Labellerr vs. Dataloop
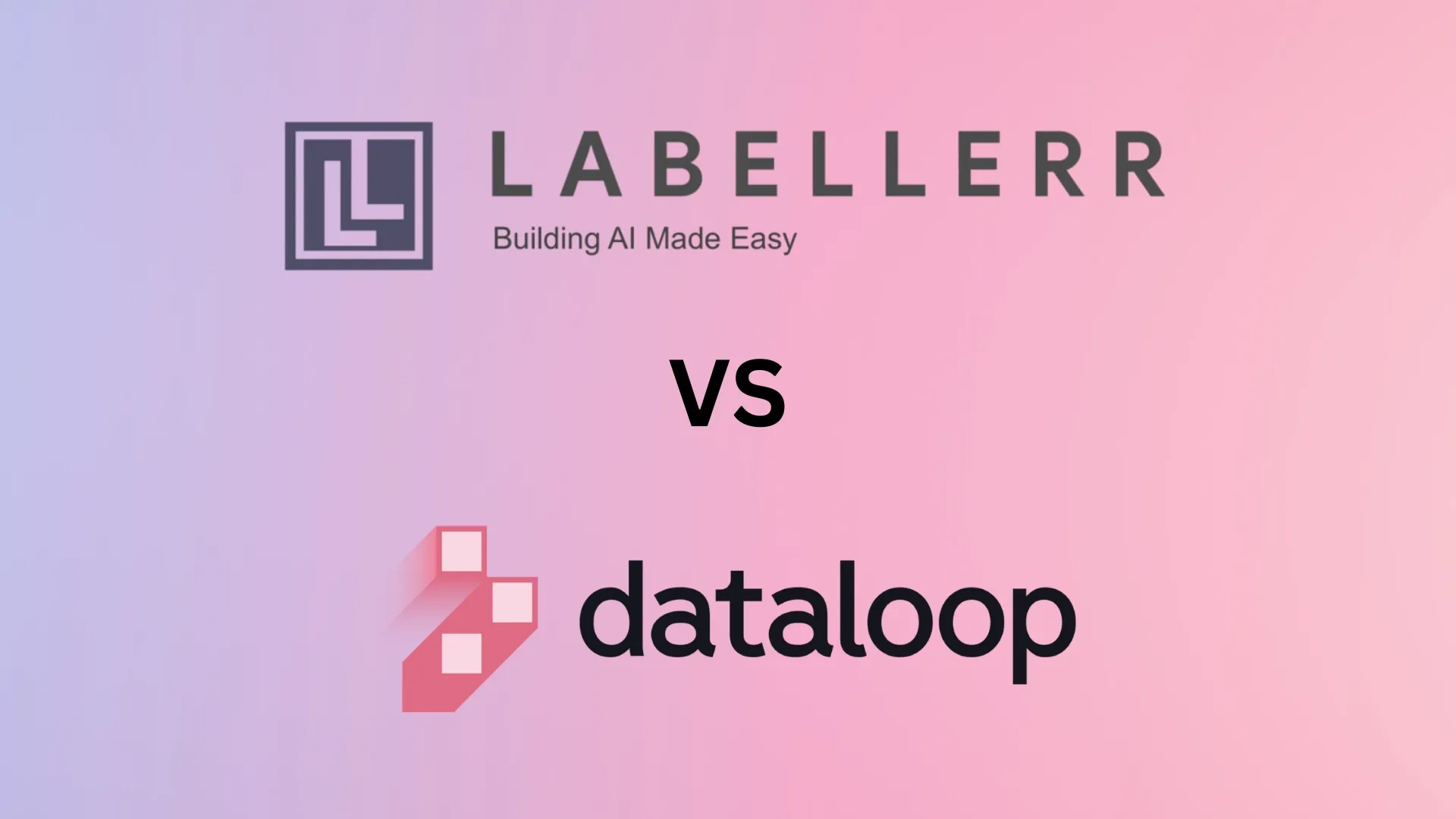
Are you overwhelmed by the complex task of labeling vast datasets for AI/ML projects? Whether it’s annotating images, videos, or text, the process can feel never-ending, with each mislabeled data point potentially derailing your entire model’s performance.
It’s a challenge that every AI developer knows all too well. But what if there were a way to simplify this crucial step, ensuring speed and accuracy without the manual grind?
Enter data annotation platforms like Labellerr and Dataloop, which are designed to automate and streamline the labeling process, saving you time and boosting efficiency.
