7 Best Medical Image Annotation & Labeling Tools In 2024
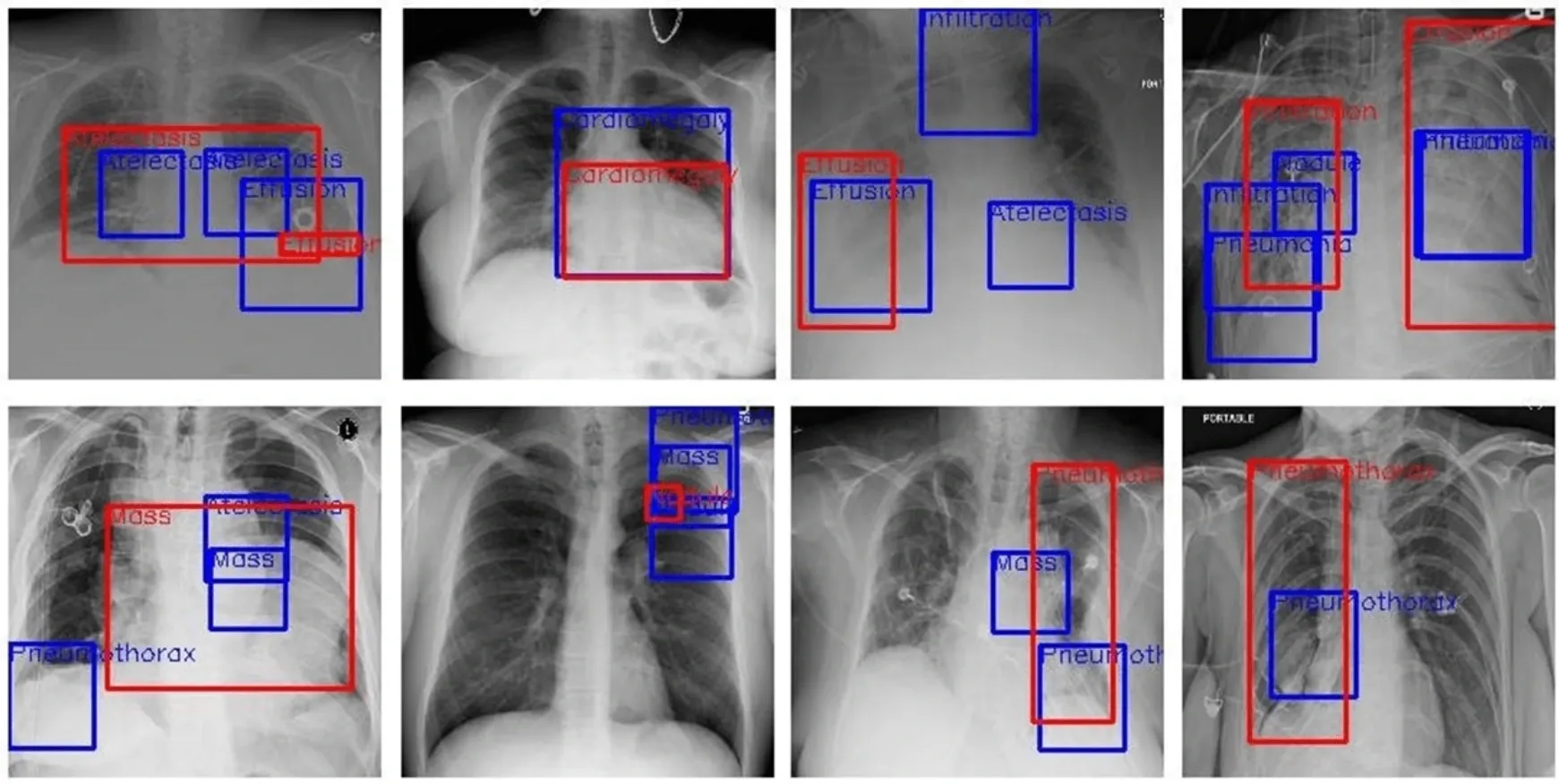
Ever wondered how AI doctors are learning to spot broken bones and sneaky tumors? It's all thanks to medical image annotation -a fancy way of saying "training AI to understand medical pictures." Just like teaching a kid their ABCs, we show the AI lots of labeled images, helping it recognize patterns and differences. This blog dives into the top 7 medical image annotation tools used by these AI doctors-in-training, giving you a peek behind the curtain of this medical marvel.
