Labellerr vs Roboflow: A Comparitive Analysis
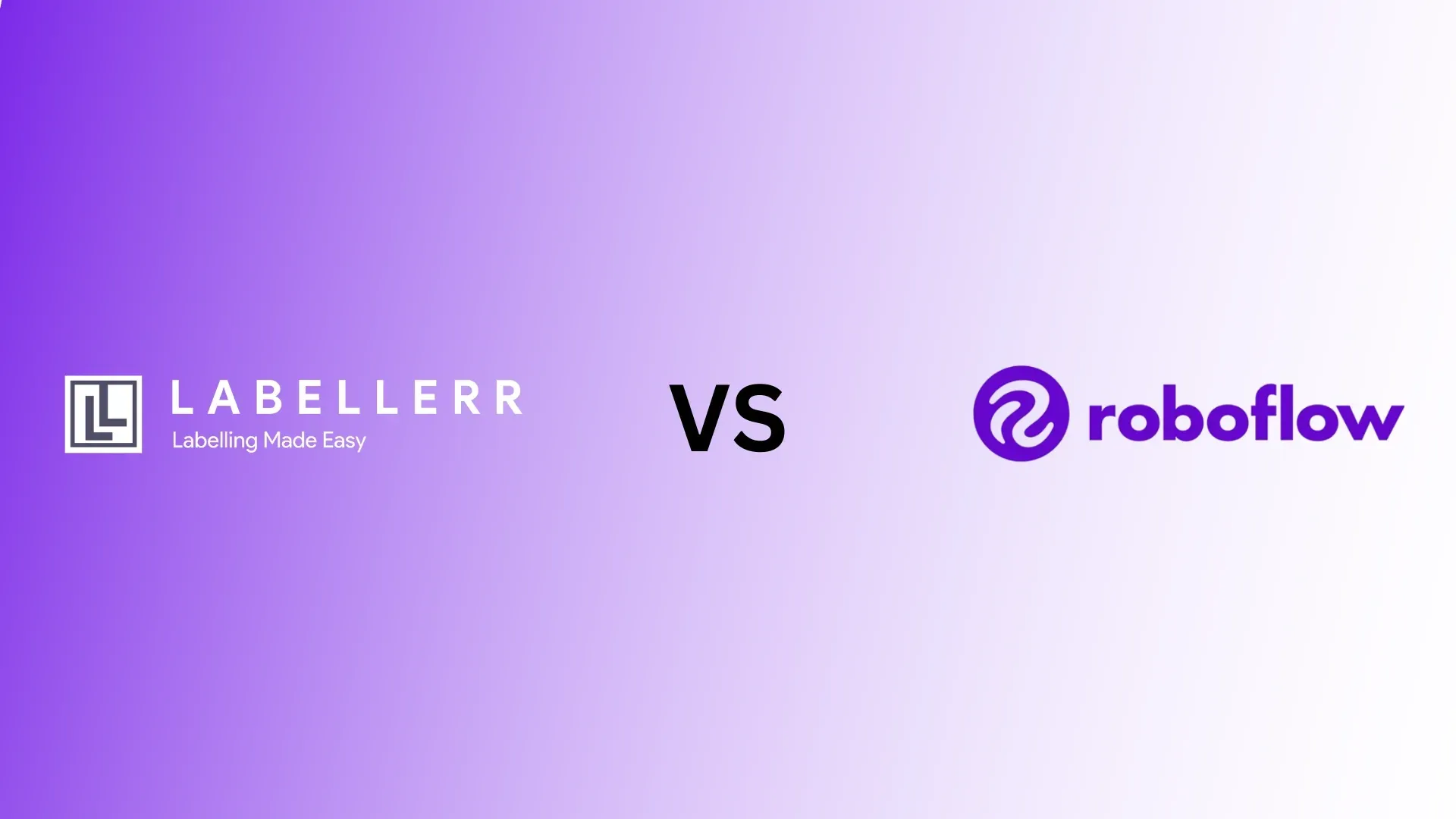
Global data annotation tools market was valued at approximately $1.355 billion in 2020 and is projected to grow to $13.696 billion by 2030, highlighting the increasing reliance on these tools for AI development.
Two leading platforms in this space are Labellerr and Roboflow, each bringing distinct strengths tailored to different project needs.
Labellerr excels in large-scale, complex annotation tasks across multiple data types, offering managed services and customizable workflows.
On the other hand, Roboflow focuses on more streamlined, computer vision-based projects, providing a user-friendly interface for quick image annotation and model deployment.
In this article, we’ll dive into a detailed comparison between Labellerr and Roboflow, exploring their features, strengths, and best use cases.
