Accelerate Vision AI Development: Solar Farm Monitoring with LabelGPT's Zero-Shot Annotation
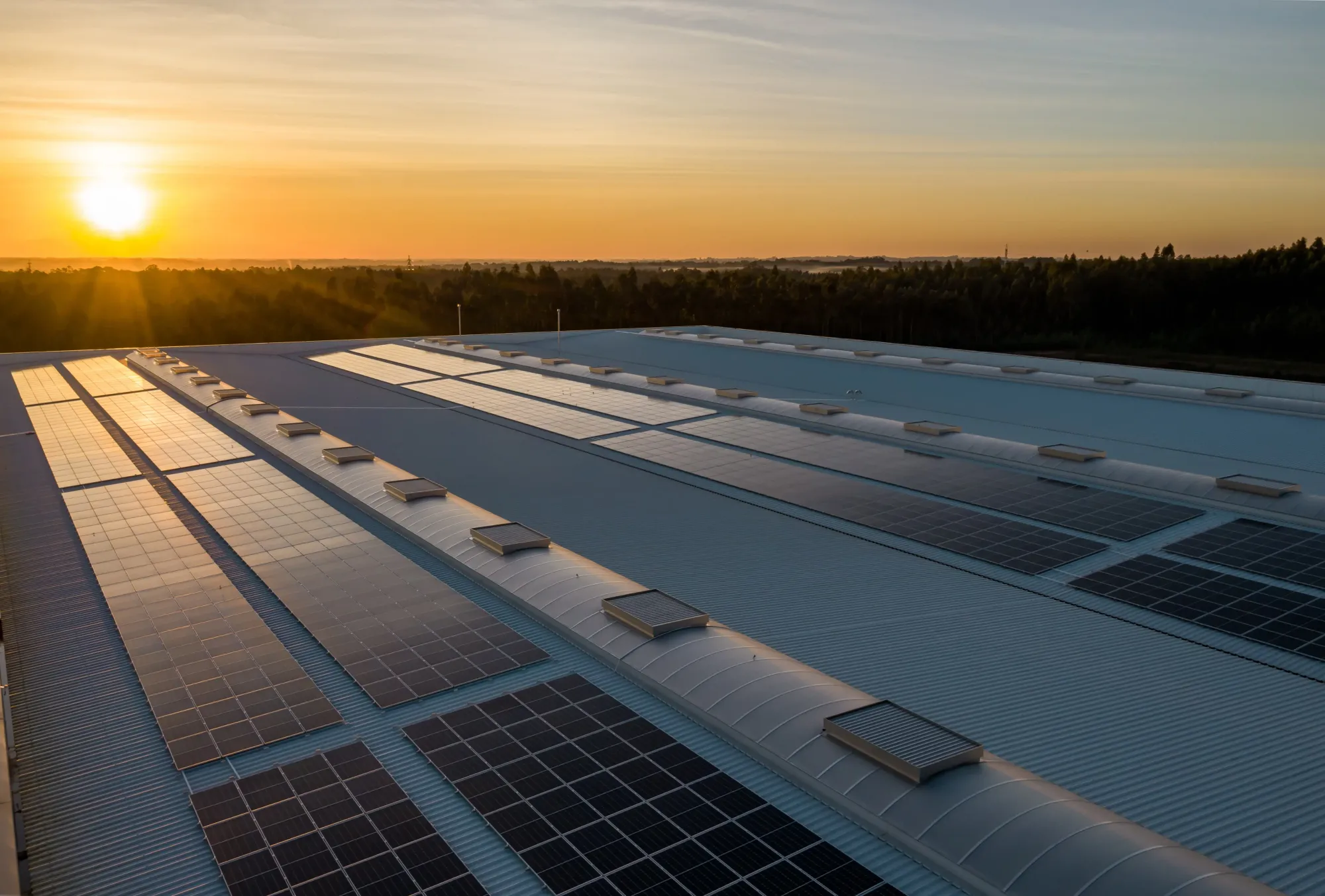
Solar energy has emerged as a powerful and sustainable solution to our energy needs. With the proliferation of solar farms across the globe, monitoring these expansive installations has become a critical endeavor.
Solar farm owners and operators face a myriad of challenges, from identifying electrical and physical damage to monitoring environmental factors like shadows, sun glare, dust, hot spots, and snow. Traditional monitoring methods can be time-consuming and inefficient.
Enter the era of cutting-edge computer vision technology with LabelGPT.
