6 Smart Animal Livestock Management Using Vision AI
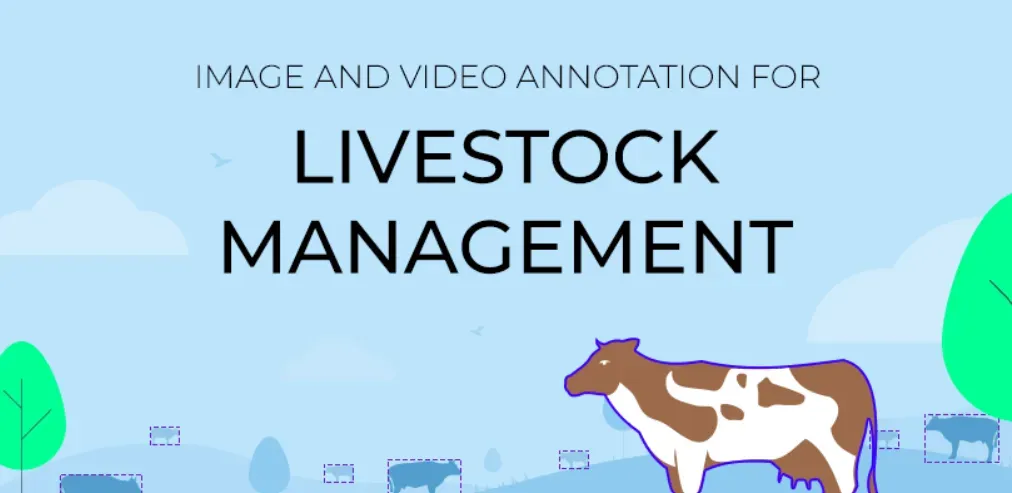
In the face of pressing challenges like climate change and supply chain instability, food producers, particularly those in livestock management, are embracing cutting-edge technology to ensure a sustainable future.
This blog delves deep into the profound impact of AI in livestock management, emphasizing the indispensable role of data annotation in creating accurate training datasets.
We will explore a range of use cases where data annotation is revolutionizing the world of animal livestock management and discuss how innovative tools are reshaping the industry.
Table of Contents
- The Impact of AI on Livestock Management
- The Crucial Role of Data Annotation in Livestock Management
- Innovative Annotation Tools
- The Need for Efficient Data Annotation in Animal Livestock Management
- LabelGPT: Transforming Data Annotation
- Conclusion
The Impact of AI on Livestock Management
The integration of AI in livestock management is not just a technological advancement but a necessity for a resilient and productive future. AI-driven solutions are poised to boost yields, enhance animal welfare, and optimize efficiency, thereby securing the future of this critical industry.
1. Aerial Management: Among the most remarkable applications of AI in livestock management is the deployment of computer vision-equipped drones. These drones autonomously survey animal populations across expansive fields and farms. Their significance lies in their capacity to cover large areas and access remote locations. Integrated with AI systems, drones can promptly alert herd managers when an animal is missing, bolstering the safety and security of the herd.
2. Monitoring Health and Wellbeing: AI models play a pivotal role in monitoring the health of livestock and identifying signs of illness or injury. These models are trained to recognize abnormal movements, often the initial indicators of health problems among animals. This early detection empowers herd managers to intervene swiftly, mitigating the spread of disease and enhancing overall animal welfare.
3. Feeding Rates: AI models are also employed to track feeding rates by identifying when an animal is bending down to eat. This technology enables herd managers to closely monitor each animal's health by assessing their feeding patterns. A decrease in feeding rates can be a signal of poor health, enabling early intervention.
Vision AI use cases are as follows:
4. Precision agriculture: Vision AI can be used to monitor livestock and their environment in real-time to collect data on feeding, drinking, movement, and behavior. This data can be used to optimize farm management practices, such as feeding schedules and grazing patterns.
5. Disease detection: Vision AI can be used to identify signs of disease and injury in livestock early on. This can help to reduce the spread of disease and improve animal welfare.
6. Animal welfare: Vision AI can be used to monitor animal behavior and identify signs of stress, discomfort, and pain. This information can be used to improve animal welfare and reduce the need for human intervention.
Here are some specific examples of Vision AI in action:
- Drones equipped with cameras can be used to inspect livestock herds for signs of disease or injury.
- Cameras installed in barns and other livestock facilities can be used to monitor animal behavior and identify signs of stress or discomfort.
- Computer vision algorithms can be used to analyze images and videos of livestock to identify their body condition, weight, and other health indicators.
- Vision AI can be used to automate the sorting and grading of livestock products.
The Crucial Role of Data Annotation in Livestock Management
The efficacy of AI in livestock management heavily depends on the availability of precise training datasets. Data annotation, the process of meticulously labeling and categorizing data for machine learning, plays an indispensable role in generating these datasets.
Various annotation methods are employed in the livestock management AI sector to craft high-quality training data:
1. Polygon Annotation: Irregular shapes, particularly animals, are meticulously annotated using polygon annotation. This method is essential for identifying and tracking animals in training images and videos.
2. Skeletal Annotations: Skeletal annotation simplifies the representation of animal movements in training videos. It involves creating a simplified version of the animal, facilitating AI models' recognition of movement patterns.
3. Semantic Segmentation: Semantic segmentation entails assigning a class to every pixel in an image or video frame. In livestock training data, it is utilized to outline and label specific objects, such as cows in a field.
