Regulations and Ethical Considerations in Image Annotation
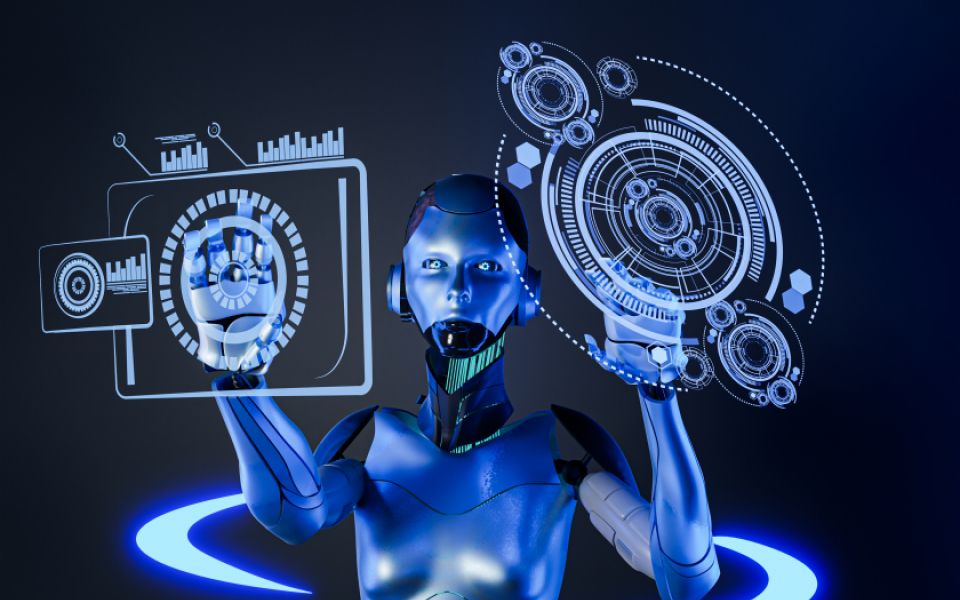
When we talk about annotating data, like images or text, it's important to be fair. This means ensuring the labels accurately show what's in the data and not favor specific people or groups.
For example, if we're labeling pictures of people, we should include a mix of genders, races, and body types. Those doing the labeling need training to avoid any biases they might have.
Using computer programs or existing labeled data can also introduce biases. If the data is biased, the results from the program will be too, causing mistakes.
To fix this, the people labeling the data should be trained to find and fix any biases and include different experiences and perspectives in the labeling process.
Being clear and open is also really important in ethical data annotation. People using the data should know how it was labeled and be aware of any limits or biases. Those doing the labeling should be open about what they're doing, how they're doing it, and if there might be any conflicts of interest.
Table of Contents
- GDPR and Data Privacy in Image Annotation
- Addressing Bias and Fairness in Annotation
- Impact of AI Biases Across Various Sectors
- Ethical Considerations in AI
- Conclusion
GDPR and Data Privacy in Image Annotation
As the demand for data grows, adhering to stricter and often overlapping laws and regulations governing personal data processing becomes crucial.
Harmonizing interpretation and enforcement across the 27 EU states and neighboring regions is essential to facilitate the evolution of AI technology while safeguarding personal data. The European General Data Protection Regulation (GDPR) currently stands as the most stringent data protection regulation, known for its complexity in interpretation.
Figure: Rules of GDPR
Essentially, GDPR mandates using personal information to safeguard individuals' privacy and ensure their full awareness of how their data will be utilized, both presently and in the future. Individuals have the right to control the use of their personal data, including the option to withhold consent for its use.
In summary, GDPR mandates:
- Full control over personal data and transparency about its intended use. At the point of data collection, companies must clearly articulate the purpose of collecting personal data to the individual, allowing them to decide whether to consent to the data collection.
- Limiting the amount of data collected to the minimum necessary to achieve the intended goal for which the data will be collected and processed.
- Informing data subjects about the data controller's identity, how to contact them, the legal basis for processing the data, and the categories of personal data to be processed. Data subjects' rights must also be explicitly communicated in plain language, avoiding technical jargon.
- Documenting how the company meets the requirements of data protection.
Achieving GDPR compliance necessitates expert legal guidance and may involve outsourcing data collection and annotation to GDPR-compliant companies like Sigma, equipped with specialized personnel for handling personal data projects.
Addressing Bias and Fairness in Annotation
The swift progress of machine learning has brought attention to the issues of biases and fairness in AI.
This heightened focus is driven by concerns about the potential harm that biased AI systems might cause. Biased machine learning models can perpetuate existing inequalities, reinforce biases, and adversely impact historically disadvantaged groups.
Figure: Mitigating Bias in the Real World
Even achieving 100% accuracy on a dataset doesn't guarantee foolproof stability or learning for a model. Consequently, there is an increased emphasis on addressing fairness and mitigating bias during the development and deployment of AI systems.
Understanding AI Bias
AI bias occurs when an AI system consistently favors certain groups or individuals over others in its outcomes.
This bias can originate from factors such as biased training data, algorithmic processes, and assumptions made during model development.
Instances of biased AI systems include facial recognition software struggling to identify individuals with darker skin tones or voice recognition systems performing poorly with specific accents.
The Federal Trade Commission's investigation into OpenAI in March 2023, prompted by a Center for Artificial Intelligence and Digital Policy complaint, underscored the crucial need for fair and unbiased AI systems.
Impact of AI Biases Across Various Sectors
AI's impact extends across healthcare, finance, and transportation sectors, but biases can undermine its potential benefits.