Before we dive into the exciting world of computer vision and its applications in solar farm monitoring, let's kick things off with a little geeky humor:
Why did the 'computer vision system' apply for a job at the 'solar farm'? Because it wanted to "brighten" up its career and "shine" a light on solar power efficiency! 🌞🔍💡
In this blog, we will explore the revolutionary potential of LabelGPT to perform zero-shot annotation in the realm of solar farm monitoring. We will delve into the significance of computer vision use cases, discussing how our software can accurately annotate images and revolutionize the way we manage and optimize solar energy production.
What is Solar Farm Monitoring?
Before we dive into the exciting world of computer vision and its applications in solar farm monitoring, let's understand the fundamentals. Solar farm monitoring involves the continuous assessment and surveillance of solar installations, ensuring their efficiency, safety, and longevity. This process traditionally relies on manual inspection, which can be labor-intensive, time-consuming, and subject to human error. Computer vision technology, however, introduces a game-changing approach.
The problem statement and their corresponding labels in which LabelGPT's zero-shot annotation results high accuracy are -
1. Physical Damage Detection
2. Electrical Damage Detection
3. Sun Detection
4. Shadow Detection
5. Snow Detection
6. Bird Poop Detection
Let's discuss this problem statement in detail here and how zero-shot labeling performance of LabelGPT can help accelerate vision AI development -
1. Physical Damage Detection
Physical damage to solar panels can significantly reduce their efficiency. With zero-shot annotation, our software can pinpoint cracks, chips, or breakages on panels with an accuracy rate of 85%. This enables timely maintenance and repair, safeguarding the long-term performance of solar assets.
Moreover, to bolster the credibility of our software's performance, we conducted comprehensive testing on a tailored dataset of solar panels. Through these tests, we established that for every 20 instances of physically damage solar panels, our system successfully detected 17, resulting in an average accuracy rate of 85%.
This rigorous assessment underscores the robustness and effectiveness of LabelGPT in addressing physical damage concerns within solar farm operations.
Figure: Zero-shot Physical Damage Detection
2. Electrical Damage Detection
One of the foremost challenges in solar farm management is identifying electrical damage. Our software LabelGPT, powered by advanced computer vision algorithms boasts an impressive accuracy rate of 75% in detecting anomalies such as damaged cables, junction boxes, or connectors.
It does so with exceptional accuracy, minimizing downtime and maximizing energy production.
Furthermore, to validate the robustness of our software, we conducted extensive testing on a custom dataset of solar panels. In this testing, we discovered that for every 20 electrically damaged solar panels, we were able to correctly detect 15, yielding us an average accuracy of 75%.
This rigorous evaluation underscores the reliability and effectiveness of LabelGPT in addressing electrical damage concerns in solar farm operations.
Figure: Zero-shot Electrical Damage Detection
3. Sun Detection
In the quest to maximize solar energy production, harnessing the power of the sun is paramount. With a remarkable accuracy rate of 95%, LabelGPT excels at identifying the presence of sunlight.
This capability allows for the precise mapping of areas where sunlight falls, ensuring that solar panels are positioned to capture this positive energy source optimally.
By detecting the sun with exceptional accuracy, our software enables solar farms to align panels precisely, ensuring they are angled to receive maximum sunlight exposure throughout the day.
This dynamic adjustment optimizes energy generation and enhances the overall efficiency of the solar array.
Figure: Zero-shot Sun's Position Detection
4. Shadow Detection
Shadows can cast a cloud over solar panel performance, leading to sub-optimal energy production. LabelGPT's prowess extends to shadow detection, boasting an accuracy rate of 85%. By identifying areas under shadow, our software facilitates the automated adjustment of solar panel positioning.
When labelgpt detects shadows falling on specific panels, it triggers real-time adjustments, such as altering the angle of these panels or redirecting nearby objects causing the shadow.
This proactive response ensures that shadows have a minimal negative impact on energy production, ultimately leading to higher overall efficiency and energy yield.
In essence, LabelGPT's dual capability to detect both sunlight and shadows with impressive accuracy plays a pivotal role in optimizing solar panel layouts.
By automatically adjusting the orientation of panels to capture sunlight and mitigate shadow effects, our software maximizes energy production, making solar farms more efficient and sustainable contributors to the clean energy landscape.
Figure: Zero-shot Shadow Detection
5. Snow Detection
In snowy climates, the presence of snow on solar panels can disrupt energy production. With a remarkable accuracy rate of 94%, our computer vision system instantly identifies snow-covered areas. This capability facilitates efficient snow removal, allowing solar farms to swiftly restore functionality and meet energy production targets.