Table of Contents
Top 7 Medical Image Annotation Tools
1. Labellerr
Labellerr is your resource for accessing data-driven healthcare insights. Data is everything in the era of personalised medicine. However, raw data is not very useful without precise and effective annotation. This is where Labellerr comes into play, turning raw healthcare data into priceless insights that drive creativity and enhance patient care.
Why Labellerr is the Perfect Fit for Healthcare:
(i) Accuracy and Trustworthiness: Labellerr goes beyond simply labeling data. They specialize in healthcare, ensuring annotations are precise, consistent, and compliant with industry regulations.
(ii) Streamlined Data Flow: Their state-of-the-art platform and intelligent feedback loop simplify data management, saving you time and effort.
(iii) Insightful Information Extraction: From medical images to EHRs, Labellerr extracts hidden patterns and trends, leading to quicker diagnoses, better research, and personalized treatment plans.
Uses Cases where Labellerr is ideal:
(i) Medical Imaging: Precise annotations of X-rays, CT scans, and other images aid in faster and more accurate diagnosis, treatment planning, and surgical guidance.
(ii) EHR Analysis: Annotating EHRs for easier organization, faster data access, and improved research opportunities.
(iii) Telemedicine Support: Analyzing data from remote patients, enabling real-time monitoring and diagnosis, even in far-flung areas.
(iv) Precision Medicine: Annotating genetic data to develop personalized treatment strategies tailored to individual patients.
(v) Clinical Trials and Research: Make sense of clinical trial data to speed up research and drug development.
(vi) Healthcare IoT Data Annotation: Gain real-time insights and personalized health recommendations from annotated IoT data.
Building a Computer Vision Model in Healthcare with Labellerr:
- Identify Issues: Define the specific healthcare challenge you want to address with a computer vision model.
- Gather and Label Images: Train your model with high-quality, accurately annotated images relevant to your chosen issue with the help of Labellerr.
- Continuously Enhance and Scale: Labellerr provides ongoing support to refine your model and ensure its accuracy as you scale its use.
Maximizing the Utility of Medical Images:
Beyond simply labeling, Labellerr offers valuable post-implementation support to maximize the impact of your medical images:
- Utilize Public Datasets: Leverage existing high-quality datasets to jumpstart your project.
- Integrate Labeling and Training: Streamline the process by seamlessly integrating data labeling with model training.
- Incorporate Augmentations: Enhance your model's robustness by using augmented data generated from your labeled images.
- Prioritize Privacy and Security: Labellerr takes data security seriously, ensuring patient privacy is always protected.
With its commitment to accuracy, privacy, and expertise, Labellerr is the ideal partner for your healthcare data annotation needs. They empower you to unlock the potential of your data, driving innovation, improving patient care, and paving the way for a healthier future.
2. Encord
Encord is a sophisticated annotation platform tailored specifically for medical AI teams working with healthcare institutions. It focuses on annotating medical images to train machine learning and computer vision models.
Key Features:
(i) Native DICOM Rendering: It allows high-resolution image rendering in the browser with a user-friendly interface.
(ii) 3D Views: Supports multiple viewing angles for better understanding of medical images.
(iii) Windowing Support: Offers preset window settings for different body parts, aiding in efficient detection and annotation.
(iv) Hanging Protocols Support: Particularly useful for mammography, CT, and MRI images.
(v) Expert Review Workflows: Designed for collaboration among medical teams for scalable data operations.
(vi) Foundation Models Support: Uses AI to generate mask predictions, aiding in segmentation.
(vii) Configurable Labeling Protocols: Enables the creation of complex medical labels for training annotation teams.
(viii) Multiple Annotation Types: Supports various annotation types like bounding boxes, polygons, segmentation, etc.
Encord is best for teams deploying healthcare AI models, computer vision DataOps teams, and medical organizations.
3. 3D Slicer
3D Slicer is an open-source software primarily used for processing and visualizing medical images. It serves as a platform for 3D image segmentation and registration.
Key Features:
(i) Ease of Use: Simple and free tool for annotating DICOM files, suitable for manual annotation and semi-assisted labeling.
(ii) Robust Annotation Capabilities: Offers comprehensive annotation features for various computer vision applications.
