How Industries Are Using Automated Labeling in 2025
Discover how industries like healthcare, autonomous vehicles, and retail are using automated labeling in 2025. AI-powered annotation speeds up data processing, enhances model accuracy, and improves efficiency in various sectors, enabling smarter automation and better decision-making.
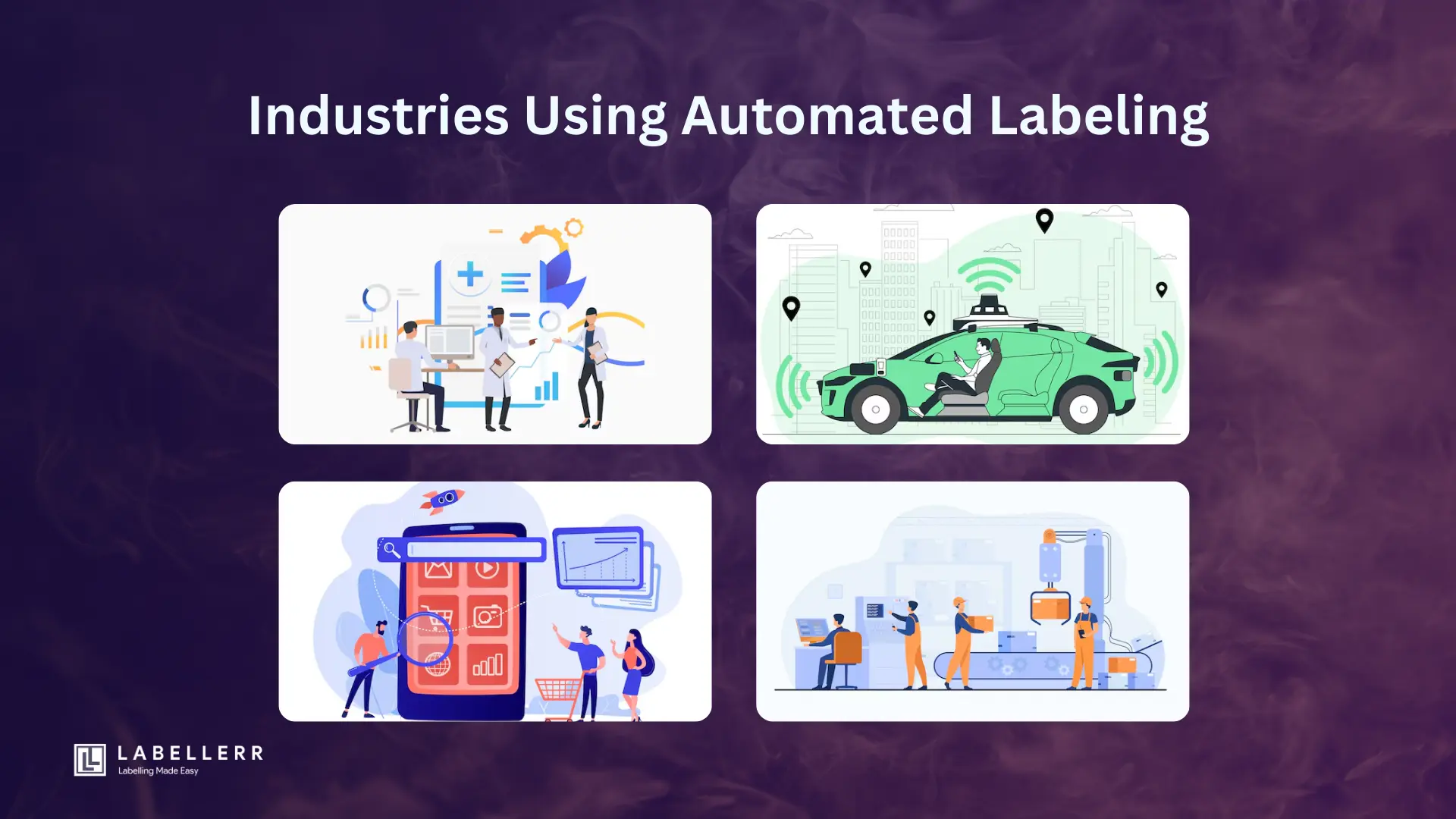
Automated labeling is transforming how industries handle data annotation. Studies show that AI-powered automation in data labeling can reduce annotation time by up to 70%, significantly accelerating AI model development.
As businesses generate vast amounts of unstructured data, automation has become essential for making AI models more efficient and accurate.
Different industries, such as healthcare, retail, autonomous vehicles, and finance, are adopting it to speed up data processing and improve AI model accuracy.
It reduces human effort, lowers costs, and ensures consistent high-quality annotations. By leveraging AI and machine learning, businesses can scale their AI solutions without being limited by manual data labeling constraints.
With the growing demand for AI across industries, the need for accurate and efficient data labeling is higher than ever.
This article explores how different industries use it and how it enhances AI model performance, reduces costs, and drives innovation.
Table of Contents
- Industry Use Cases
- Challenges in Implementation Across Industries
- Industry-Specific Future Trends
- Conclusion
- FAQs
Industry Use Cases
Healthcare
It is transforming healthcare by enabling AI models to analyze medical images with greater accuracy.
AI-powered annotation tools help segment MRI, CT scans, and X-rays, allowing doctors to detect diseases like cancer, fractures, and organ abnormalities faster.
In drug discovery, labeled datasets assist AI models in identifying potential drug compounds and predicting treatment outcomes.
It also improves patient monitoring by analyzing real-time health data, helping doctors make better clinical decisions.
Retail and E-Commerce
Retailers and e-commerce platforms use it to improve product categorization, enhancing recommendation engines and search accuracy.
