How to Reduce Data Annotation Costs Without Compromising Quality
Reducing data annotation costs while maintaining quality requires AI-assisted labeling, inter-annotator agreement metrics, and automated quality control. Ensuring high-quality labeled data improves AI model performance for applications like autonomous vehicles, facial recognition, and NLP.
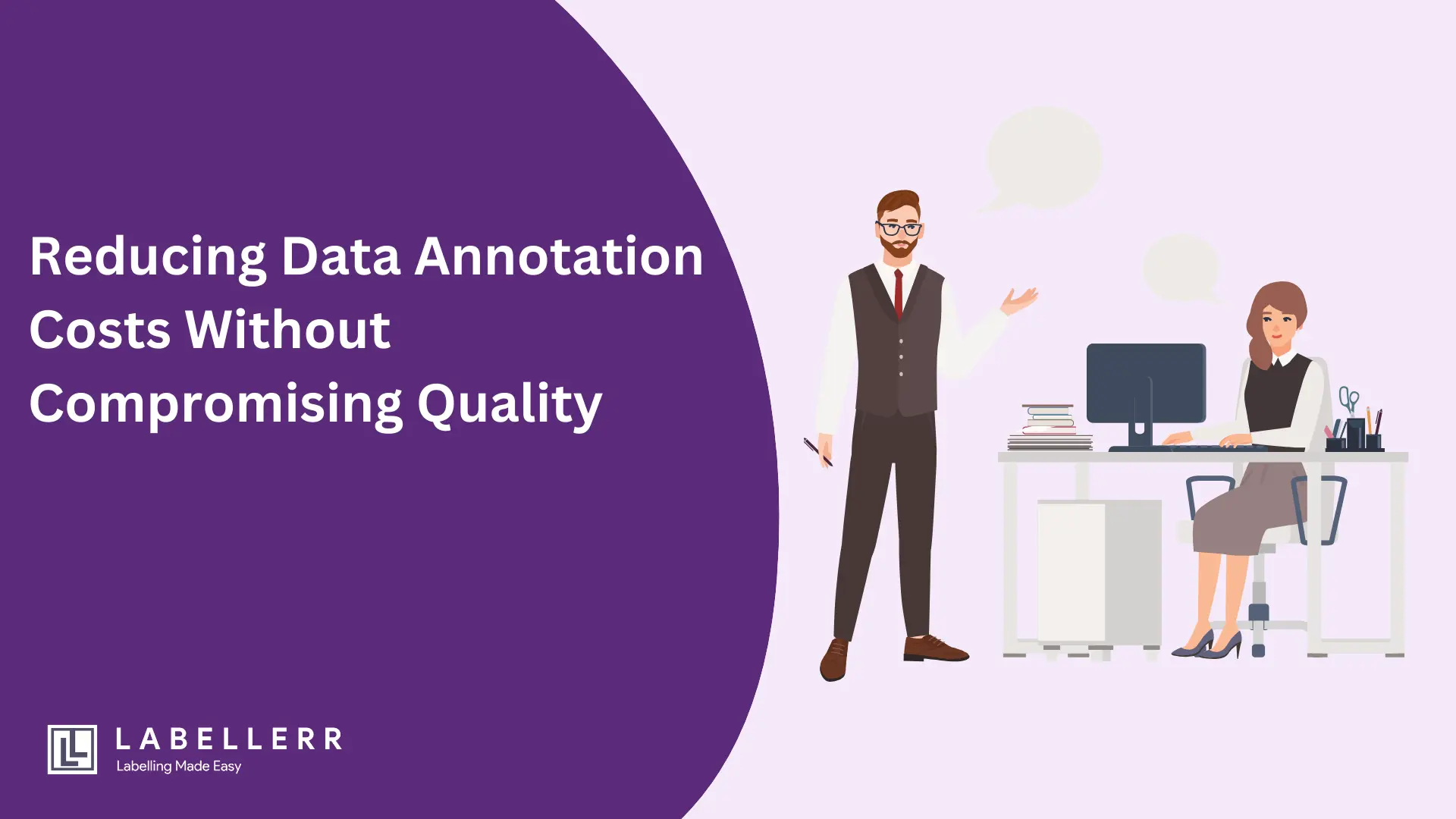
Data annotation is a crucial step in training AI models, but it often comes with high costs. Businesses spend significant amounts on manual labeling, hiring skilled annotators, and ensuring high-quality labeled data.
While high costs may seem unavoidable, there are ways to optimize expenses without sacrificing accuracy.
By using AI-assisted pre-labeling, outsourcing, and leveraging open-source tools, businesses can save money while maintaining reliable annotations.
This article explores different strategies to reduce data annotation costs while ensuring high-quality AI training data. It also discusses the trade-offs between free and paid annotation software, helping businesses choose the best option for their needs.
Why Is Data Annotation Expensive?
One of the main reasons data annotation is costly is the amount of human effort required. Skilled annotators must manually review and label vast amounts of data, which increases labor costs.
Large-scale AI projects, such as autonomous driving or medical imaging, require highly accurate labels, further driving up costs. Additionally, the complexity of datasets plays a role in expenses.
Some industries, like healthcare and finance, demand extra precision and compliance with strict regulations, making the annotation process even more expensive.
Another factor contributing to the cost is the time-consuming nature of data labeling. Manually labeling thousands or even millions of images, text samples, or audio files takes months, delaying AI model development.
This slows down the time-to-market for AI products and increases overall operational costs.
Maintaining data quality and validation also adds to the expense. Poorly labeled data can lead to inaccurate AI predictions, requiring costly rework.
