Labellerr vs Supervisely: Better Alternative To Faster Annotation
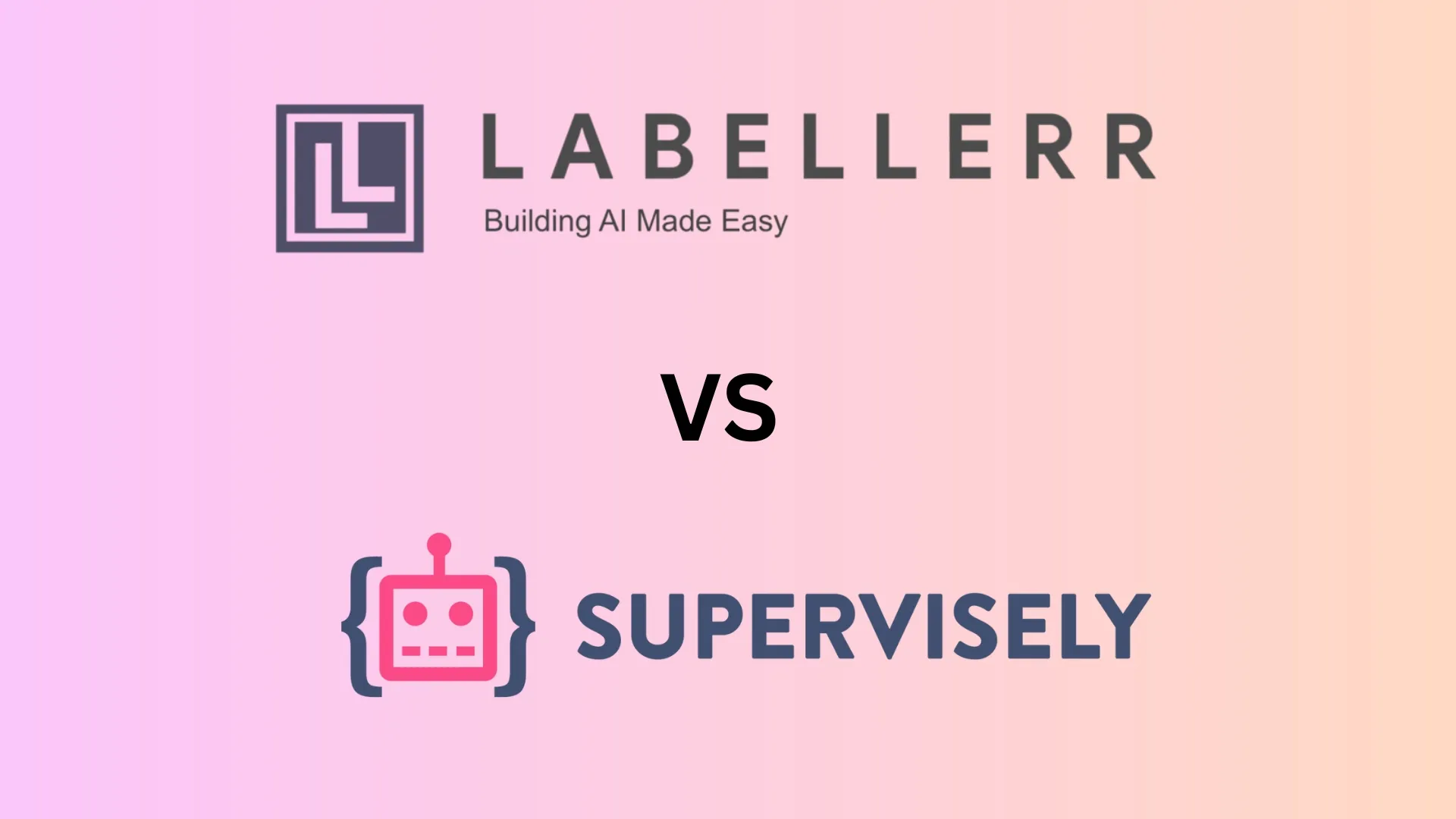
Are you tired of manually labeling massive datasets for your AI and machine learning projects? The process can be painstakingly slow, requiring careful attention to detail, with one small mistake jeopardizing the accuracy of your entire model.
It’s a common frustration in the world of AI development, but what if there was a way to automate the process and ensure precise results?
That’s where advanced data annotation tools like Labellerr and Supervisely come into play. These platforms take the burden off your shoulders by automating the labeling process, making it faster, more efficient, and more accurate.
According to Grand View Research, the global data annotation tools market was valued at USD 1.02 billion in 2023, and it’s projected to grow at a CAGR of 26.3% from 2024 to 2030.
This growth is fueled by the increasing demand across industries like automotive, healthcare, and retail all of which rely on high-quality data to power their AI systems.
