Quality Assurance in Data Labeling: Best Practices from Leading Companies
Data labeling companies ensure quality by using AI tools, regular checks, and expert knowledge. They review data carefully, use multiple reviewers for accuracy, and spot mistakes quickly to make sure the labels are correct and useful for training AI models.
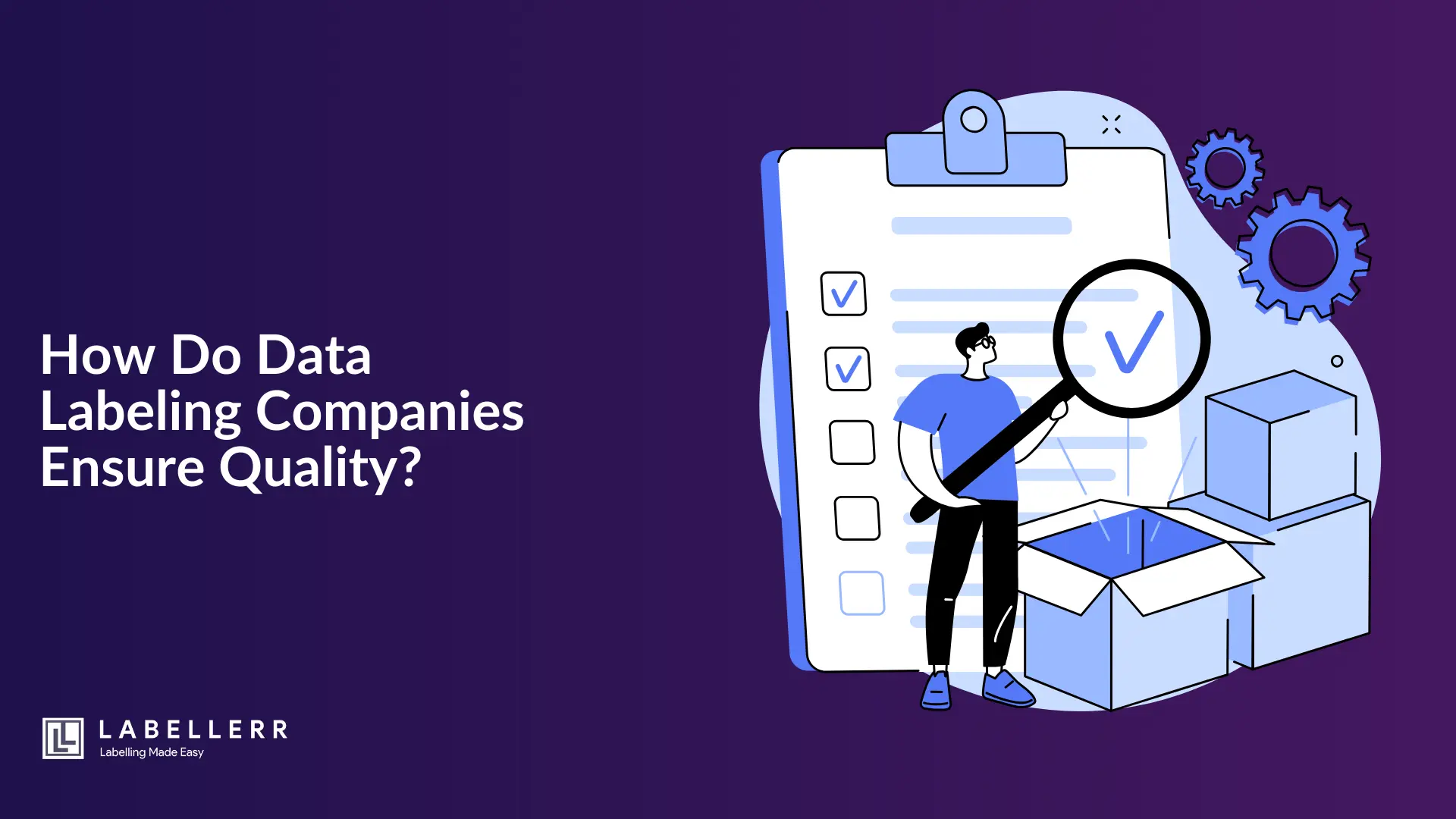
A recent study by Vanson Bourne and Fivetran highlights a critical challenge in AI development: poor data quality.
The survey, conducted across companies with annual revenues ranging from $25 million to over $50 billion, revealed that despite growing investments in AI, low-quality data is devaluing these initiatives.
Ninety-seven percent of respondents plan to invest in generative AI within the next two years.
However, AI models trained on inaccurate or incomplete data led to misinformed decisions, causing an average revenue loss of 6%, equivalent to $406 million for organizations with $5.6 billion in annual revenue.
This emphasizes the importance of high-quality data for AI success. Poor data quality can hinder AI model performance, leading to unreliable predictions and costly mistakes.
