Scale AI vs Labellerr vs Appen
Compare Scale AI, Labellerr, and Appen for data annotation: Scale AI excels at complex, large-scale projects, Labellerr offers balanced automation and affordability, and Appen provides a human-powered approach for varied project sizes with global reach.
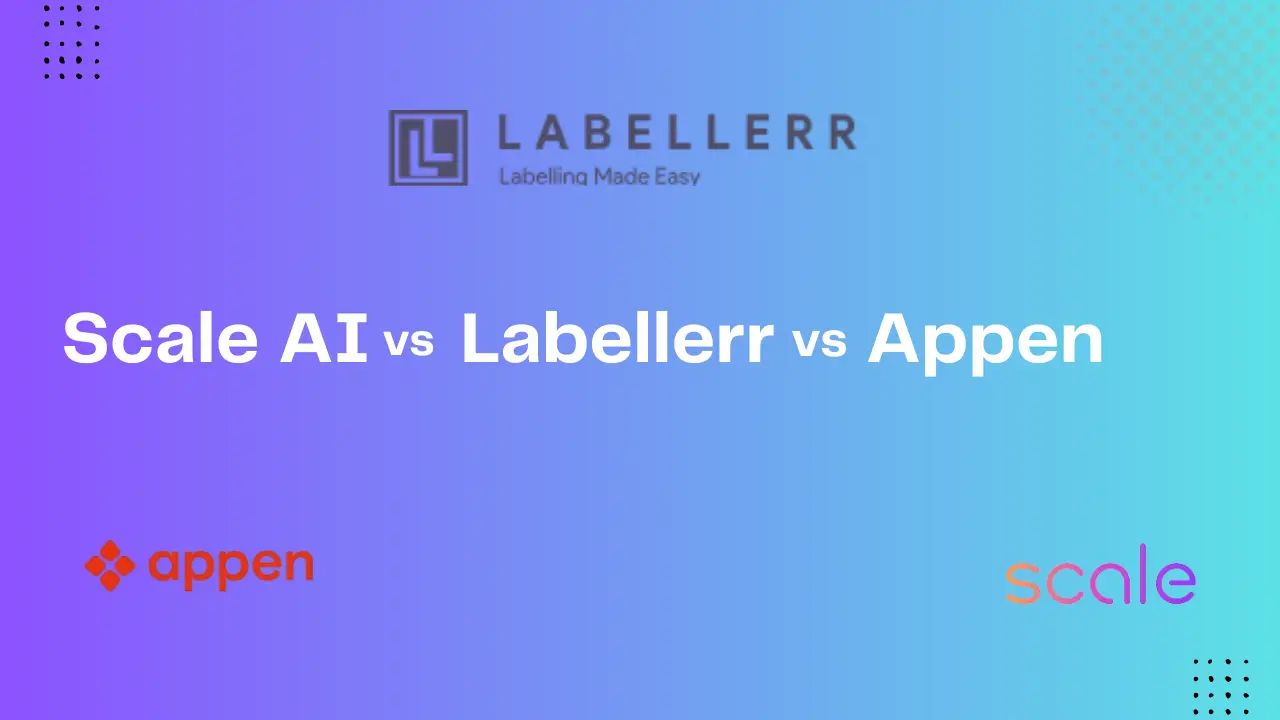
Table of Contents
Introduction
In the dynamic realm of artificial intelligence (AI) and machine learning (ML), the efficacy of models hinges significantly on the quality and precision of the annotated data used for training.
Annotation platforms have thus emerged as critical tools, enabling the labeling of vast datasets required to develop and fine-tune these intelligent systems.
Among the options available, Scale AI, Labellerr, and Appen stand out as prominent players, each offering distinct capabilities and features designed to cater to the diverse needs of AI practitioners and organizations.
These platforms not only streamline the annotation process but also ensure the delivery of high-quality labeled data that can enhance the performance of AI models across various applications.
By comparing these platforms, we can gain deeper insights into how they address the challenges of data annotation, and identify the optimal solutions for different AI development scenarios.
Scale AI vs Labellerr
1. Project Size and Complexity
Scale AI: Specializes in handling large-scale, intricate projects demanding high-quality data. They excel at complex tasks like image segmentation, natural language processing (NLP), and object detection in autonomous vehicles.
Labellerr: Targets medium to large projects, offering a balance between self-service tools and managed services. They provide solutions for various annotation tasks including image and video classification, bounding boxes, and segmentation.
