Limitations Of Small ML Team's Data Annotation? How to Solve It?
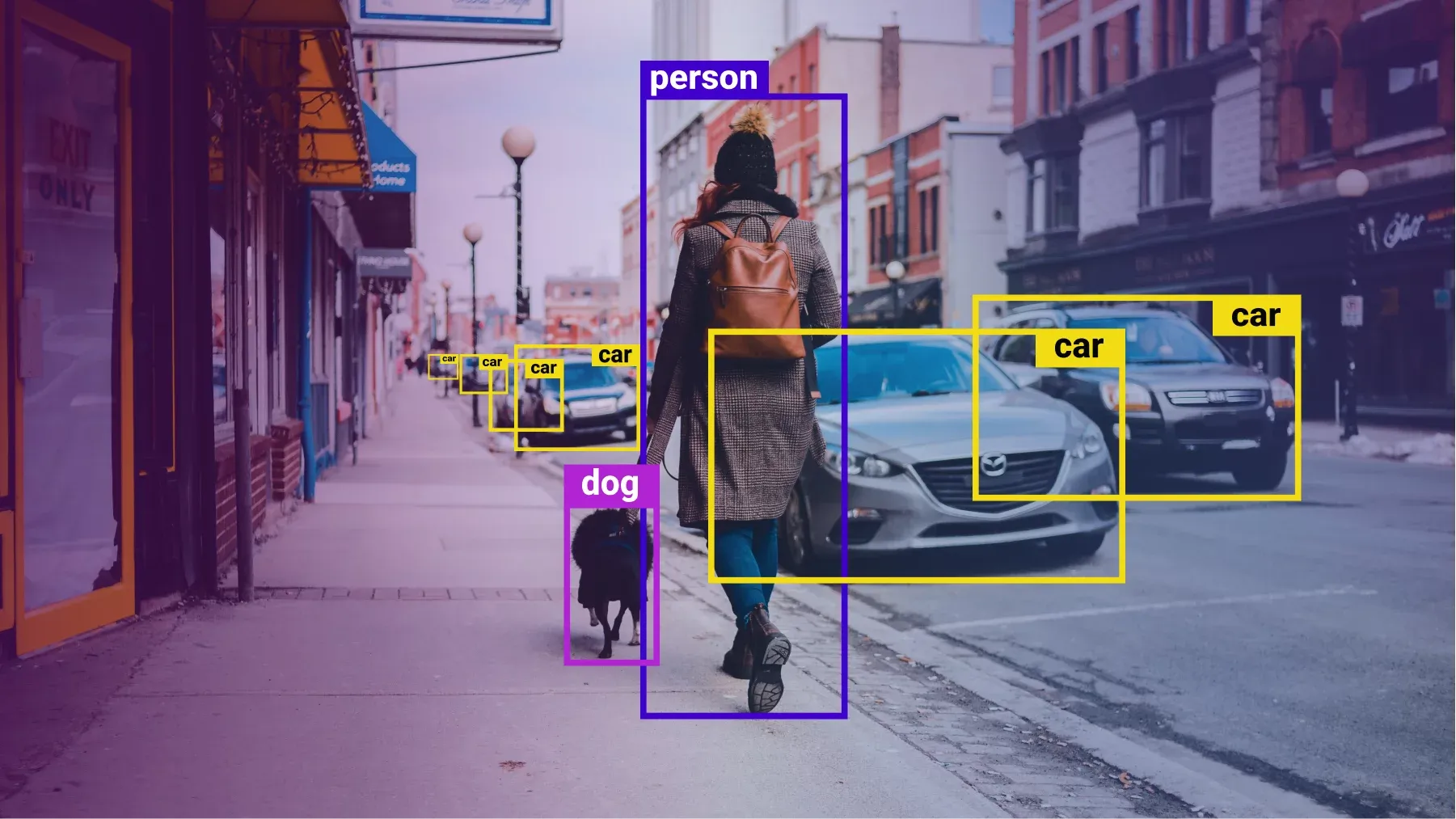
In the fast-paced field of artificial intelligence (AI), startups are emerging as significant actors, pushing innovation and challenging existing businesses. These tiny AI-based firms are using the strength of machine learning algorithms to create cutting-edge products that have the potential to revolutionize several industries.
To train AI models to produce accurate and trustworthy results, data annotation is a crucial procedure that frequently presents difficulties for these firms.
Data annotation is the process of labeling and categorizing massive amounts of data to build annotated datasets that will be used to train AI systems. While large organizations can afford to create dedicated data annotation teams, small AI-based businesses frequently suffer resource limits, making it critical for them to develop efficient and cost-effective solutions to conduct this critical activity.
