable and successful, highly effective data is needed in the areas of IoT, ML, DL, robotics, fraud detection systems, predictive analytics, and recommender systems. This is arguably the most significant element this year causing advances in data labeling. It's time to discuss the most recent trends in this sector now.
Present and future data annotation expediting strategies
We can expect big changes in the global AI business this year as a result of the high prospects for data labeling this year. Additionally, we might anticipate a drop in manual annotation methods as intelligent algorithms become more prevalent as data annotation services spread their wings.
Data annotation, whether automatic or manual, is essential for enhancing the reliability, validity, security, and usefulness of data in a variety of settings and across a wide range of businesses. But with change comes the necessity to use fresh approaches to fortify the industry and guarantee that the most recent developments are properly included in the world's data labelling ecosystem.
In addition to conventional (also known as manual) data annotation techniques, the market is now using a new scenario-
Unsupervised Learning: In the upcoming years, supervised learning will still be the most widely used form of machine learning, predicts Gartner. On the other side, clustering will become more prevalent as unsupervised learning gains traction (unsupervised ML method). Clustering algorithms are essential for decreasing manual input and delivering quicker results given the large volume of data.
Manifold and Contrastive Learning: ML learning methods for unlabeled datasets. Since these methods use models that do encodings and embedding, they are not dependent on labelled data. Google, as an illustration, has used contrastive learning on the Wikipedia site.
AI with neural symbols: Modern DL techniques with traditional methods form the foundation of a new branch of AI research. As a result, there is less need to classify data and more reliance on statistical and conceptual frameworks. By using this method, engaging subject-matter experts to annotate data can be avoided, saving time and money.
As a general rule, ML usually depends on supervised learning since it needs labelled training data in order to create powerful predictive models. The methodologies and trends stated above to call for the utilization of unsupervised learning and labelled training data, nonetheless. If unsupervised machine learning doesn't involve annotation, why do we mention it?
Unsupervised learning can considerably enhance supervised machine learning, improving the performance of the entire ML system. The following trends will call for the use of labelled data:
- AI in Medicinal Industry: With improvements in medical imaging and CV technologies, data annotation will continue to revolutionise healthcare as it has done so in the past. By strengthening medical applications powered by AI and minimising human involvement, data labelling tools will maintain their leadership position in the market. Additionally, it will improve industrywide capacities for conducting medical research.
- Cloud: In 2022, the market for data collection and annotation, encompassing digital advertising channels and e-commerce, will be shaped by the increasing need for cloud-based services. To extract useful information and obtain insights into their online behaviour, they need tagged data. The growth of AI platforms housed in the cloud will improve annotation for face recognition, object recognition, landmark detection, etc.
- Social Media: Data annotation will be an effective tactic for managing data dissemination and micro-communications, as well as for assessing emotional transmission on social media platforms like Twitter and Facebook. For social media monitoring, intelligent and safe data labeling procedures are also necessary for visual listening and analytics.
- Active Learning: To assure the most accurate results with the fewest labels, a novel method for training ML models concurrently builds and annotates training datasets. Active learning necessitates the use of a clever data annotation strategy that combines labelled and unlabeled data.
- Auto ML: a popular subject that will aid in the creation of sophisticated data labeling systems for the automatic improvement of neural network architectures. Building ML models will be safer thanks to the annotation process being automated.
- Synthesis AI: In 2023, it's anticipated that the Metaverse virtual phenomena would permeate the majority of significant organizations. To create such virtual spaces, a lot of synthetic data is needed. To put it simply, it is a 3D dataset that cannot be accurately classified manually by humans. However, the primary benefit of this approach is the automatic generation and annotation of synthetic data.
The final outcomes — data quality, privacy, and scalability — are what matter most, whether AI or human annotation is used. The dynamic automation-driven change of the labeling sector will completely reshape the digital economy and business models.
The future of business is cognitive automation, powered by expert data annotation. As a result, in the context of the Worldwide AI economy in 2023, data annotation will be a contentious topic.
Will data annotation remain the key to AI's future?
Lastly, machine learning data annotation has quickly developed into a brand-new industry. As a result, organizations looking to capitalize on the current AI boom are focusing their efforts on data labeling service providers like Label Your Data.
Beginning this year, the market for data annotation will grow significantly, creating more chances for AI, ML, and deep learning to pervade every aspect of business and our daily lives. We can now confidently assert that the painstaking and highly specialized process of qualified data annotation offers the key to the advancement of AI.
You must seek the aid of qualified data annotators if you are thinking about developing your own original AI project. Time is a precious resource, so don't squander it and get in touch with us for additional help!
Check out our blogs for more amazing information!
Book our demo with one of our product specialist
Book a Demo