As the data annotation market continues to grow, it’s expected to reach a valuation of $8.22 billion by 2028, with a CAGR of 26.6%. This highlights the increasing demand for advanced, high-quality annotation tools that can handle the complexity of AI/ML projects across various industries from autonomous vehicles to healthcare.
Choosing the right platform, however, can be daunting. With so many options available, it’s essential to evaluate key factors like data annotation capabilities, user experience, scalability, and pricing.
In this article, we dive deep into a comparison between two leading platforms: Labellerr and Dataloop. We’ll break down their features and show why Labellerr might be the better choice for your unique project needs.
Table of Contents
- Overview of Data Annotation Tools
- Platform Overviews
- Data Annotation Capabilities
- Ease of Use
- Integration and Workflow Automation
- Scalability and Performance
- Collaboration and Team Features
- Pricing Models
- Use Cases and Industry Applications
- Customer Support and Community
- Pros and Cons
- Conclusion
- FAQs
Overview of Data Annotation Tools
Data annotation tools are essential in structuring datasets, whether they involve images, videos, text, or other data types. These platforms enable AI models to learn from well-annotated datasets, leading to better performance in real-world applications.
Importance of Choosing the Right Platform
Choosing the right data annotation platform can make or break your AI/ML project. Factors such as accuracy, speed, scalability, and ease of use are critical. Labellerr and Dataloop are both robust options, but they cater to different needs and project scales.
What does Labellerr and Dataloop do?
Labellerr is known for its strong analytics, efficient project management, and ability to handle large-scale projects across various industries. Dataloop, on the other hand, focuses on providing a comprehensive platform for end-to-end AI lifecycle management, including data labeling.
Platform Overviews
Labellerr: Key Features and Strengths
- Advanced analytics for quality control
- Scalable solutions for large datasets
- Managed services and Human-in-the-Loop (HITL) features
- Customizable workflows and seamless integration with existing tools
Dataloop: Key Features and Strengths
- Comprehensive platform for AI lifecycle management
- Robust support for a wide range of data types
- High flexibility with API and SDK integrations
- Strong focus on automation and efficiency
Data Annotation Capabilities
Supported Data Types (Images, Videos, Text, etc.)
- Labellerr: Supports a wide array of data types, making it versatile for various AI projects.
- Dataloop: Also supports multiple data types, with a strong emphasis on structured data workflows.
Annotation Tools and Techniques
- Labellerr: Offers tools for precise annotations, including polygon, bounding box, and semantic segmentation.
- Dataloop: Provides similar tools with added automation features for quicker labeling.
Quality Assurance and Data Validation
- Labellerr: Implements rigorous QA processes, with the ability to track and validate annotations through analytics.
- Dataloop: Also offers QA tools but focuses more on automated validation.
Ease of Use
User Interface and Experience
- Labellerr: Designed for intuitive use, with an emphasis on user-friendly navigation and minimal learning curve.
Read the product review on G2 here.
- Dataloop: Offers a more complex interface, which may require a steeper learning curve.
Onboarding and Learning Curve
- Labellerr: Quick onboarding process, supported by comprehensive documentation and responsive customer support.
- Dataloop: Onboarding may take longer due to the platform’s broad capabilities, but it is supported by detailed guides.
Customization and Flexibility
- Labellerr: Highly customizable, allowing users to adapt the platform to their specific workflows.
- Dataloop: Also customizable, with extensive options for API and SDK integrations.
Integration and Workflow Automation
API and SDK Availability
- Labellerr: Provides robust API support, allowing seamless integration with various machine learning frameworks.
- Dataloop: Offers extensive API and SDK tools, designed for full lifecycle management.
Integration with Machine Learning Frameworks
- Labellerr: Easily integrates with popular frameworks like TensorFlow and PyTorch.
- Dataloop: Also supports integration with major ML frameworks, with added tools for end-to-end project management.
Automation Capabilities (Pipelines, Scripting, etc.)
- Labellerr: Focuses on simplifying automation processes, making it easier for teams to streamline workflows.
- Dataloop: Provides extensive automation tools, but may require more setup and customization.
Scalability and Performance
Handling Large Datasets
- Labellerr: Designed for scalability, making it ideal for handling large datasets without compromising performance.
- Dataloop: Also scalable, but the complexity of its platform may affect performance with very large projects.
Speed and Efficiency
- Labellerr: Known for its rapid turnaround times, as highlighted by Tyler Kapp, CEO of Butterfly Positronics, "Labellerr helps me get months of work in just a few weeks."