2. Focus and Expertise
Scale AI: Primarily caters to enterprise and government clients, with solutions tailored to specific industries like healthcare, finance, and manufacturing.
Labellerr: Targets a broader range of clients, offering general data annotation services across various industries. Their focus lies in providing efficient and cost-effective solutions.
3. Technology and Automation
Scale AI: Run manual annotation at a big scale with some automation and leverages machine learning (ML) tools to streamline the annotation process. This approach can significantly reduce project turnaround times for large datasets.
Labellerr: Offers a strong balance of human-in-the-loop and automated annotation tools. Their platform includes features like auto-labeling and active learning to accelerate workflows.
4. Pricing and Cost Structure
Scale AI: Provides custom pricing based on project specifics. Given their focus on complex tasks and automation, their costs may be higher than Labellerr's for simpler projects.
Labellerr: Generally offers more transparent pricing with tiered options based on data volume and complexity. This can be more budget-friendly for medium-sized projects. Also, support sporadic and irregular data inflow without extra onboarding charges.
5. Data Security and Compliance
Scale AI: Maintains high data security standards with robust compliance certifications relevant to specific industries, ensuring client confidentiality.
Labellerr: Provides strong data security protocols, but they may not cater to the same range of industry-specific compliance requirements as Scale AI. They also comply with HIPAA and GDPR regulations.
6. User Interface and Workflow
Scale AI: Offers a user-friendly platform designed for managing large datasets and complex workflows efficiently.
Labellerr: Provides a simple and intuitive interface, making it easy to learn and use. This can be beneficial for teams with limited technical expertise.
7. Customer Support
Scale AI: Offers dedicated customer support teams catering to enterprise clients' specific needs.
Labellerr: Provides responsive customer support, with a focus on clear communication and user education. However, the level of customization may be less extensive compared to scale AI.
Scale AI vs Appen
1. Project Size and Complexity:
Scale AI: Specializes in handling large-scale, intricate projects requiring high-quality data. Their platform caters to complex tasks like image segmentation, natural language processing (NLP), and object detection in autonomous vehicles.
Appen: Handles projects of various sizes, offering flexibility for both large and smaller datasets. Their platform excels at common tasks like image and text classification, sentiment analysis, and content moderation.
2. Focus and Expertise:
Scale AI: Focuses on enterprise and government clients, providing solutions tailored to specific industries like healthcare, finance, and manufacturing.
Appen: Caters to a broader clientele, offering general-purpose data annotation services across various industries.
3. Technology and Automation:
Scale AI: Prioritizes automation and utilizes machine learning (ML) tools to streamline the annotation process. This can significantly reduce project turnaround times.
Appen: Offers a mix of human-in-the-loop and automated annotation tools. While they're incorporating more automation, their core strength lies in a large, global workforce.
4. Pricing and Cost Structure:
Scale AI: Offers custom pricing based on project specifics. Given their focus on complex tasks, their costs may be higher than Appen's for simpler projects.
Appen: Provides a more transparent pricing structure with tiered options based on project complexity and data volume. This can be more cost-effective for smaller-scale projects.
5. Data Security and Compliance:
Scale AI: Prioritizes high data security standards to ensure client confidentiality. They offer robust compliance certifications relevant to specific industries.
Appen: Maintains strict data security protocols but may not cater to as wide a range of industry-specific compliance requirements as Scale AI.
6. User Interface and Workflow
Scale AI: Offers a user-friendly platform designed for managing large datasets and complex workflows efficiently.
Appen: Provides a well-established platform with a focus on usability. However, their interface may not be as feature-rich for managing intricate projects compared to Scale AI.
7. Customer Support
Scale AI: Offers dedicated customer support teams catering to enterprise clients' specific needs.
Appen: Provides responsive customer support, but the level of customization may vary depending on your project size.
Labellerr vs Appen
1. Focus and Expertise
Labellerr: Targets a broad range of clients, offering general data annotation services across various industries. Their strength lies in user-friendly tools and cost-effective solutions.
Appen: Caters to a wider clientele, including both enterprise and individual clients. They offer a mix of general and specialized services, including content moderation and search engine optimization (SEO) tasks.
2. Technology and Automation
Labellerr: Offers a blend of human-in-the-loop and automated annotation tools. Their platform prioritizes user experience and includes features like auto-labeling and active learning to enhance efficiency.