In addition to that we found that for every 20 snow-covered regions, we were able to detect 15, yielding us an average accuracy of 75%. This indicates that our system can effectively identify snow-covered areas and damaged parts on solar panels, even in challenging conditions.
Figure: Zero-shot Region's Snow Covered Region Detection
6. Bird Poop Detection
In the realm of solar farm monitoring, even seemingly minor issues matter. Bird droppings, for example, can significantly impact solar panel efficiency. When left unchecked, they accumulate, obstructing sunlight and reducing energy production.
In areas with abundant bird populations, this problem can be especially challenging.
Manual inspections for bird droppings are time-consuming and may lead to delayed detection and decreased panel performance. With an impressive 85% accuracy rate, LabelGPT can swiftly identify bird droppings on solar panels.
This feature empowers solar farm operators to take immediate action, ensuring clean and unobstructed panels, ultimately leading to optimal energy generation.
By incorporating bird poop detection into LabelGPT's repertoire, solar farms can further boost their efficiency and productivity, contributing to the success of renewable energy initiatives.
Figure: Zero-shot Bird Poop Detection
The Accuracy of Zero-Shot Annotation
The heart of our software lies in its remarkable accuracy. Thanks to sophisticated deep learning models, our solution can learn and adapt to different solar farm environments, making it capable of zero-shot annotation on virtually any image. Our software's precision ensures that almost no anomaly or issue goes unnoticed, enhancing the overall efficiency and longevity of solar installations.
Best Practices
When it comes to harnessing the full potential of LabelGPT for this particular use case of solar farm monitoring, there are several best practices that can significantly enhance the accuracy and efficiency of the annotation process. While LabelGPT's zero-shot annotation capabilities are versatile and powerful, we have found that achieving higher accuracy often involves performing zero-shot annotation label by label. Here is what I mean by it.
This means first focusing on one specific label, such as electrical damage, and annotating images exclusively for that label. Then, proceed to the next label, such as physical damage, and so on. Rather than to use LabelGPT for zero-shot annotation on the entire dataset with all the labels combined.
In conclusion, the choice between these two approaches depends on your monitoring objectives. If you require a quick and broad assessment of solar farm conditions, using zero-shot annotation on the entire dataset can provide valuable insights. However, for meticulous and precise analysis of specific issues like electrical or physical damage, adopting a label-by-label zero-shot annotation approach is recommended. Ultimately, the flexibility of LabelGPT allows you to tailor your annotation strategy to best suit your solar farm monitoring needs, ensuring the accuracy and efficiency of your assessments.
Conclusion
Solar farm monitoring has reached a new frontier with zero-shot annotation powered by computer vision technology. The applications are vast, from identifying electrical and physical damage to managing shadows, dust, hot spots, and snow. With LabelGPT, the solar energy industry can boost production, reduce downtime, and ensure the sustainability of these essential installations.
The future of solar farm monitoring has arrived. Embrace the power of computer vision, and join us in revolutionizing the world of renewable energy. By combining the precision of science with the accessibility of user-friendly technology, we are on the brink of a greener, more sustainable energy future.
Resources and References
These resources offer a deeper understanding of the technologies and methodologies used in solar farm monitoring, empowering you to contribute to the advancement of renewable energy and sustainability.
i. Dataset Link : Click Here
ii. S. Siddula, C. Dennis Gleeson and P. Geetha kumari, "Solar Panel Position Control and Monitoring System For Maximum Power Generation," 2020 International Conference on Smart Technologies in Computing, Electrical and Electronics (ICSTCEE), Bengaluru, India, 2020, pp. 169-174, https://doi.org/10.1109/ICSTCEE49637.2020.9277293
iii. Fahad Saleh M. Abdallah , M.N. Abdullah, Ismail Musirin, Ahmed M. Elshamy, "Intelligent solar panel monitoring system and shading detection using artificial neural networks", https://doi.org/10.1016/j.egyr.2023.05.163
iv. M. Maheshwaran, G. Bagyalakshmi, R. Valarmathi and V. Gomathi, "Monitoring of Solar cell and Damage Detection Using LabVIEW," 2023 5th International Conference on Smart Systems and Inventive Technology (ICSSIT), Tirunelveli, India, 2023, pp. 233-238, https://doi.org/10.1109/ICSSIT55814.2023.10060881
Book our demo with one of our product specialist
Book a Demo