Whether you’re managing large datasets requiring precise annotation or building a computer vision model, this guide will help you determine which tool aligns with your specific project needs.
1. Overview of Data Annotation Tools
Data annotation tools allow users to label various data types (images, videos, text, etc.) to create structured datasets for machine learning. The common use cases include:
1. Computer Vision: Image classification, object detection, segmentation.
2. Natural Language Processing (NLP): Text classification, named entity recognition, sentiment analysis.
3. Audio Analysis: Speech recognition, transcription.
These tools enhance data quality and accuracy, efficiency, and scalability, ensuring the success of machine learning projects.
2. Importance of Choosing the Right Platform
The right data annotation platform can significantly impact the success of your project. Factors to consider include:
1. Data Quality: Ensures precise, consistent annotations.
2. Efficiency: Reduces manual annotation, speeding up workflows.
3. Scalability: Manages large datasets without performance issues.
4. Flexibility: Adapts to your existing workflows and offers customization.
5. Managed Services: For large, complex projects, having managed services is vital.
Labellerr and Roboflow cater to different project needs, offering varying strengths in terms of functionality, integration, and scalability.
3. Project Size and Complexity
Labellerr is well-suited for medium to large-sized projects, supporting complex annotation tasks across multiple data types (images, videos, text).
It provides managed services such as human-in-the-loop workflows and data validation to ensure high-quality annotations.
The platform scales efficiently, making it ideal for ongoing, large-scale projects with extensive annotation requirements.
Key Benefits:
1. Support for multiple data types.
2. Managed services for quality assurance.
3. Scalable for large datasets.
On the other hand, Roboflow focuses on medium-sized projects, particularly in computer vision. It excels at image annotation and model deployment, making it an excellent choice for developers working on quick model training and deployment.
However, it lacks the comprehensive support for multi-data type projects that Labellerr offers.
Key Benefits:
1. Optimized for computer vision tasks.
2. Simple, intuitive interface.
3. Quick model deployment.
4. Features and Functionality
Labellerr offers versatile support for images, videos, and text, making it ideal for teams working across industries such as healthcare, retail, and NLP. The platform supports various annotation types like object detection, segmentation, and classification.
Additionally, Labellerr provides customizable workflows and managed services for high-quality annotations in complex projects.
Key Features:
1. Multi-data type support.
2. Tools for classification, bounding boxes, segmentation.
3. Managed services for quality assurance.
Roboflow is tailored for image annotation and model deployment. Its streamlined tools simplify tasks like object detection, classification, and segmentation.
The platform also excels in dataset management and model training, making it a go-to solution for teams focused on computer vision.
Key Features:
1. Focused on computer vision tasks.
2. Tools for image classification and object detection.
3. Seamless integration with TensorFlow, PyTorch, and other frameworks.
5. Ease of Use
Labellerr offers a user-friendly interface with onboarding support, making it easy for teams to start annotating datasets quickly.
Its customizable workflows ensure flexibility, allowing users to adapt the platform to their specific needs. Collaboration features enable teams to work efficiently on large-scale projects.
1. Free onboarding support.
2. Customizable workflows for complex projects.
3. Collaboration features for team efficiency.
Roboflow is known for its simplicity, particularly for image annotation. Its drag-and-drop interface, combined with automated data preprocessing, helps developers quickly label datasets and build models.
It’s an ideal solution for quick setup and model deployment, particularly for small to medium-sized teams working on computer vision.
1. Intuitive, straightforward interface.
2. Minimal setup time for image annotation.
3. Streamlined workflow for quick deployment.
6. Integration and Flexibility
Labellerr provides robust APIs and custom pipelines, enabling teams to integrate the platform seamlessly into their existing workflows.
It supports flexible workflows for large-scale projects and diverse data types, making it a highly adaptable platform for enterprises.
Key Benefits:
1. APIs for seamless integration.
2. Support for custom workflows and diverse data types.
Roboflow is optimized for computer vision workflows, with easy integration into machine learning frameworks like TensorFlow and PyTorch. The platform also offers automated preprocessing for image datasets, streamlining model training and deployment.
Key Benefits:
1. Integration with popular computer vision frameworks.
2. Automated dataset preprocessing.
7. Data Quality and Accuracy
Labellerr prioritizes high-quality annotations, especially for projects with complex labeling requirements like healthcare or autonomous driving. The platform ensures accuracy through:
- Human-in-the-Loop (HITL): Human oversight for complex tasks, improving accuracy.
- Data Validation: Built-in tools for reviewing and verifying annotations.
- Managed Services: Expert annotation teams for large-scale projects, ensuring consistency.
