Human Emotion Recognition Annotation using Labellerr
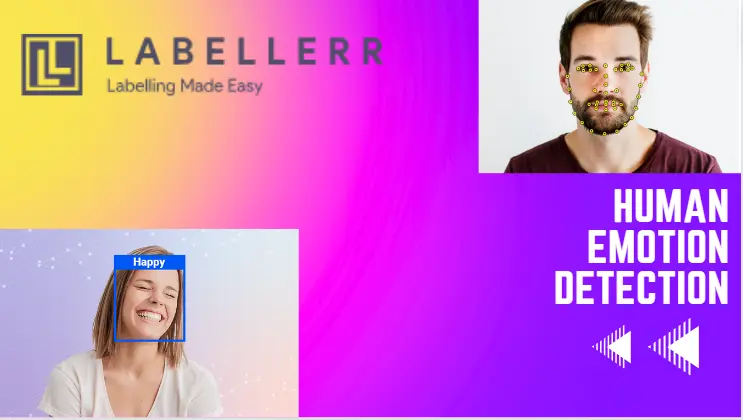
Table of Contents
- Introduction
- Solution: Labellerr's Role in Human Emotion Detection
- Conclusion
- Frequently Asked Questions
Introduction
In the world of artificial intelligence and human-computer interaction, the ability to accurately recognize and interpret human emotions is very important.
From personalized user experiences to mental health assessments, human emotion recognition plays a pivotal role in various applications across industries.
One of the fundamental steps in developing emotion recognition systems is the annotation of emotional expressions in images or videos.
Labellerr offers advanced capabilities to facilitate the annotation of emotional faces for training Deep learning models.
Challenges
Annotating human emotions has significant challenges due to the complex and subjective nature of emotional expressions.
Subjectivity of Emotions: Different people can convey the same feeling in different ways, and even trained eyes can disagree on the meaning of a video or picture. Consistent annotation becomes challenging due to this subjectivity.
Context Dependence: Emotional expression frequently depends on the surrounding situation. In one context, a brow raised may indicate that the person is angry, but in another, it might indicate that the person is attentive or concentrated. When categorizing emotional states, annotators should keep the surrounding context in mind.
Differences in Cultural Norms and Practices: Different cultures have different standards for how people should show their emotions through facial expressions. If annotators don't know the subject's cultural background, they could misunderstand expressions.
Data Ambiguity: In low-resolution photos or videos, facial emotions might be subtle, which can lead to data ambiguity. Distinguishing between happy and surprised feelings, or between grief and disappointment, isn't always easy. It is difficult to accurately annotate expressions that are ambiguous.
Dataset Limitations: The size and diversity of publicly available HER datasets may be restricted. Models' generalizability to new situations and emotions may suffer as a result.
To overcome these challenges annotators need attention to detail, and advanced annotation tools to ensure the accuracy and reliability of annotated emotional data for training machine learning models.
Solution: Labellerr's Role in Human Emotion Detection
Intuitive Interface
Labellerr provides an intuitive user interface designed to simplify the annotation process for annotators working with large datasets.
Its user-friendly design and navigation tools enable annotators to label data with ease, reducing the learning curve and enhancing productivity.
Labellerr's intuitive interface allows annotators to focus on labeling tasks without being overwhelmed by the size of the dataset.
Robust Segmentation Features
Labellerr incorporates robust segmentation features that enable accurate and precise annotation of complex datasets.
Its advanced algorithms can effectively outline objects and structures within images or videos, even in large and diverse datasets.
Labellerr's segmentation capabilities can ensure the accuracy and reliability of labeled data, enhancing the performance of machine learning models trained on these datasets.
Cost Savings
Labellerr offers significant cost savings for labeling large datasets by streamlining the annotation process and minimizing manual labor costs.
With its advanced automation features, Labellerr reduces the need for extensive human intervention, allowing organizations to annotate large volumes of data more efficiently and cost-effectively.
Custom Workflows
Labellerr supports customizable workflows tailored to the specific requirements of labeling large datasets.
Organizations can define their annotation protocols, designate labeling criteria, and customize workflows to suit their unique needs.
This flexibility ensures that annotations are consistent, standardized, and aligned with the objectives of the project, ultimately leading to more reliable and accurate labeled datasets.
Active Learning Based Labeling
Labellerr leverages active learning techniques to optimize the labeling process for large datasets.
It iteratively selects the most informative samples for annotation which maximizes the efficiency of data labeling and reduces manual effort while improving the performance of trained models.
This active learning-based approach allows organizations to prioritize labeling efforts on data points that are most beneficial for model training, enhancing the overall accuracy and effectiveness of the labeled dataset.
Automated Import and Export of Data
Labellerr streamlines the process of importing and exporting data for large dataset labeling tasks with its automated functionalities.
Organizations can effortlessly upload large volumes of data into the platform and export annotated data for compatibility with various analysis tools and frameworks.
By automating data import and export processes, Labellerr ensures seamless integration with existing workflows, maximizing workflow efficiency and productivity.
Collaborative Annotation Pipeline
Labellerr fosters collaboration among annotators with its collaborative annotation pipeline.
Multiple users can work simultaneously on labeling large datasets, allowing for distributed workflows and real-time collaboration.
This collaborative approach enhances productivity and accelerates dataset annotation, enabling organizations to label large volumes of data more efficiently and accurately.
Automated QA (Quality Assurance)
Labellerr integrates automated quality assurance mechanisms to ensure the accuracy and reliability of labeled datasets.
Advanced algorithms analyze annotations in real-time, flagging inconsistencies or errors for review by annotators.
By automating quality assurance processes, Labellerr enhances the overall reliability of labeled datasets, reducing the risk of errors and ensuring the high quality of annotated data for downstream tasks.
Conclusion
In conclusion, Labellerr presents a transformative solution for advancing human face emotion detection through precise and efficient annotation.
By addressing the challenges associated with annotating emotional expressions in facial images, Labellerr allows researchers and developers to create accurate and reliable datasets essential for training robust emotion recognition models.
With its intuitive interface, robust segmentation features, and advanced automation capabilities, Labellerr streamlines the annotation process, enhances collaboration among annotators, and ensures the quality and consistency of labeled data.
Frequently Asked Questions
Q1) What is human face emotion detection, and why is it important?
Human face emotion detection is the process of identifying and categorizing emotional expressions on human faces, such as happiness, sadness, anger, and surprise. It is important for various applications, including human-computer interaction, sentiment analysis, and mental health assessment.
Q2) How does Labellerr help in annotating emotional expressions in facial images?
Labellerr provides advanced annotation tools and features specifically designed for annotating emotional expressions in facial images.
Its intuitive interface, robust segmentation features, and automation capabilities streamline the annotation process, making it efficient and accurate.
Book our demo with one of our product specialist
Book a Demo