Accurate labeling, whether for images, videos, or text, is crucial for building effective AI models. When comparing Labellerr and Supervisely, both offer unique features and strengths, but Labellerr emerges as the superior choice for managing large-scale, complex projects with unmatched precision.
In this blog, we’ll dive into a detailed comparison of Labellerr and Supervisely, exploring their features, capabilities, and use cases.
1. Overview of Data Annotation in Machine Learning
Data annotation is the process of labeling data, which is essential for training machine learning models.
Whether you're working on image classification, object detection, or natural language processing, the accuracy of your annotations directly influences the effectiveness of your models.
This process is crucial in ensuring that AI systems can recognize patterns, make predictions, and ultimately deliver reliable results.
Importance of Choosing the Right Platform
Choosing the right data annotation platform is a critical decision that can significantly impact the success of your project.
The platform you select should not only support the types of data you work with but also offer the scalability, accuracy, and efficiency required for your specific needs.
Factors to consider:
- Data Quality: Ensuring precise and consistent annotations.
- Efficiency: Speeding up workflows and reducing manual labor.
- Scalability: Handling large datasets without compromising performance.
- Flexibility: Adapting to existing workflows and offering customization.
- Managed Services: Providing support for complex projects.
Labellerr and Supervisely cater to different project needs, but as we will explore, Labellerr excels in offering comprehensive solutions that meet the demands of large-scale, high-stakes projects.
Labellerr is a versatile data annotation platform that supports multiple data types, including images, videos, and text. It is designed for large-scale, complex projects and offers managed services like human-in-the-loop workflows, data validation, and quality assurance.
Labellerr's platform is known for its scalability, accuracy, and ability to handle diverse annotation tasks.
Supervisely is a data annotation and computer vision platform that primarily focuses on image and video data. It offers a range of tools for object detection, segmentation, and classification, making it a popular choice for teams working on computer vision projects.
While it provides robust features for image-related tasks, it lacks the broader support for multiple data types and complex workflows that Labellerr offers.
2. Platform Overview
Labellerr: Key Features and Capabilities
Labellerr is built to support complex, large-scale annotation projects across various industries. Some of its key features include:
- Multi-Data Type Support: Labellerr supports images, videos, and text, making it versatile for different project requirements.
- Human-in-the-Loop (HITL): Ensures high accuracy by incorporating human oversight in complex tasks.
- Scalability: Labellerr is designed to handle large datasets efficiently, making it ideal for ongoing, large-scale projects.
- Managed Services: Labellerr offers expert annotation teams, ensuring quality and consistency across all tasks.
- Customizable Workflows: Allows teams to create custom workflows that align with specific project needs.
Supervisely: Key Features and Capabilities
Supervisely is a powerful platform focused primarily on computer vision tasks. Its key features include:
- Image and Video Annotation: Specialized tools for object detection, segmentation, and classification.
- Project Management: Tools for tracking progress and managing large teams.
- Integration with ML Frameworks: Seamless integration with popular machine learning frameworks like TensorFlow and PyTorch.
- Collaboration Features: Facilitates teamwork with collaboration tools.
3. Data Annotation Capabilities
Supported Data Types (Images, Videos, Text)
Labellerr supports a broad range of data types, including images, videos, and text, making it suitable for diverse industries like healthcare, finance, and retail.
Read more such customer reviews of Labellerr on G2 here.
Supervisely, on the other hand, primarily focuses on image and video data, which may limit its applicability for projects requiring text or multi-data type support.
Read G2 customer reviews of Supervisely here.
Annotation Tools and Techniques
Labellerr provides advanced annotation tools for object detection, segmentation, classification, and more.
It also offers customizable annotation workflows, enabling teams to tailor the process according to their project needs.
Supervisely’s tools are robust for image and video annotation but lack the flexibility and customization options that Labellerr provides.
Flexibility and Customization
Labellerr stands out for its highly customizable workflows, allowing users to adapt the platform to their unique requirements.
This flexibility is essential for complex projects that require specific annotation techniques or processes. While Supervisely offers some customization, it does not match the level of flexibility offered by Labellerr.
4. Integration and Workflow
API and Third-Party Integration
Labellerr offers robust APIs that facilitate seamless integration with third-party tools and existing workflows. This makes it easy for teams to incorporate Labellerr into their current systems without disruption.
Supervisely also provides integration options but is more focused on specific ML frameworks, which might limit its adaptability in broader use cases.
Workflow Automation and Custom Pipelines
Labellerr excels in workflow automation, allowing teams to automate repetitive tasks and streamline the annotation process.
Its custom pipelines enable users to design workflows that fit their exact needs, enhancing efficiency and productivity. Supervisely offers basic automation but does not provide the same level of customization as Labellerr.
