Meta's Llama 3.1- Is It A Gamechanger For Gen AI?
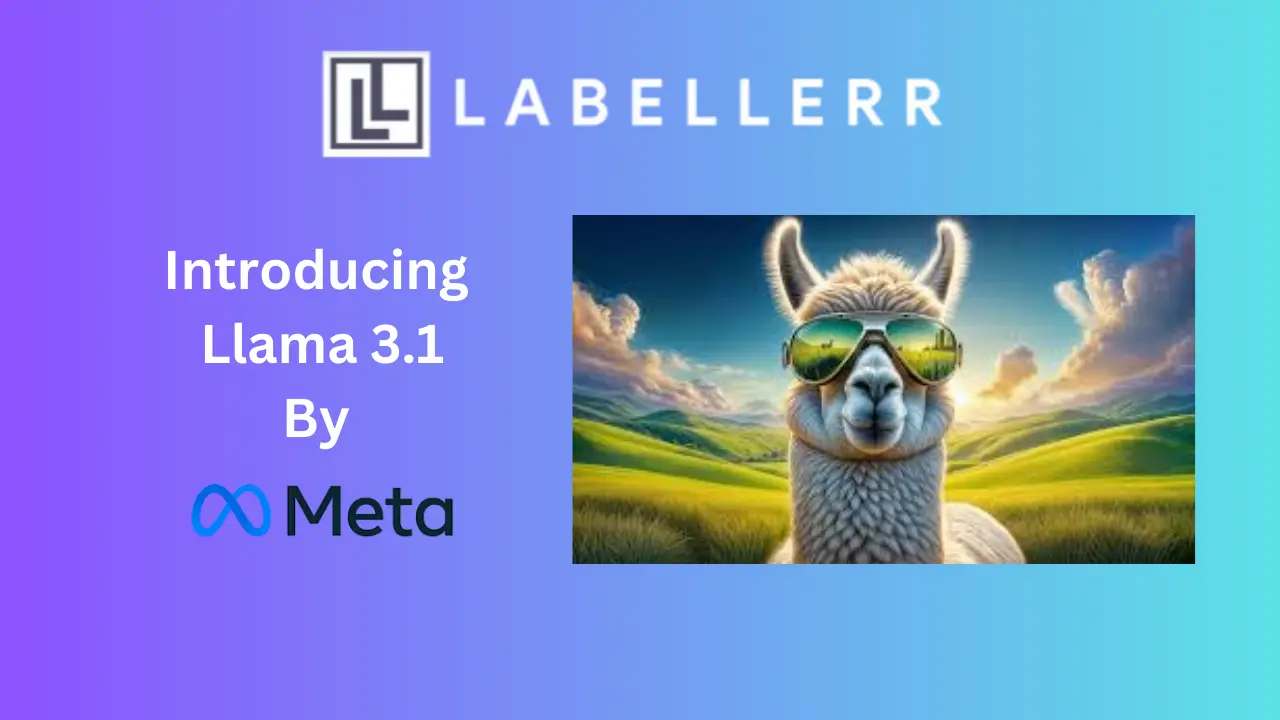
Table of Contents
- Introduction
- Llama 3.1 Model Variants
- Technical Details of Llama 3.1
- Performance Evaluation
- Key Usages of Llama 3.1
- Llama 3.1 Pricing Comparisons
- Llama 3.1 vs Other Models: Industry Use Case Comparison
- Does Llama 3.1 Live Up to the Hype?
- Conclusion
- FAQS
Introduction
2024 is the era of generative AI, where groundbreaking innovations like GPT-4o, Mistral, and Llama have transformed the landscape of artificial intelligence.
Amidst these giants, a new titan has emerged that has taken the AI community by storm: Llama 3.1.
This latest marvel in the Llama series is not just an upgrade; it's a revolution, pushing the boundaries of what we thought would never be possible.
With its staggering advancements in architecture, performance, and versatility, Llama 3.1 is set to redefine the standards of generative AI, leaving everyone—from industry experts to AI enthusiasts—utterly astonished.
Llama 3.1 Model Variants
LLaMA 3.1 brings three powerhouse models to the table, each tailored to different needs within the generative AI space.
Llama 3.1 8B
Let's start with the 8 billion parameter variant. This model is perfect for environments where you need quick, efficient performance without a ton of resources.