In healthcare, AI assists in diagnosing illnesses and predicting treatment outcomes, but biased models may lead to inaccurate diagnoses for certain patient groups. In finance, AI-driven creditworthiness and insurance premiums assessments could unfairly penalize individuals due to biased training data.
Autonomous vehicles, guided by AI, might struggle to navigate diverse pedestrian scenarios because of biased training data. These examples highlight how AI biases can compromise effectiveness and fairness in various contexts.
Addressing Bias in AI: Practical Steps
To tackle biases in AI, developers can adopt various strategies:
- Collecting and Incorporating Diverse Data: Ensuring that the training data encompasses a broad range of individuals and diverse groups.
- Data and Model Audits: Analyzing both training data and model performance to detect and rectify any existing biases.
- Fairness Metrics: Utilizing specialized metrics beyond mere accuracy to assess the fairness of a model.
- Human Oversight: Introducing human judgment into the process to correct biases and uphold ethical outcomes.
- Algorithmic Transparency: Making AI algorithms comprehensible and transparent to facilitate the identification of biases.
- Diverse Development Teams: Forming teams with diverse backgrounds to construct AI systems that are free from bias.
- Continuous Measurement: Regularly evaluating and adjusting AI systems to accommodate evolving biases.
Ethical Considerations in AI
Artificial Intelligence (AI) is widely viewed as a transformative technology, and its societal impacts can be profound.
When incorporating AI and Machine Learning (ML) technologies into your organization, it is crucial to consider their intended functions and address ethical concerns carefully. Planning for ethical considerations ensures that these systems are developed with the collective well-being of humanity in mind.
In the realm of technology, ethics pertains to how humans use technology to achieve goals and how technology systems interact with humanity.
Ethical considerations predominantly involve human-to-human, human-to-machine, and machine-to-human interactions, with occasional relevance to machine-to-machine interactions that involve a human component, impact, or aspect.
Figure: Ethical Considerations in AI Design
Here are ten considerations to take into account when building and using AI systems:
- Fairness and Bias: Ensure that AI technology is fair and unbiased, preventing discrimination based on factors like race, gender, and socioeconomic status. Pay attention to the data the system is trained on.
- Transparency: Be transparent about how AI systems operate, providing users with visibility into system behavior. Clearly communicate how user data is used and protected, addressing disclosure and user consent.
- Privacy: Prioritize privacy as a critical consideration, taking steps to protect user data and prevent misuse or mishandling.
- Safety: Ensure user safety by preventing accidents or harm caused by AI systems. Consider environmental impact and avoid resource use that significantly harms the environment.
- Explainability: Emphasize explainability, ensuring users understand how AI systems make decisions and provide explanations when requested. If using less explainable algorithms, offer means to interpret results.
- Human Oversight: Implement human oversight to ensure AI systems align with human values, laws, regulations, or company policies.
- Trustworthiness: Build trust with users by being transparent about how AI systems work and taking accountability for errors or issues.
- Human-Centered Design: Prioritize human-centered design, considering the needs and wants of users rather than solely focusing on technical capabilities.
- Responsibility: Take responsibility for the actions of AI systems and be accountable for any negative impacts they may have.
- Long-Term Impacts: Consider the long-term effects of AI systems on society and the planet, taking steps to mitigate any negative impacts.
Conclusion
In the realm of data annotation, particularly for images and text, a fundamental principle is fairness.
Accurate representation in labels is crucial, ensuring that the data does not favor specific individuals or groups. For instance, when annotating pictures of people, including a diverse mix of genders, races, and body types is imperative. Adequate training of annotators is essential to minimize biases.
The utilization of computer programs or existing labeled data can introduce biases, potentially leading to errors. To address this, annotators must undergo training to recognize and rectify biases, fostering inclusivity and varied perspectives in the annotation process.
Transparency is a cornerstone of ethical data annotation. Users of the data should be fully informed about how it was labeled, including any limitations or biases. Annotators should uphold openness about their methods, intentions, and potential conflicts of interest.
In the context of GDPR and data privacy, adherence to stringent regulations becomes paramount as the demand for data grows.
Harmonizing interpretation and enforcement across the EU and neighboring regions is vital, especially with the complexity of regulations like GDPR. GDPR mandates full control over personal data, transparency in its use, limiting data collection, informing data subjects, and documenting compliance.
Addressing biases and ensuring fairness in AI annotation is critical due to the swift progress of machine learning. Biased AI systems can perpetuate inequalities and harm historically disadvantaged groups. Understanding AI bias involves recognizing that biases can arise from training data, algorithms, and model development. The impact of biases spans healthcare, finance, and transportation, compromising the potential benefits of AI in these sectors.
Practical steps to address bias involve collecting diverse data, auditing data and models, using fairness metrics, incorporating human oversight, ensuring algorithmic transparency, forming diverse development teams, and continuously measuring and adjusting AI systems.
In the broader context of AI ethics, careful consideration of ten key aspects is crucial. These include fairness and bias, transparency, privacy, safety, explainability, human oversight, trustworthiness, human-centered design, responsibility, and consideration of long-term impacts.
This ethical framework guides the development and use of AI systems, emphasizing the importance of human values, user needs, and societal well-being.
Frequently Asked Questions
1. What tasks and duties does image annotation involve?
Image annotation encompasses assigning labels to an image or a collection of images. A human operator examines a set of images, identifies pertinent objects within each image, and annotates the image by specifying attributes such as the shape and label of each object.
2. What does image annotation entail in image processing?
Image annotation is tagging images to educate AI and machine learning models. This typically involves human annotators utilizing an image annotation tool to label images or attach pertinent information, such as assigning appropriate classes to distinct entities within an image.
Book our demo with one of our product specialist
Book a Demo