4. Instance Segmentation: Going a step further, instance segmentation identifies each individual instance of a particular class. For instance, in an image of cows in a field, each cow is individually labeled and colored.
Innovative Annotation Tools
To craft high-quality training datasets for innovative livestock monitoring systems, annotation tools such as LabelGPT are indispensable. These tools offer unique and innovative features that streamline and enhance the annotation process:
- Integration of Custom Workflow Automation Code: Annotation tools facilitates the seamless integration of custom-written workflow automation code, which enhances efficiency and precision during annotation.
- Efficient Workflow Systems: The workflow systems within tools foster seamless collaboration between annotators and verifiers, ensuring tasks are distributed efficiently while maintaining high data quality.
- Object Interpolation: A standout feature of annotation tools is object interpolation, automating the tracking of livestock across multiple video frames. This not only heightens accuracy but also expedites the video annotation process.
The Need for Efficient Data Annotation in Animal Livestock Management
Livestock management is a complex and dynamic field where precision and efficiency are paramount. As discussed in our previous blogs, AI has rapidly become an invaluable asset in this sector, with applications ranging from aerial management to health monitoring and feeding rate tracking. To harness the full potential of AI in livestock management, the creation of precise training datasets is essential. This is where data annotation comes into play.
LabelGPT: Transforming Data Annotation
LabelGPT is a powerful prompt-based labelling tool that promises to be a game-changer in data annotation, particularly in the context of animal livestock management. Here's how LabelGPT works and the benefits it offers for this industry:
How LabelGPT Works
Import Your Data: LabelGPT simplifies the data import process. You can effortlessly connect your images from various sources, including popular cloud services like AWS, GCP, Azure, and APIs, or work with local data directly on our platform.
Give Prompt: Specify the classes or objects that require labelling by using text prompts. Choose your desired labelling type, whether it's bounding boxes or segmentation. This streamlined process significantly reduces the effort required for manual annotation.
Review the Labels: In just a matter of minutes, LabelGPT will provide you with labelled images, complete with confidence scores. This enables you to swiftly assess the quality of labels and seamlessly integrate the data into your machine learning training engine.
Benefits of LabelGPT
No Manual Labeling: LabelGPT's foundation model-powered labelling engine is capable of detecting and segmenting labels based on simple class/object names. This revolutionary feature offers complete zero-shot labelling, eliminating the need for manual annotation. In the context of livestock management, where labelling can be particularly challenging due to the dynamic nature of animals, this is a game-changer.
Thousands of Labels in Minutes: Bid farewell to the laborious task of labelling images one by one. With LabelGPT, you can label all your data with a single prompt. This not only saves valuable time but also ensures consistent and efficient data annotation.
Easy Review: LabelGPT goes a step further by providing confidence scores for the labeled images. This feature empowers you to quickly validate the labels, ensuring high-quality results for your machine learning pipeline. In the context of livestock management, where precision is critical, this feature is invaluable.
LabelGPT in Animal Livestock Management
So, how does LabelGPT fit into the world of animal livestock management? As we've explored in previous blogs, AI plays a pivotal role in transforming the industry. From monitoring animal health to assessing feeding rates, AI-driven solutions are poised to revolutionize the sector. However, the success of these solutions hinges on the availability of accurate training datasets. This is where LabelGPT excels.
By streamlining the data annotation process, LabelGPT enables livestock managers to create high-quality training datasets with remarkable speed and precision. The no-manual-labeling approach is particularly beneficial in this context, where the dynamic behavior of animals can make manual annotation both time-consuming and error-prone. With LabelGPT, the process becomes efficient and consistent.
Conclusion
As the global population continues to burgeon, the demand for sustainable and efficient food production, including meat and eggs, intensifies. Livestock management is at the intersection of technological innovation and agricultural necessity. AI-driven solutions, underpinned by accurate training datasets generated through data annotation, are reshaping the future of this industry.
These solutions promise increased yields, improved animal welfare, and enhanced efficiency, all of which are vital in an era marked by climate change and supply chain instability.
The adoption of AI in livestock management is not merely a technological trend; it is an imperative for a resilient and sustainable future. LabelGPT is not just a tool; it's a game-changer for data annotation in animal livestock management.
By offering speed, precision, and a no-manual-labeling approach, it paves the way for seamless AI integration in the industry.
Frequently Asked Questions
1.Can AI improve livestock production?
Machine learning algorithms play a crucial role in the field of precision livestock farming, where they are employed to enhance the efficiency of monitoring animal behavior and well-being, forecast the occurrence of disease outbreaks, and fine-tune feeding schedules.
2. Can computer vision be used for animal monitoring?
Utilizing autonomous systems for animal observation have demonstrated the potential to achieve accuracy levels that rival or even surpass human performance. The use of computer vision for animal monitoring presents the opportunity for unobtrusive surveillance in the context of livestock farming, which is essential in meeting the world's increasing demand for food products.
3. How is computer vision used in livestock farming?
Computer vision techniques are widely utilized within the realm of precision livestock farming (PLF), serving to maximize both the productivity and well-being of each individual animal. These systems for monitoring livestock supply up-to-the-minute data and support farmers in formulating strategic choices.
4. Can vision AI be used in cattle counting?
Plainsight has partnered with JBS USA, one of the world's leading food companies, to incorporate vision AI technology into its operational procedures. The initial solutions to be put into practice include using vision AI to count cattle.
Book our demo with one of our product specialist
Book a Demo