Labellerr’s HITL workflows and data validation tools make it a reliable choice for projects where precision is critical.
Roboflow primarily relies on user-generated annotations but also offers:
- Automation Tools: Features like auto-labeling and data augmentation to speed up the process.
- User-Driven Quality: The accuracy depends on the expertise of the users, with limited validation features.
While Roboflow’s automation is helpful for speeding up annotations, it might not match Labellerr’s level of accuracy in complex projects.
Labellerr’s data validation tools and managed services make it ideal for high-stakes industries, whereas Roboflow is better suited for projects needing quick and efficient annotation.
8. Scalability and Suitability
Labellerr scales efficiently for large, ongoing projects with high volumes of data. Its platform supports a wide range of data types (images, videos, text) and complex workflows, making it suitable for:
- Autonomous Driving: Accurate video data annotations.
- Healthcare: Medical imaging and diagnostics.
- Finance: Text annotation for compliance and fraud detection.
Labellerr’s scalability and managed services ensure that quality is maintained even as project sizes grow.
Roboflow is more tailored for medium-sized computer vision projects. It excels in:
- Image Annotation: Focus on quick image labeling and model deployment.
- Streamlined Workflows: Optimized for computer vision tasks like object detection and facial recognition.
For smaller datasets and teams needing fast annotation for image-based models, Roboflow is an efficient solution.
Labellerr is ideal for large, diverse data annotation projects, while Roboflow shines in medium-sized image-based tasks.
9. Pricing Models
Labellerr
Labellerr offers custom pricing, making it flexible for enterprises and teams with specific needs.
Costs depend on factors like data volume and managed services, which makes it cost-effective for large-scale projects requiring high accuracy.
Roboflow
Roboflow typically offers tiered pricing based on features and data usage, which can be more predictable but may limit flexibility for complex projects.
Labellerr’s custom pricing is a better fit for teams with specific requirements, while Roboflow’s tiered model is more straightforward for smaller projects.
10. Best Use Cases
Labellerr
Labellerr is best suited for teams handling:
- Autonomous Vehicles: Accurate video annotations with managed services.
- Healthcare: Medical imaging requiring high accuracy.
- Retail and Finance: Multi-data type annotations for product classification and fraud detection.
With its emphasis on flexibility, Labellerr excels in large, ongoing projects where precision is essential.
Roboflow
Roboflow is optimized for:
- Object Detection: Projects like security systems or retail analysis.
- Facial Recognition: For surveillance or customer analytics.
- Drone and Satellite Imagery: Agricultural monitoring and urban planning.
- Custom Computer Vision Models: Quick setup for model training and deployment.
Roboflow is ideal for medium-sized projects focused on computer vision, especially when speed and ease of use are priorities.
Conclusion
When comparing Labellerr and Roboflow, both platforms offer unique advantages depending on the scope and type of the project.
Roboflow is an excellent solution for teams focusing on medium-sized, image-centric computer vision projects where quick annotation and model deployment are essential.
It excels in simplicity and efficiency, particularly for projects like object detection, facial recognition, and drone imagery.
However, Labellerr stands out as the better choice for more complex, large-scale projects that require high accuracy and scalability across multiple data types like images, videos, and text.
Labellerr's strengths lie in:
- High-Quality Data Annotations: Its Human-in-the-Loop (HITL) workflows, data validation tools, and managed services ensure precise annotations, making it ideal for industries like healthcare, autonomous driving, and finance, where accuracy is critical.
- Scalability: Labellerr can handle vast datasets while maintaining performance, making it suitable for ongoing projects with diverse and complex data needs.
- Customizable Workflows: Labellerr’s flexibility allows teams to tailor their workflows, adapting to the specific needs of their project.
- Comprehensive Multi-Data Support: Unlike Roboflow, which focuses mainly on computer vision tasks, Labellerr supports multiple data types, making it a more versatile option.
FAQ
1. What types of projects are best suited for Labellerr?
Labellerr is ideal for large-scale, complex projects that require high accuracy and support for multiple data types (images, videos, text). For example, autonomous driving, healthcare, and finance.
2. When should I choose Roboflow over Labellerr?
Roboflow is best suited for medium-sized computer vision projects where speed, ease of use, and quick deployment are priorities. It excels in image-centric tasks such as object detection, facial recognition, and drone/satellite imagery.
3. How does Labellerr ensure high data quality and accuracy?
Labellerr uses Human-in-the-Loop (HITL) workflows, and built-in data validation tools, and offers managed services to ensure consistent, high-quality annotations.
These features make it a strong choice for projects requiring precise data labeling.
Book our demo with one of our product specialist
Book a Demo