The model excels at text summarization, text classification, sentiment analysis, and language translation requiring low-latency inferencing.
Think chatbots and basic content creation. Despite its size, it packs a punch with robust capabilities in natural language understanding and generation.
Llama 3.1 70B
Next up, we have the 70 billion parameter model. It's ideal for more complex tasks like advanced conversational agents and detailed content generation.
This model is well-suited for more complex applications requiring nuanced language understanding, such as advanced conversational bots, detailed content generation, and sophisticated analysis tasks.
It offers a significant boost in accuracy and contextual awareness while maintaining manageable computational requirements.
Llama 3.1 405B
Finally, meet the champion: the 405 billion parameter model. This is the model you call in for the big tasks—handling tasks like large-scale data analysis, high-precision language translation, and intricate content generation.
It's the pinnacle of what LLaMA 3.1 has to offer, delivering unparalleled performance and accuracy for the most demanding AI applications.
But here's the million-dollar question: Which one is right for you? Well, that depends on whether you're trying to power a chatbot or solve world hunger. Choose wisely!
Technical Details of Llama 3.1
Llama 3.1 is not just an incremental upgrade; it represents a significant leap in the field of large language models. Let’s delve into the key features and improvements that make Llama 3.1 stand out.
Architecture
Llama 3.1 follows a standard Transformer architecture to its predecessors(Llama and Llama 2).
- Input Text Tokens: The process begins with text input, which is broken down into individual tokens (substrings with specific meanings).
- Token Embeddings: Each token is converted into a numerical representation (embedding) that captures its semantic and syntactic information.
- Self-Attention Layers: This is where the model shines. Self-attention layers analyze the relationships between different parts of the input sequence, helping the model understand context and dependencies.
- Feedforward Neural Networks: These networks process the information from the self-attention layers, extracting deeper patterns and features.
- Decoder: The decoder takes the processed information and generates the output text, one token at a time.
Decoding Process
The decoding process in Llama 3.1 is autoregressive. This means the model predicts the next token based on the previously generated tokens. It iteratively generates the output sequence until it reaches the end-of-sequence token.
Key Improvements in Llama 3.1
- Grouped Query Attention (GQA): Uses 8 key-value heads to enhance inference speed and reduce the size of key-value caches during decoding.
- Attention Mask: Prevents self-attention between different documents within the same sequence, especially useful for very long sequences.
- Vocabulary Size: Increased to 128K tokens, combining 100K tokens from the tiktoken tokenizer with 28K additional tokens for better support of non-English languages, improving compression rates and performance.
- RoPE Base Frequency: Increased to 500,000 to support longer contexts, allowing effective handling of sequences up to 32,768 tokens.
These enhancements in Llama 3.1 primarily focus on improving data quality, diversity, and training scale rather than changing the fundamental architecture.
Llama 3.1 Training Process
The pre-training process for Llama 3.1 consists of three main stages: Initial pre-training, long-context pre-training, and Annealing.
In the initial pre-training stage, the model is trained using a cosine learning rate and starting with a batch size of 4 million tokens and sequences of 4,096 tokens.
This batch size is doubled to 8 million tokens and sequences of 8,192 tokens after pre-training on 252 million tokens, and again doubled to 16 million tokens after 2.87 trillion tokens.
During this stage, the data mix is adjusted to improve model performance, incorporating more non-English data, mathematical data, and recent web data while downsampling lower-quality data.
In the long-context pre-training stage, the model is trained on long sequences to support context windows of up to 128K tokens.
This is done gradually in six stages, starting from 8K tokens and ending at 128K tokens, using approximately 800 billion training tokens to ensure the model adapts successfully without performance loss on short-context evaluations.
Finally, during the annealing stage, the learning rate is linearly annealed to zero over the final 40 million tokens while maintaining a context length of 128K tokens. High-quality data sources are upsampled, and model checkpoints are averaged (Polyak averaging) to produce the final pre-trained model.
Performance Evaluation
Meta has done thorough testing of Llama 3.1 across variety of standard benchmark datasets.
It has evaluated over 150 diverse benchmark datasets, These benchmarks include a broad range of linguistic activities and abilities, including multilingualism, coding, arithmetic, and general knowledge and thinking.