Companies must implement quality control mechanisms, such as multiple rounds of review or AI-assisted verification, which increases costs further.
Additionally, securing infrastructure and data privacy is essential, especially for businesses handling sensitive information. Platforms that ensure compliance with GDPR, HIPAA, or CCPA have higher operational expenses, adding to the overall cost.
Strategies to Reduce Data Annotation Costs
Use AI-Assisted Pre-Labeling
One of the most effective ways to reduce annotation costs is by automating part of the labeling process.
AI-assisted pre-labeling allows machine learning models to generate initial annotations, which human reviewers then refine. This significantly reduces the time and effort required for manual labeling.
AI-assisted tools can pre-label simple objects, leaving only complex cases for human annotators. This approach not only lowers labor costs but also increases efficiency.
Many annotation platforms, like Labellerr, offer AI-powered automation to speed up the process while maintaining high accuracy.
Outsource Annotation to Cost-Effective Services
Instead of maintaining an in-house annotation team, companies can outsource labeling tasks to specialized annotation service providers.
Outsourcing is often more affordable than hiring full-time annotators, especially when working with large datasets. Many third-party services offer pay-per-label pricing models, allowing businesses to scale their annotation efforts based on demand.
Outsourcing also helps companies avoid additional costs related to infrastructure, employee benefits, and training. By working with an experienced annotation provider, businesses can achieve high-quality results at a fraction of the cost.
Leveraging Open-Source Annotation Tools
Using open-source tools is another effective way to cut costs. Many high-quality tools are available for free, eliminating the need for expensive software licenses.
Open-source platforms like CVAT, LabelMe, and VIA provide robust annotation features for images, videos, and text data. However, businesses must consider whether they have the technical expertise to set up and manage these tools.
Unlike paid tools, open-source solutions may require manual installation, customization, and maintenance, which could introduce hidden costs.
Automate Quality Control to Reduce Rework
Data quality plays a significant role in reducing annotation costs. If labels are incorrect or inconsistent, businesses must spend additional time and resources correcting them.
Using AI-powered quality control mechanisms can help identify errors early in the process, preventing costly rework. Features such as confidence scoring, anomaly detection, and inter-annotator agreement ensure data accuracy without requiring excessive manual review.
Automated quality checks allow businesses to minimize human involvement in reviewing already accurate annotations, cutting labor costs.
Optimize Annotation Workflows for Efficiency
Efficient annotation workflows can streamline the entire process, reducing the time and costs involved. One way to achieve this is through batch processing, where similar data points are grouped together for annotation.
This approach helps annotators work more quickly by focusing on repetitive patterns rather than switching between different types of data.
Another optimization strategy is parallel annotation, where multiple annotators work on different parts of a dataset simultaneously. This speeds up the labeling process without increasing costs significantly.
Companies can also define annotation guidelines and templates in advance, ensuring that annotators follow consistent labeling practices. This reduces the need for multiple rounds of corrections, saving both time and money.
Focus on High-Value Data First
Not all data needs the same level of annotation detail. Businesses should focus on high-impact data points that contribute the most to AI model accuracy.
Using active learning, companies can let AI models select the most valuable data for human annotation. Instead of labeling an entire dataset, businesses can prioritize critical data points that improve model performance.
This approach reduces the overall number of annotations required, cutting costs while maintaining high accuracy.
Train In-House Teams for Basic Labeling
For companies handling large datasets, training internal employees to manage basic annotation tasks can reduce expenses.
Instead of outsourcing everything, businesses can create an in-house team that handles simple labels while leaving complex annotations to external experts.
This strategy is especially useful for long-term AI projects where continuous annotation is required.
Free vs. Paid Tools
Free Tool (Open-Source Solutions)
Open-source annotation tools provide cost savings but come with technical challenges. They require setup, maintenance, and customization, which can be difficult for businesses without dedicated developers.
While they eliminate licensing costs, companies may still need to invest in infrastructure and training to use them effectively.
Paid Tool
Paid annotation tools offer automation, AI-assisted labeling, and cloud storage, making them more efficient than free alternatives.
These platforms are designed for scalability, providing features like collaborative workflows, quality control, and integration with ML pipelines. However, they come with subscription fees or pay-per-label pricing models.
Businesses must evaluate their needs to determine if the benefits of paid tools outweigh the costs.
Finding the Right Balance
Many companies use a hybrid approach, combining free and paid tools to balance cost and efficiency. Open-source tools can handle basic annotations, while paid platforms help with complex and large-scale projects.
This strategy allows businesses to control expenses while benefiting from automation and advanced features.
Conclusion
Reducing data annotation costs does not mean compromising quality. By using AI-assisted pre-labeling, outsourcing, automation, and open-source tools, businesses can lower expenses while ensuring high-quality training data.
Efficient workflows, automated quality control, and focusing on high-value data help maximize accuracy while cutting costs.
Looking for a cost-effective annotation solution?
Try Labellerr for AI-powered, scalable, and high-quality data annotation!
FAQs
Which technique is used to ensure the reliability of annotations?
Data annotation accuracy is measured using inter-annotator agreement metrics like Cohen's kappa, Fleiss' kappa, Krippendorff's alpha, and the F1 score to assess consistency.
How to evaluate the quality of annotated data?
Quality is assessed using key metrics such as Precision, Recall, F1-Score, Inter-Annotator Agreement, and Accuracy. These metrics help ensure continuous improvement in text annotation.
What is the key benefit of high-quality annotated data?
High-quality annotated data ensures AI models can accurately classify and recognize objects, crucial for applications like facial recognition, autonomous vehicles, and ADAS.
Book our demo with one of our product specialist
Book a Demo