Data labeling company play a vital role in overcoming this challenge by ensuring accurate, consistent, and reliable datasets for AI training.
This article explores how these companies maintain quality and why their expertise is essential for effective AI development.
Table of Contents
- Why Quality Matters in Data Labeling?
- Best Quality Assurance Practices
- The Role of Automation in Ensuring Quality
- Choosing a Data Labeling Company with Strong Quality Assurance
- Conclusion
- FAQs
Why Quality Matters in Data Labeling?
High-quality labeled data is the backbone of successful AI models. It ensures that models learn the correct patterns, make accurate predictions, and deliver reliable results.
On the other hand, poor data quality can have a devastating impact on AI projects, leading to financial losses, delays, and reputational damage.
How Labeled Data Impacts AI Performance
Accurate labeling allows AI models to identify patterns, classify objects, and predict outcomes effectively.
For example, a healthcare AI model trained on well-labeled medical images can detect diseases like cancer with high precision. In contrast, models trained on poorly labeled datasets produce unreliable results, which can lead to misdiagnoses or false positives.
Risks of Low-Quality Data
- Financial Costs: According to Gartner, poor data quality costs organizations an average of $12.9 million per year. In some cases, retraining a 530-billion parameter model can cost up to $100 million.
- Misinformed Decisions: A survey found that low-quality data caused AI-driven misinformed business decisions, resulting in an average revenue loss of 6%, equating to $406 million for organizations with $5.6 billion in annual revenue.
- Delayed AI Projects: Between 33% and 35% of AI projects fail or experience delays due to inadequate data quality. These delays slow innovation and increase costs.
- Erosion of Trust: Poor data quality reduces trust in generative AI applications, as users encounter biased or inaccurate outputs.
Data Quality Characteristics
To achieve high-quality labeling, datasets must meet specific criteria:
- Accuracy: Labels must correctly represent the data.
- Completeness: Every relevant detail in the data should be labeled.
- Consistency: Labels must follow uniform standards across the dataset.
- Integrity: The data should remain unaltered during processing.
- Reasonability: Labels should make logical sense within the context of the dataset.
- Timeliness: Data must be up-to-date for relevance.
- Uniqueness/Duplication: Duplicate data entries should be removed.
- Validity: Labels must align with defined rules or guidelines.
- Accessibility: Data should be easy to retrieve and utilize for model training.
Best Quality Assurance Practices
Inter-Annotator Agreement
Inter-annotator agreement ensures consistency when multiple annotators work on the same dataset. This process involves comparing annotations from different individuals to identify discrepancies and resolve conflicts.
For example, if one annotator labels an object as a "car" while another labels it as a "vehicle," the inconsistency is flagged for review. Annotators rely on predefined guidelines to ensure they label data uniformly, but occasional disagreements are inevitable.
These disagreements are resolved through a systematic review process, often involving quality assurance teams or experts. This practice is essential for maintaining uniformity across datasets, which directly impacts the reliability of AI models trained on this data.
Confidence Scoring
Confidence scoring plays a crucial role in identifying uncertain or low-confidence annotations that need further review. Each annotation is assigned a confidence level, which reflects how certain the annotator or tool is about the label.
For instance, a bounding box with low confidence might indicate unclear boundaries, overlapping objects, or poor image quality. Labels with low confidence are flagged for review, allowing teams to revisit and refine them as necessary.
This approach minimizes errors and ensures high-quality annotations. Companies use advanced algorithms to calculate confidence scores, which helps streamline the review process and maintain overall data accuracy.
Anomaly Detection
Anomaly detection helps identify and correct errors or outliers in the dataset. This technique is particularly valuable for maintaining consistency in large datasets where manual checks are impractical.
For example, if most annotations classify a vehicle as a "car," but one instance is labeled as a "truck," the system identifies it as an anomaly. These anomalies can arise due to human error, misinterpretation, or unique edge cases.
By flagging such inconsistencies, anomaly detection ensures the dataset remains uniform and free of biases that could negatively impact the AI model’s performance.
Companies often use machine learning algorithms and quality control tools to automate this process, saving time and resources while ensuring accuracy.
Iterative Feedback Loops
Iterative feedback loops enable continuous improvement in the labeling process by fostering collaboration between annotators, clients, and quality assurance teams.
After each review cycle, feedback is shared with annotators to address recurring issues or refine their techniques. For example, clients may identify areas where labeling guidelines need adjustments to better align with the project’s goals.
This feedback is incorporated into subsequent rounds of labeling, leading to higher accuracy and consistency over time.
Iterative feedback loops are particularly valuable for complex projects where requirements evolve, ensuring that the final dataset meets the desired quality standards.
The Role of Automation in Ensuring Quality
Automation plays a vital role in improving the quality of data labeling while reducing manual errors.