In this blog, we will look at how small AI-based firms are solving the data annotation dilemma and offer insight into the issues they face. We will discuss the significance of high-quality annotations, the methodologies used by startups to undertake data annotation, and the possible problems that they can encounter along the road.
Methods Used by Small AI-based Startups for Data Annotation
Small AI-based businesses frequently use affordable and effective data annotation techniques to train their machine learning models. Spreadsheets and open-source data annotation software are two often employed techniques.
1. Spreadsheet
Small AI-based businesses frequently train their machine learning models using spreadsheets and open-source annotation tools. They start by setting up their raw data in a spreadsheet, with each row denoting a data instance and each column denoting a distinct category of annotation.
Startup annotators manually annotate each data instance by reviewing it and filling up the associated annotation fields with labels, bounding boxes, and textual descriptions as necessary.
To ensure correctness, quality assurance procedures are used, such as cross-checking annotations and defining standards. Startups export the annotated data from the spreadsheet in an appropriate format for training their models after the annotations are finished.
2. Open Source Annotation Tool
Alternatives use open-source annotation tools, which offer sophisticated functionality and automated capacities. Startups often automate and scale their annotation operations by establishing the annotation workflow, customizing the tool's user interface, and utilizing collaboration and automation capabilities.
The tool then allows the annotated data to be exported in well-known formats for model training, such as COCO or TFRecord. Small businesses can easily annotate their data and develop precise machine learning models using these techniques.
Challenges of Using Inaccurate Data Labeling Methods
Small AI-based startups can face several challenges when performing data annotation with inaccurate data labeling methods. Here are some key challenges they can encounter:
1. Poor Data Quality
Inaccurate data labeling methods can result in poor-quality labeled data. This can include incorrect labels, inconsistent annotations, or labeling errors. Poor data quality hampers the training process and can lead to AI models with low accuracy and unreliable performance.
2. Bias and Skewed Results
Inaccurate data labeling methods can introduce bias into the training data. If the labeling process is influenced by the biases of the labelers or if the data used for labeling is not representative of the target population, the resulting AI models can inherit and perpetuate those biases. This can lead to biased outcomes and discriminatory results.
3. Lack of Consistency
Inaccurate labeling methods can lead to inconsistent annotations across different labelers or labeling iterations. Inconsistency in data labeling can undermine the reliability of the training data and make it difficult to build robust and consistent AI models.
4. Increased Iteration Time and Costs
Inaccurate labeling methods may require additional iterations to correct errors and inconsistencies. This can significantly increase the time and costs associated with data annotation, as labelers and reviewers need to go back and forth to resolve labeling issues, resulting in delays in model development and deployment.
5. Reduced Model Performance
Inaccurate data labeling can have a direct impact on the performance of AI models. If the labeled data is incorrect or inconsistent, the models trained on such data may exhibit poor accuracy, low precision, or high error rates. This can diminish the overall value and usability of the AI solution developed by the startup.
6. Reputation and Trust Concerns
Inaccurate data labeling practices can damage the reputation and trustworthiness of small AI-based startups. If users or clients discover inaccuracies or biases in the AI models, it can erode confidence in the startup's offerings and hinder their business growth.
So, addressing these challenges requires startups to prioritize and invest in accurate data labeling methods. This can involve implementing clear annotation guidelines, conducting quality control checks, engaging domain experts, providing proper training to labelers, and establishing feedback loops for continuous improvement. Startups need to recognize the significance of accurate data labeling as a foundation for building reliable and ethical AI solutions.
How using Labellerr can help you track the progress of your project?
Let’s understand with an example:
Scenario: A computer vision technology startup, has just begun a large-scale data annotation project. To achieve the stringent standards, they chose Labellerr, a well-known provider of data annotation services.
However, they are now looking for efficient ways to track the project's development and assure its completion on schedule. Fortunately, Labellerr has several options that can help this startup and accelerate the project's progress.
1. User Activity Tracking
Labellerr's user activity tracking feature enables startup's internal team to monitor the annotators' involvement and productivity. The platform gives precise information on each annotator's time spent on the project, the number of annotations performed, and any communication or comments shared. Startup's internal team can uncover bottlenecks or possible concerns in the annotating process by monitoring user behavior.
2. User-Level Velocity Tracking
Labellerr's user-level velocity tracking tool allows startup's internal team to measure the speed and efficiency of individual annotators. It displays the average time it takes each annotator to complete annotations, the number of annotations completed every hour, and other important data. This enables startup's internal team to identify annotators who are excelling or who want further help or direction.