Collaboration Features
Both Labellerr and Supervisely offer collaboration features, but Labellerr’s platform is more comprehensive, supporting larger teams and complex projects.1
Its managed services ensure that collaboration is smooth and that quality is maintained across the board.
5. Scalability and Performance
Handling Large Datasets
Labellerr is designed to scale efficiently, making it the ideal choice for projects with large datasets.
Its platform can handle significant volumes of data without compromising on performance, ensuring that projects run smoothly from start to finish.
Supervisely is capable of handling medium to large datasets but may struggle with the scale and complexity that Labellerr manages effortlessly.
Performance in Complex Projects
For complex projects that require high accuracy and detailed annotations, Labellerr’s platform excels.
It combines human-in-the-loop workflows with automation to deliver precise results, even in challenging scenarios. Supervisely performs well in simpler projects but may fall short when it comes to managing the intricacies of large-scale, multi-data type projects.
Managed Services and Human-in-the-Loop
Labellerr’s managed services, including human-in-the-loop annotation, are a significant advantage for companies that require high-quality annotations.
This feature ensures that even the most complex tasks are completed with precision. While Supervisely offers some level of human oversight, it does not provide the comprehensive managed services that Labellerr does.
6. Data Quality and Accuracy
Quality Assurance Features
Labellerr places a strong emphasis on quality assurance, offering tools for data validation, review, and correction.
This ensures that annotations meet the highest standards, which is critical for industries where accuracy is paramount, such as healthcare or autonomous driving. Supervisely also offers quality assurance features, but they are more limited in scope compared to Labellerr.
Human-in-the-Loop (HITL) and Data Validation
Labellerr’s human-in-the-loop workflows are a standout feature, providing an extra layer of accuracy in complex annotation tasks.
This, combined with its data validation tools, ensures that the final output is of the highest quality. Supervisely, while offering some level of data validation, does not match the comprehensive approach of Labellerr.
Automation and Efficiency
Labellerr combines automation with human oversight to maximize efficiency without sacrificing accuracy.
This balanced approach allows for quick turnaround times, as evidenced by the 99.5% accuracy achieved in just three days, as mentioned by Will Dehnert of Perceptly Inc. Supervisely also uses automation but does not provide the same level of efficiency, particularly in large-scale projects.
7. Pricing and Cost-Effectiveness
Pricing Models and Plans
Labellerr offers flexible pricing models that cater to different project sizes and requirements.
This makes it a cost-effective option for both small startups and large enterprises. Supervisely also offers various pricing plans, but they may not provide the same level of flexibility or value, especially for large-scale, complex projects.
Cost Comparison for Different Project Sizes
For large-scale projects, Labellerr’s pricing is competitive, offering excellent value for the comprehensive services it provides. Supervisely may be more cost-effective for smaller, simpler projects but could become more expensive as the project scale increases.
ROI and Long-Term Value
Labellerr offers a strong return on investment, particularly for long-term projects that require ongoing data annotation.
Its managed services, combined with its scalability and accuracy, ensure that teams get the most value from their investment. Supervisely also offers good ROI, but it may not match the long-term value provided by Labellerr, especially in complex projects.
8. Best Use Cases
When to Choose Labellerr
Labellerr is the ideal choice for:
- Large-scale, complex projects requiring high accuracy.
- Projects involving multiple data types (images, videos, text).
- Teams need customizable workflows and managed services.
- Industries where data quality and precision are critical, such as healthcare, autonomous driving, and finance.
When to Choose Supervisely
Supervisely is best suited for:
- Smaller to medium-sized projects focused on computer vision.
- Teams that primarily work with image and video data.
- Projects with simpler annotation requirements.
Conclusion
Labellerr excels in handling large-scale, complex projects that require high accuracy and flexibility.
Its support for multiple data types, customizable workflows, and managed services make it a superior choice for teams needing comprehensive solutions. Supervisely, while a strong contender in the computer vision space, does not offer the same level of versatility or scalability.
For teams working on large, multi-data type projects, or those requiring high accuracy and comprehensive managed services, Labellerr is the clear winner.
It offers the flexibility, efficiency, and scalability needed to ensure the success of even the most challenging projects. Supervisely remains a solid choice for smaller, image-focused projects, but it may not meet the demands of more complex use cases.
Frequently Asked Questions (FAQ)
Which platform is better for large-scale projects?
Labellerr is better suited for large-scale projects due to its scalability and managed services.
Can I use these platforms for text annotation?
Labellerr supports text annotation, while Supervisely focuses primarily on images and videos.
Which platform offers better integration with existing workflows?
Labellerr offers robust API and third-party integrations, making it more adaptable to existing workflows.
Book our demo with one of our product specialist
Book a Demo