AI-powered visual search allows customers to find products using images instead of text. It also helps businesses track inventory by tagging product images, reducing errors in stock management.
Additionally, AI-driven sentiment analysis of customer reviews helps brands understand consumer preferences and improve marketing strategies.
Autonomous Vehicles
Self-driving cars rely on massive amounts of labeled data to navigate safely. It helps identify objects, such as pedestrians, vehicles, and road signs, for AI-powered object detection.
Scene segmentation allows AI to differentiate between roads, sidewalks, and obstacles. Lane detection models use labeled images to guide autonomous navigation, while pedestrian recognition helps improve safety.
Real-time data labeling continuously updates AI models, making self-driving technology more reliable.
Agriculture
AI-powered it helps farmers monitor crops, detect diseases, and predict yields. Labeled satellite and drone images help identify areas affected by pests or environmental stress.
Precision farming benefits from it, which helps track soil health and optimize irrigation.
AI also supports livestock monitoring by analyzing images and videos to track animal movement, detect illnesses, and improve farm management.
Manufacturing and Robotics
Manufacturers use it to detect defects in products and ensure quality control. AI-powered defect detection helps reduce waste and improve efficiency on assembly lines.
In robotics, it enhances AI-driven robotic arms, enabling them to perform complex tasks like sorting, picking, and assembling components.
AI-assisted predictive maintenance uses labeled data to analyze machine performance and prevent equipment failures before they happen.
Finance and Banking
The financial sector leverages it for fraud detection, risk assessment, and document processing. AI models analyze labeled transaction data to identify suspicious activities and prevent financial fraud.
Labeled financial records help automate loan approvals, credit scoring, and investment risk analysis. Additionally, AI-powered text classification enables faster document processing, reducing manual work and improving operational efficiency.
Challenges in Implementation Across Industries
Industry-Specific Complexities in Data Annotation
Different industries have unique challenges in data labeling. In healthcare, AI models require highly precise annotations for medical imaging, and errors can lead to misdiagnosis.
In autonomous vehicles, real-time data labeling must handle dynamic environments with unpredictable elements like pedestrians and road signs. Each industry requires tailored labeling techniques, making automation more complex.
Balancing Automation with Human Oversight
While it speeds up annotation, human oversight is necessary to ensure accuracy.
AI-powered annotation tools may struggle with ambiguous or low-quality data, requiring human experts to verify labels.
For example, in finance, mislabeled transaction data could lead to false fraud alerts, and in manufacturing, incorrect defect labeling could cause production issues.
A hybrid approach combining AI with human review ensures high-quality annotations.
Data Security and Privacy Concerns
Many industries handle sensitive data that requires strict security measures. In healthcare, patient data must comply with regulations like HIPAA, while in finance, transaction records must meet GDPR and other security standards.
Tools must protect data privacy by implementing encryption, access controls, and secure storage. Companies must also ensure compliance with regional and industry-specific regulations when using AI-driven annotation tools.
Despite these challenges, it continues to improve AI models across industries. By addressing complexity, human oversight, and security concerns, businesses can fully leverage automation for accurate and scalable data annotation.
Industry-Specific Future Trends
AI-Powered Quality Assurance and Real-Time Annotation
AI is improving the accuracy and reliability of it through advanced quality assurance techniques.
Real-time annotation is becoming more common, especially in industries like autonomous driving and security surveillance, where AI models must process and label data instantly.
AI-powered quality control helps detect labeling errors, reduce bias, and ensure consistency across datasets.
Integration of Synthetic Data for Improved Model Performance
Industries are increasingly using synthetic data to train AI models when real-world labeled data is scarce or expensive to obtain.
In healthcare, synthetic medical images help AI models learn without exposing sensitive patient data. In autonomous vehicles, AI uses simulated driving environments to improve object detection.
By combining synthetic and real-world labeled data, companies can enhance AI model performance while reducing costs.
Role in Edge Computing and IoT Applications
As edge computing and IoT applications grow, it is becoming essential for real-time decision-making in smart devices.
In manufacturing, AI-powered sensors label and analyze production data instantly, improving efficiency. In agriculture, drones use real-time labeling to monitor crop health and detect pests.
As industries adopt AI-driven automation at the edge, it will play a key role in delivering faster and more accurate insights.
These future trends show how it is evolving to meet the growing demands of AI-driven industries. By integrating real-time AI quality assurance, synthetic data, and edge computing, businesses can unlock new possibilities for AI innovation.
Conclusion
Automated labeling is transforming industries by making AI model development faster, more accurate, and scalable.
Sectors like healthcare, retail, autonomous vehicles, agriculture, manufacturing, and finance rely on it to improve efficiency, reduce costs, and enhance decision-making.
By reducing manual effort and ensuring high-quality annotations, businesses can train AI models faster and deploy them with greater accuracy.
As AI continues to evolve, automation in data annotation will become even more critical. Companies that adopt it will stay ahead in innovation and gain a competitive edge in their industries.
If you're looking to streamline your AI data annotation process, explore Labellerr’s Auto Label solutions to enhance accuracy, scalability, and efficiency for your business.
FAQs
Which industries are using automated labeling in 2025?
Industries like healthcare, autonomous vehicles, retail, finance, and manufacturing benefit from it, improving AI model accuracy, efficiency, and scalability.
How is automated labeling is used in the healthcare industry?
It speeds up medical image annotation, enabling faster disease detection, diagnosis, and treatment planning with AI-assisted analysis of X-rays, MRIs, and CT scans.
What role does automated labeling is being used in autonomous vehicles?
It helps self-driving cars recognize road signs, detect pedestrians, and segment lanes by efficiently annotating vast amounts of image and sensor data, enhancing safety and accuracy.
Book our demo with one of our product specialist
Book a Demo