Benchmark Evaluations
Llama 3.1 405B demonstrates strong performance across a wide range of benchmarks, often leading or closely matching the performance of other models like GPT-4 and Claude 3.5.
Llama 3.1 8B and llama 70B demonstrate exceptional performance across various benchmarks, often surpassing other models, including GPT-3.5 Turbo.
Human Evaluations
Human evaluations of the Llama 3 405B model compared it with GPT-4 (0125 API), GPT-4o, and Claude 3.5 Sonnet, showing that Llama 3 405B performs similarly to the 0125 API version of GPT-4, with mixed results against GPT-4o and Claude 3.5 Sonnet.
It generally matches GPT-4 on most capabilities, with notable strengths in multiturn reasoning and coding, but weaknesses in multilingual tasks.
Llama 3 405B competes well with GPT-4o on English prompts and with Claude 3.5 Sonnet on multilingual prompts, while outshining Claude 3.5 Sonnet in single and multiturn English prompts but lagging in coding and reasoning.
Qualitative assessments highlight that aspects like model tone, response structure, and verbosity significantly impact performance.
Overall, Llama 3 405B is very competitive with leading models and is considered the best-performing openly available model.
Key Usages of Llama 3.1
Llama 3.1 stands out from its predecessors and competitors due to its exceptional capabilities, flexibility, and accessibility. Let's delve into the key areas where it surpasses other LLMs:
Inference Flexibility and Efficiency
Imagine you need quick responses for a chatbot or have tons of data to process. Llama 3.1 has got you covered with its dual-mode inference services.
Llama 3.1 offers both real-time and batch inference services, catering to a wide range of application requirements. This flexibility is crucial for applications demanding immediate responses or those processing large volumes of data in batches.
Users can also download the model weights to their own infrastructure, significantly reducing costs associated with token generation. This level of control over inference is unparalleled in many other LLMs.
Fine-Tune, Distill, and Deploy
Have you ever wanted a model that’s perfectly tuned for your specific tasks?
With Llama 3.1, you can fine-tune it to your own content. Plus, through distillation, you can create smaller, faster versions without losing performance. It’s like having a custom-made sports car!
Whether you prefer on-premises or cloud deployment, Llama 3.1 gives you the flexibility to choose based on your data privacy, security, and operational needs. Talk about versatility!
Groundbreaking RAG and Tool Use
Llama 3.1 isn’t just smart; it’s practically a genius! Thanks to its Retrieval Augmented Generation (RAG) and zero-shot tool use, this model can seek out information, use external tools, and reason over its findings.
Think of it as having a mini-detective at your service, always ready to dig up the right info.
Synthetic Data Generation at Scale
Need more data? No problem! Llama 3.1 can generate high-quality synthetic data from a massive 405 billion token dataset. This can help you build better models, solve data scarcity issues, and speed up your projects. It’s like having a magic wand for data generation!
Other Notable Advantages
Extended Context Window: Llama 3.1's ability to process longer inputs allows for better understanding of complex topics and maintaining context in extended conversations.
Multilingual Support: With support for multiple languages, Llama 3.1 has a broader reach and can be applied to a wider range of global applications.
Llama 3.1 Pricing Comparisons
Image Source: Artificial Analysis
The 3.1 series offers a range of models with competitive pricing, making it a viable option for various applications.
Llama 3.1 8B model stands out as exceptionally cost-effective, with both input and output prices at $0.18, making it suitable for applications where minimizing cost is crucial.
Llama 3.1 170B model provides a balanced option between cost and capability, offering competitive pricing compared to other mid-range models.
On the higher end, the Llama 3.1 405B model, with an input price of $3 and an output price of $5, remains a reasonable choice when compared to more expensive models like GPT-4.0 and Command-R+.
For applications where cost sensitivity is important, the Llama 3.1 8B model is highly recommended due to its low pricing. For more demanding applications that require higher performance, the Llama 3.1 170B offers a good balance of cost and capability.
In scenarios where performance is critical and budget is less of a concern, high-end models like GPT-4.0 may be considered despite their higher costs. Overall, Llama 3.1 models provide cost-effective solutions across different application needs.
Llama 3.1 vs Other Models: Industry Use Case Comparison
Let's talk about how different AI models stack up for businesses of all sizes. Llama 3.1 is making waves, and for good reason.