- Dataloop: Focuses on efficient processing, but the broader scope of its platform may lead to slower initial setup.
Managed Services and Human-in-the-Loop (HITL) Features
- Labellerr: Excels in providing managed services, ensuring high-quality annotations with minimal oversight.
- Dataloop: Also offers HITL features, but may require more user involvement to achieve optimal results.
Collaboration and Team Features
Multi-user Support and Collaboration Tools
- Labellerr: Offers robust collaboration features, making it easy for teams to work together on large-scale projects.
- Dataloop: Provides similar collaboration tools, with a focus on integrated project management.
Project Management and Reporting
- Labellerr: Strong project management capabilities, supported by detailed reporting and analytics.
- Dataloop: Also strong in project management, with additional tools for tracking AI lifecycle progress.
Pricing Models
Overview of Pricing Structures
- Labellerr: Offers flexible pricing plans tailored to different project sizes, with options for subscription or pay-as-you-go models.
- Dataloop: Provides custom pricing based on project requirements, which may be less predictable for smaller projects.
Cost-effectiveness for Different Project Sizes
- Labellerr: Generally more cost-effective for large-scale projects due to its efficient management and lower overhead.
- Dataloop: May be more expensive due to its comprehensive feature set, especially for smaller projects.
Use Cases and Industry Applications
Best Use Cases for Labellerr
- Large-scale projects requiring high-quality annotations
- Industries like healthcare, autonomous vehicles, and robotics
- Teams looking for quick, efficient annotation with minimal setup
Best Use Cases for Dataloop
- End-to-end AI lifecycle management
- Complex projects requiring extensive customization and integration
- Industries needing detailed project tracking and management
Customer Support and Community
Availability and Quality of Support
- Labellerr: Highly praised for its responsive customer support, as noted by Jaideep Rayapudi, VP Clinical Solutions at Foresight Health Solutions, "Labellerr's powerful analytics gives me full control on the quality of the output as well as the project management."
- Dataloop: Offers solid support, but may be less responsive due to the platform’s complexity.
Documentation and Resources
- Labellerr: Comprehensive documentation, easy to navigate and understand.
- Dataloop: Extensive resources, though they may require more time to fully digest due to the platform's breadth.
Community and Ecosystem
- Labellerr: Growing community with active participation and resource sharing.
- Dataloop: Established community, but more focused on advanced users and developers.
Pros and Cons
Strengths and Weaknesses of Labellerr
- Strengths: High scalability, quick setup, strong support, cost-effective for large projects.
- Weaknesses: Does not support dicom and point cloud annotations.
Strengths and Weaknesses of Dataloop
- Strengths: Comprehensive toolset, strong for end-to-end lifecycle management, highly customizable.
- Weaknesses: Steeper learning curve, higher costs, potential for slower initial setup.
Conclusion
Labellerr excels in scalability, speed, and cost-effectiveness, making it ideal for large-scale, high-quality annotation projects.
Dataloop, while offering a more comprehensive toolset, may be better suited for projects requiring extensive customization and end-to-end lifecycle management.
For teams looking to quickly scale annotation projects with minimal setup, Labellerr is the better choice. For those needing detailed project tracking and lifecycle management, Dataloop offers a more tailored solution.
FAQs
Which platform is better for large datasets?
Labellerr is known for its high scalability and efficiency with large datasets, making it a strong choice. Dataloop also handles large datasets well but is more geared toward comprehensive lifecycle management.
How do the pricing models compare?
Labellerr offers cost-effective solutions for larger projects, while Dataloop tends to have higher costs associated with its more extensive features and capabilities. It’s essential to review both platforms' pricing structures based on your specific needs.
What are the best use cases for each platform?
Labellerr excels in scenarios requiring quick setup and strong support for large datasets. Dataloop is ideal for projects needing extensive customization and end-to-end lifecycle management, particularly in more complex workflows.
How do Labellerr and Dataloop handle scalability?
Both platforms handle scalability well, but Labellerr is specifically designed for high scalability, allowing users to efficiently manage increasing data volumes. Dataloop also supports scalability, but its performance may vary based on the complexity of the projects.
What support options are available for each platform?
Labellerr provides robust support, including documentation and customer service. Dataloop also offers strong support options, with detailed resources and community forums, but may require more extensive onboarding due to its complexity.
Book our demo with one of our product specialist
Book a Demo