3. Labeling Trends
Labellerr's labeling trends function offers startup's internal team a detailed picture of the annotation progress over time. It depicts the rate at which annotations are finished, emphasizing any variations or trends. This assists startup's internal team in identifying moments of high activity or possible slowdowns, allowing them to commit more resources or change timetables as needed.
4. Label Distribution
Using Labellerr's label distribution capability, startup's internal team can learn about the distribution of annotated labels within a dataset. It offers a breakdown of the frequency and distribution of various labels or categories, providing useful information on the annotation process's progress and balance. This feature allows startup's internal team to verify that annotations are appropriately distributed over the dataset and that no labels are over-represented or ignored.
Now, startup's internal team can efficiently follow the progress of the data annotation project outsourced to Labellerr by exploiting these functionalities. They can track user behavior, identify top annotators, analyze labeling patterns, and assure correct label distribution. This not only keeps them up to date on the project's progress but also gives actionable insights to optimize productivity and hasten completion.
Have low-quality images and want to Improve the quality and attribute of data?
Here’s this feature that can help you-
Labellerr's Image Enhancement feature and Attribute Changing feature for Object Detection can help improve the quality and attributes of low-quality images in different ways.
1. Image Enhancement Feature:
Labellerr's Image Enhancement feature can help improve the quality and visual appearance of low-quality images. It typically includes tools and techniques to enhance image sharpness, adjust brightness and contrast, reduce noise, and improve overall clarity. By using this feature, users can enhance the visual quality of their low-quality images, making them more visually appealing and easier to analyze.
For example, if a user has low-resolution images that appear blurry or pixelated, the Image Enhancement feature can help sharpen the images, enhance their details, and reduce artifacts. This can be beneficial when analyzing or sharing the images for various purposes, such as object recognition, visual inspection, or training machine learning models.
2. Attribute Changing Feature for Object Detection:
Labellerr's Attribute Changing feature for Object Detection allows users to modify or update the attributes associated with objects in an image. Object detection involves identifying and classifying objects within an image and assigning attributes to those objects. However, in certain cases, the attributes assigned to objects might be incorrect, incomplete, or need modification.
With this feature, users can change or update attributes such as object labels, bounding box positions, sizes, or any other relevant attributes associated with objects in the image.
It can be particularly useful when the initial object detection results are inaccurate or when the user wants to refine the object annotations to improve the quality and accuracy of the dataset.
For example, if a user has low-quality images with objects that were not correctly labeled during the initial annotation process, the Attribute Changing feature allows them to rectify the labels and ensure accurate object detection. This improves the reliability and usefulness of the annotated dataset for downstream tasks like training object detection models.
In summary, Labellerr's Image Enhancement feature helps improve the visual quality of low-quality images, making them clearer and more visually appealing. The Attribute Changing feature for Object Detection allows users to modify or update attributes associated with objects in an image, enabling them to rectify any inaccuracies or refine the annotations for improved object detection accuracy.
Streamline Annotation Process with Labellerr's "Copy Previous" Feature
Labellerr's "Copy Previous" feature can be immensely helpful when a user has a large amount of data and needs to annotate similar types of data repeatedly. This feature allows users to quickly copy annotations from previously annotated images to new images, reducing the annotation time and effort required for similar data.
“This feature is particularly useful for tasks where the same object appears in multiple images, such as object detection or segmentation. By copying the annotations from the previous file, users can quickly annotate the same object in multiple images without having to repeat the annotation process for each image.”
Here's how Labellerr's "Copy Previous" feature can assist in this scenario:
- Annotation Consistency: When annotating a large dataset, maintaining consistency across annotations is crucial. The "Copy Previous" feature ensures that annotations for similar images are consistent, as it copies annotations from previously annotated images that share similar characteristics or attributes. This consistency is vital for training accurate machine learning models and conducting meaningful data analysis.
- Time and Effort Savings: Manually annotating each image individually can be time-consuming and labor-intensive, especially when dealing with a vast amount of similar data. With the "Copy Previous" feature, users can save time and effort by copying annotations from one image to another that requires the same or similar annotations. This significantly speeds up the annotation process, allowing users to annotate large datasets more efficiently.
- Quality Assurance: Copying annotations from previous images helps maintain annotation quality. If a user has already carefully annotated similar images, they can be confident that the copied annotations are accurate and reliable. This reduces the chances of errors or inconsistencies that may arise from annotating each image from scratch.
- Flexibility and Customization: Labellerr's "Copy Previous" feature often offers flexibility and customization options. Users can choose to copy all annotations or specific annotation types, depending on their requirements. This allows users to adapt the copied annotations to fit the specific characteristics of each new image while still benefiting from the initial annotations.