For startups on a low budget, Llama 3.1 8B is a game-changer. At just $0.18 for both input and output, it's a steal compared to the big guns like GPT-4.0, which can set you back $5 for input and a whopping $15 for output.
Plus, being open-source means you can tinker with it to your content without breaking the bank. Sure, there are middle-ground options like Gemini 1.5 Flash, but Llama flexibility is hard to beat when you're watching every penny.
Moving up to mid-sized companies, Llama 3.1 170B hits a sweet spot. At $0.88 across the board, it's not too shabby for companies looking to dip their toes into serious AI without drowning in costs.
The open-source factor is still a huge plus, letting you mold it to fit your specific needs. Mistral 8x22B is another solid contender at $1.2, but Llama might give you more for your buck. Of course, if you've got deeper pockets and need the cream of the crop, GPT-4.0 is still on the table.
Now, for the big players – the multinational corporations. These guys can really leverage Llama 3.1's flexibility, deploying different-sized models across their global operations.
The 405B version, priced at $3 for input and $5 for output, lets them fine-tune their spending. But let's be real – when it comes to mission-critical stuff, the top-tier models like GPT-4.0, Command-R+, or Reka Core (both at $3 input, $15 output) might be worth the splurge.
They come with all the bells and whistles that big corporations often need.
So, what's the takeaway? Llama 3.1 is shaking things up, especially for smaller players and mid-sized companies looking for a good deal and room to experiment.
For the big corporations, it's a mixed bag – Llama 3.1 offers great flexibility, but sometimes you need the heavyweight champs like GPT-4.0, Gemini 1.5 Pro, or Mistral Large for those high-stakes applications.
In the end, it all boils down to what you need, what you can afford, and where you're headed. There's no one-size-fits-all in the AI world – it's about finding that perfect fit for your unique situation.
Does Llama 3.1 Live Up to the Hype?
So, after all the comparisons and deep dives, does Llama 3.1 really live up to the hype? Well, it depends on what you’re looking for.
On one hand, Llama 3.1 boasts a blend of features that are impressive and useful. Its flexibility with inference, powerful fine-tuning, and innovative RAG and tool use capabilities make it a strong contender in the world of language models. For those who need a model that can be customized and deployed with ease, LLaMA 3.1 certainly delivers.
However, it's important to note that many of the groundbreaking features touted by Llama 3.1 can also be found, more or less, in other leading LLMs.
Whether it's code generation, image generation, or complex contextual understanding, other models like GPT-4, Mistral, and even older versions like Llama 2 offer similar capabilities. In essence, while Llama 3.1 excels, it doesn't necessarily bring something entirely new to the table.
In conclusion, the hype around Llama 3.1 is understandable given its robust features and improvements.
However, if you're looking for a truly revolutionary leap, you might find that other LLMs can achieve similar outcomes. So, while Llama 3.1 is a fantastic tool, whether it's worth the hype depends on your specific needs and how much you value its unique blend of features.
Ultimately, it's a solid choice but not a singular game-changer in the crowded landscape of advanced language models.
Conclusion
Llama 3.1 is not just another update; it's a game-changer in the world of generative AI.
With its advanced architecture, impressive performance enhancements, and broad applicability, it's set to reshape industries like healthcare, customer service, and content creation. This model isn't just about pushing boundaries—it's about redefining them.
Whether you're an industry expert or an AI enthusiast, Llama 3.1 offers a glimpse into the future of AI, where machines understand and generate human language with unprecedented accuracy and efficiency.
So, what are you waiting for? Dive into the world of Llama 3.1 and see for yourself how this revolutionary model is setting new standards in AI
FAQS
Q1) What is Llama 3.1 405B?
Llama 3.1 405B is a large language model developed by Meta AI. It's known for its advanced capabilities in understanding and generating human-quality text, making it a significant leap forward in AI technology.
Q2) What are the key improvements in Llama 3.1 405B over previous models?
Llama 3.1 405B offers significant advancements in areas like reasoning, coding, and multilingual capabilities. It also demonstrates improved performance in handling long-form text and exhibits enhanced general language understanding.
Q3) How does Llama 3.1 405B compare to other large language models?
Llama 3.1 405B is highly competitive with leading models like GPT-4 and Claude 3.5 Sonnet, demonstrating comparable or superior performance in various benchmarks.
Book our demo with one of our product specialist
Book a Demo