Appen: Employs a large, global workforce for human-powered annotation. While they're incorporating some automation tools, their core strength lies in managing large-scale human labeling projects.
3. Pricing and Cost Structure
Labellerr: Often provides more transparent pricing with tiered options based on data volume and complexity. This can be cost-effective for smaller projects or startups.
Appen: Offers tiered pricing based on project complexity and data volume, but specifics may be less transparent compared to Labellerr. Their larger workforce can potentially lead to higher costs for simpler tasks.
4. Data Security and Compliance
Labellerr: Provides strong data security protocols, but they may not cater to the same range of industry-specific compliance requirements as some larger platforms.
Appen: Maintains strict data security protocols and offers compliance certifications relevant to specific industries.
5. User Interface and Workflow
Labellerr: Provides a simple and intuitive interface, making it easy to learn and use. This can be beneficial for teams with limited technical expertise.
Appen: Offers a well-established platform designed for managing large workforces. While user-friendly, it may not be as streamlined for individual users compared to Labellerr.
6. Project Size and Complexity
Labellerr: Targets medium to large projects, offering a balance between self-service tools and managed services. They provide solutions for various annotation tasks.
Appen: Handles projects of various sizes, offering flexibility for both large and smaller datasets. They excel at common tasks but may require more oversight for complex projects.
7. Customer Support
Labellerr: Provides responsive customer support, with a focus on clear communication and user education. However, the level of customization may be less extensive compared to larger platforms.
Appen: Offers responsive customer support, but the level of customization may vary depending on your project size and specific needs.
Conclusion
The ideal data annotation platform hinges on your specific project requirements and budget. Here's a quick recap:
For large-scale, LLM RLHF and SFT requirement Scale AI might be the best fit, However, they might not be catering to young startups and medium-scale businesses with limited budgets.
For medium to large-scale image, and video annotation projects seeking a balance between cost, user-friendliness, and automation Labellerr could be a better choice.
Appen might be a strong contender for projects of various sizes with a focus on human-powered annotation and a global workforce.
Ultimately, the best approach is to carefully evaluate your project needs, test out platform demos, and consider factors like pricing, turnaround times, and the level of customer support offered.
By carefully considering these factors, you can select the data annotation platform that propels your AI project towards success.
FAQ
1. What factors should I consider when choosing a data annotation platform?
Project size and complexity: Consider the volume and type of data you need labeled, as well as the level of automation or human oversight required.
Budget: Pricing structures vary across platforms. Factor in data volume, complexity, and desired turnaround times when comparing costs.
User interface and ease of use: Evaluate the platform's usability, especially if your team has limited technical expertise.
Data security and compliance: Ensure the platform prioritizes data security and offers necessary compliance certifications for your industry.
Customer support: Consider the level of support offered, particularly if you anticipate needing ongoing assistance.
2. What are the benefits of using a data annotation platform?
Data annotation platforms offer several advantages:
Improved data quality: These platforms provide tools and methodologies to ensure consistent and accurate labeling.
Increased efficiency: Platforms can automate repetitive tasks and streamline workflows, saving time and resources.
Scalability: They can handle large datasets and growing annotation needs as your project evolves.
Access to a global workforce: Some platforms offer access to a large pool of qualified labelers worldwide.
Collaboration features: Platforms can facilitate communication and collaboration between team members working on annotation tasks.
3. What are some best practices for data annotation?
Clear and concise labeling guidelines: Define clear instructions for labelers to ensure consistency and minimize errors.
Double-checking and quality control: Implement quality control measures to verify the accuracy of labeled data.
Active learning: Utilize tools that prioritize labeling the most impactful data points for efficient model training.
Ongoing monitoring and improvement: Continuously monitor the annotation process and make adjustments as needed.

Simplify Your Data Annotation Workflow With Proven Strategies
.png)
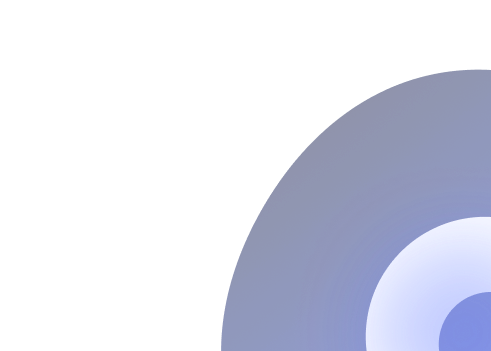