AI-assisted tools, for example, can handle repetitive tasks like drawing bounding boxes or segmenting objects in images, ensuring consistency and saving time.
These tools use techniques such as pre-labeling, where the system generates initial labels based on patterns in the data, and smart predictions, which suggest annotations based on similar labeled examples.
These features speed up the process and significantly reduce the likelihood of errors caused by fatigue or oversight during manual annotation.
Quality checks are another critical aspect of automation. Automated systems flag potential errors, inconsistencies, or low-confidence annotations, prompting human reviewers to verify or correct them.
This collaborative approach between automation and human oversight ensures that the dataset maintains high standards without compromising accuracy.
While automation handles the bulk of the work, human annotators play a key role in refining complex or ambiguous cases. This balance ensures the best of both worlds: efficiency from automation and precision from human expertise.
Scalability and Quality Maintenance
As projects scale to include thousands or even millions of data points, maintaining quality becomes increasingly challenging.
Companies address this by using cloud-based tools that efficiently process large datasets without compromising on speed or accuracy.
These tools enable seamless workflows, allowing teams to manage large volumes of data while adhering to consistent quality standards.
Streamlined workflows play a significant role in maintaining quality during scaling. Teams use predefined guidelines, automated checks, and collaborative tools to ensure annotations remain accurate across the dataset.
For instance, built-in quality assurance mechanisms, such as anomaly detection and inter-annotator agreement, help identify errors in large datasets.
Additionally, real-time updates and feedback loops keep teams aligned and improve consistency as the project grows.
By using automation and scalable tools, companies deliver high-quality datasets, even for the most complex and large-scale AI projects.
These practices ensure that businesses can trust their labeled data to drive accurate and reliable AI model performance.
Choosing a Data Labeling Company with Strong Quality Assurance
When selecting a company, it’s important to focus on their quality assurance practices.
High-quality data is the foundation of any successful AI project, and the right company should have clear processes to ensure consistency and accuracy. Start by asking key questions about their approach to quality:
What are their quality assurance methods?
Look for practices like inter-annotator agreement, confidence scoring, and anomaly detection. These methods help ensure annotations are accurate and reliable.
Do they offer regular quality checks?
Companies that perform ongoing reviews, both automated and manual, are more likely to catch and correct errors early.
How do they handle client feedback?
A good company should have a structured process for incorporating client feedback and making necessary adjustments.
You should also evaluate their ability to maintain consistency across large datasets. Ask for examples of past projects to see how they ensured uniformity in annotations, even with multiple annotators involved.
Look for companies that use standardized guidelines and provide training for their annotators to minimize inconsistencies.
Finally, consider their approach to accuracy. A reliable company will have clear metrics for measuring annotation quality and tools to flag errors or outliers.
By evaluating these factors, you can ensure that the company you choose will deliver high-quality datasets that support your AI goals.
Conclusion
Quality in data labeling is essential for the success of AI projects. High-quality labeled data ensures that AI models perform accurately, make better predictions, and deliver reliable results.
Poor labeling, on the other hand, can lead to errors, wasted resources, and a loss of trust in AI systems.
When selecting a data labeling partner, prioritize companies that emphasize quality assurance practices. Look for a team that ensures consistency, accuracy, and scalability while addressing your specific project needs.
If you're looking for a partner that combines expertise with advanced tools, explore Labellerr's quality-driven data labeling services.
With Labellerr, you can build reliable AI models that drive impactful and efficient solutions. Contact us today to see how we can support your AI development journey.
FAQs
How do companies maintain accuracy in annotations?
Companies use multiple reviewers, cross-validation, and AI tools to check for consistency and accuracy in annotations. They also conduct regular quality checks to ensure high standards.
What role do AI tools play in ensuring the quality of labeled data?
AI tools help by automatically suggesting labels, detecting anomalies, and flagging potential errors. This speeds up the process and improves accuracy, while human reviewers handle more complex decisions.
Why is domain expertise important in data labeling?
Domain experts understand the specific requirements of different industries, which helps in creating accurate and relevant labels. Their knowledge ensures that the labeled data meets the needs of the AI models being trained.

Simplify Your Data Annotation Workflow With Proven Strategies
.png)
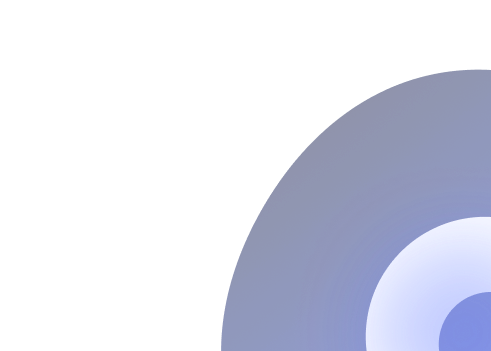