3D Slicer is best for students, researchers, and academics starting with DICOM annotation. The tool is completely free to use.
4. Labelbox
Labelbox is a data annotation platform that supports various data types (images, videos, documents) for machine learning and AI use cases.
Key Features:
(i) Annotation Support: Allows polyline and segmentation annotations for CT, MRI, and ultrasound images.
(ii) Privacy and Security: Provides SaaS or on-premise workflows with robust privacy measures.
(iii) Catalog View: Helps in managing and finding patterns within extensive multi-format datasets.
Labelbox is best for teams requiring annotation of diverse file formats alongside DICOM.
5. Kili
Kili is a data annotation platform suitable for creating and managing annotation projects across various industries, including healthcare, finance, and retail.
Key Features:
(i) Annotation Variety: Supports multiple annotation types (text, image, video, audio).
(ii) Simplified DataOps: Aims to simplify data-centric AI workflows for AI teams of different sizes.
Kili is best for ML and DataOps teams across sectors, with in-house or outsourced teams. It offers a free tier for individuals and corporate/enterprise plans for businesses.
6. ITK-Snap
ITK-Snap is a free, open-source software for image segmentation and annotation in the medical field.
Key Features:
(i) Segmentation Support: Provides tools for manual segmentation in various image formats including DICOM and NIfTI.
(ii) Advanced Tools: Offers tools for faster processing of segmentation results across multiple images.
ITK-Snap is best for medical image annotation, students, and research teams. The tool is completely free to use.
7. MONAI
MONAI is an open-source PyTorch-based framework specifically designed for deep learning in medical imaging.
Key Features:
(i) Labeling Tool: Provides a labeling tool for annotating biomedical images.
(ii) Training Capabilities: Enables training AI models for medical imaging across different specialties.
MONAI is best for medical imaging and research teams needing an open-source AI platform. This annotation tool is also free to use.
Each tool caters to different user needs, offering various functionalities and levels of sophistication in medical image annotation, catering to diverse requirements within the healthcare and research domains.
Conclusion
From free and friendly options like 3D Slicer to feature-packed pros like Encord, there's a medical image annotation tool for everyone. Whether you're a researcher unlocking medical mysteries or a developer building the next generation of AI doctors, these tools are your key to unlocking the power of medical images. Remember, the next time you get an X-ray, it might just be an AI superhero reading it, trained by the amazing technology you just learned about.
Read our other listicles:
1. 7 Best Object Detection Tools For Computer Vision in 2024
2. Top 10 Auto Annotation & Labeling Tools in 2024
Frequently Asked Questions
1. What are medical image annotation tools?
Medical image annotation tools are software applications designed for the accurate labeling and marking of medical images. These tools assist healthcare professionals and researchers in identifying and categorizing specific structures or abnormalities within medical images, such as X-rays, MRIs, or CT scans.
Annotation tools play a crucial role in training machine learning models for medical image analysis, helping to improve diagnostic accuracy and streamline the interpretation of complex medical images. They enable the creation of annotated datasets, which are essential for developing and validating advanced image recognition algorithms in the field of medical imaging.
2. What are the use cases for medical annotations & labeling?
Medical annotations and labeling find diverse applications in healthcare, serving critical roles across various use cases. These include aiding in the training of machine learning models for medical image analysis, and enhancing the accuracy of diagnostic processes by precisely identifying and categorizing abnormalities in X-rays, MRIs, or CT scans.
Annotations also support medical research by facilitating the comparison of images over time and across different patient cases. Moreover, labeled medical datasets contribute to the development and validation of advanced image recognition algorithms, ultimately improving the efficiency and reliability of automated diagnostic tools in the healthcare domain.
3. Why should medical images be annotated?
Medical images should be annotated because annotations provide crucial information for training and validating machine learning models in the field of medical image analysis. Accurate and detailed annotations help identify and label specific structures, abnormalities, or regions of interest in medical images, enabling the development of precise and reliable algorithms.
Annotation also aids healthcare professionals in improving diagnostic accuracy, as annotated images serve as valuable reference points for identifying and understanding various medical conditions. Additionally, annotated datasets contribute to the creation of robust and effective AI systems that can assist in the interpretation of complex medical images, ultimately enhancing patient care and outcomes.
Book our demo with one of our product specialist
Book a Demo