Overall, Labellerr's "Copy Previous" feature streamlines the annotation process for large datasets with similar data. It ensures annotation consistency, saves time and effort, maintains annotation quality, and offers flexibility for customization. By leveraging this feature, users can annotate their data more efficiently and effectively, accelerating their data annotation workflows.
Search Question Feature
The Search Question feature in Labellerr’s platform is extremely helpful in vast and complex datasets. It allows you to search for specific instances or patterns within the data, making it easier to find and annotate similar data points efficiently.
For example, if you are annotating images for object detection and want to see all instances of a particular object or category, the Search Question feature will save time and effort by automatically locating those specific instances.
Benefits:
- Time-saving: Instead of manually searching through the entire dataset, you can quickly find and annotate specific examples.
- Consistency: It helps ensure consistent annotation across similar instances by allowing you to review and apply annotations in a focused manner.
- Error reduction: By pinpointing specific data points, you can reduce the chances of missing relevant instances during annotation.
Auto-Label Feature
The Auto-Label feature is a powerful tool that uses pre-trained models, machine learning, or other AI algorithms to automatically suggest annotations for the data. This feature can greatly speed up the annotation process and improve overall efficiency.
Benefits:
- Faster annotation: AI-driven suggestions can significantly reduce the manual effort required for annotation, particularly for large datasets.
- Semi-automation: While the suggestions may not always be 100% accurate, they can serve as a starting point for human annotators to review and refine, speeding up the overall process.
- Improved consistency: The auto-labeling feature can help maintain consistency in annotations across the dataset by suggesting similar labels for similar instances.
However, it's essential to exercise caution with the auto-labeling feature, as it may not always produce accurate results, especially in complex or ambiguous data. Human review and validation are crucial to ensure high-quality annotations.
Client Review Feature
Labellerr's client review feature allows clients and stakeholders to actively participate in the data annotation process. Clients can access the annotated data directly through the platform, view the annotations made by the annotators, and provide feedback or comments on specific data instances.
This enables effective collaboration between the data annotation team and clients, ensuring that the annotations meet the required quality standards and project specifications.
Through this feature, clients can verify that the annotations align with their needs, and any discrepancies or improvements can be addressed promptly. This iterative feedback loop helps refine the annotation process, leading to more accurate and reliable labeled data, which is crucial for training robust machine learning models.
File Filters
Labellerr's file filters enhance the user experience by providing an intuitive and efficient way to organize and manage annotated data. Users can apply various filters to sort files based on their status, labels, or other attributes. For example, users can filter files that are ready for review or files that have been rejected during the annotation process.
By utilizing file filters, data annotation teams can prioritize their work, focus on specific subsets of data, and identify any problematic files that need attention. This feature ensures that users can work with large datasets effectively and efficiently, streamlining the overall annotation process.
Activity Status
The activity status feature in Labellerr offers real-time insights into the progress of the data annotation project. Project managers and team members can monitor key metrics, such as the number of files annotated, pending files, and reviewed files. This information allows them to assess the project's overall status, identify any potential bottlenecks, and allocate resources effectively.
By tracking activity status, data annotation teams can ensure that deadlines are met, and projects stay on schedule. This feature empowers project managers to make data-driven decisions, leading to improved productivity and better project management.
Export Support
Labellerr's export support feature provides users with the flexibility to export annotated data in various formats. Whether it's CSV, JSON, XML, or other specialized formats, users can seamlessly integrate the annotated data into their preferred downstream workflows and systems.
This export capability is essential for researchers, developers, or data scientists who need to use the annotated data for training machine learning models or conducting data analysis. The platform's export support ensures compatibility with different tools and platforms, simplifying the transition from data annotation to model training or analysis.
Explore our platform and fasten your data annotation process!

Data Annotation Workflow Plan
Simplify Your Data Annotation Workflow With Proven Strategies
Download the Free Guide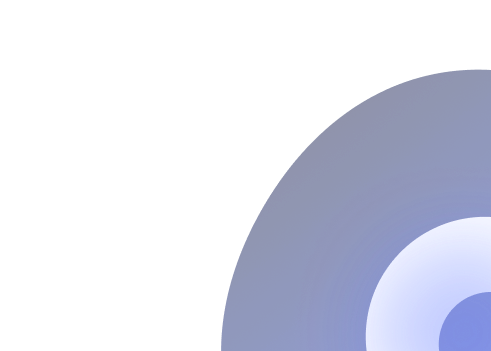