Image Annotation Basics: Types, Importance and Applications?
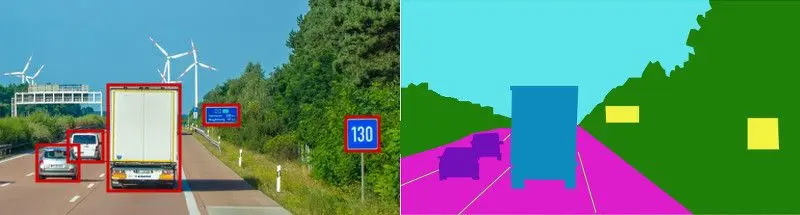
Computer engineers are becoming increasingly interested in artificial intelligence (AI) and machine learning (ML), which they can use to expand the use of this cutting-edge technology into untapped industries or enhance the effectiveness and performance of the current fields.
The availability of training data for machine learning is a fundamental requirement for enhancing AI performance. While image annotation is a method used to produce training data for a visual perception model built using AI and ML principles.
Introduction to Image Annotation
Data Annotation that focuses on recognizing and labeling certain details in images is known as an Image annotation. Image annotation in computer vision is adding labels to unprocessed data, including photos and videos. An associated object class with the data is represented by each tag.
Labels are used by supervised machine learning algorithms to identify a particular object class in unstructured data. It enables these models to give meaning to the data, which aids in model training.
Data sets for computer vision algorithms are produced using Image annotation and are divided into training and test/validation sets. The training set is used to train the model initially, while the test/validation set is used to assess the model's performance.
The dataset is used by data scientists to train and test their models, after which the models may automatically categorize hidden unlabeled data.
To further explore uncharted areas where AI is required, you must first comprehend the significance of picture annotation in AI and ML.
Types of Image Annotation: An overview
Images used as training data for AI applications can be presented in various ways through data annotation techniques. Cogito's experts specialize in a range of picture annotation methods, including:
- 2D Bounding Boxes: These boxes help with attribute calculation for computer vision models and real-world environment recognition.
- 3D Cuboid Annotation: It aids in determining the depth of objects in 3D simulated reality, like vehicles and buildings.
- Key Point Annotation: Connecting dots to identify facial expressions, postures, and emotions.
- Splines and Lines: Drawing lines to outline specific areas in photographs for boundary recognition.
- Text Annotation: Applying labels to identify identities, emotions, and intentions based on industrial or commercial criteria.
- Polygon Annotation: Precise annotations for images with irregular dimensions, such as traffic and overhead views.
- Semantic Segmentation: Segmenting images for accurate recognition and understanding at the pixel level.
- 3D Point Cloud Annotation: Visual interpretation of objects to improve recognition, categorization, and understanding of their dimensions.
Why Image Annotation is Crucial for AI and ML
Image annotation serves as the fundamental prerequisite for numerous commercial Artificial Intelligence (AI) products available in the market and constitutes a pivotal component in the realm of Computer Vision (CV).
It plays an indispensable role in deploying AI across a wide spectrum of business applications, such as automating the analysis of images related to vehicle accidents in the insurance industry, identifying vehicles and pedestrians for autonomous transportation, and aiding medical professionals in the detection of cancers within medical imagery.
In practical terms, image annotation involves the technical process of attaching descriptive labels to individual images or a sequence of images. This procedure finds application in various Machine Learning tasks, encompassing image classification, object detection, and image segmentation.
The nature of the labeling task can vary significantly based on the specific objectives and constraints of the model, ranging from assigning a single label to an entire image to assigning multiple labels to distinct pixel clusters within it.
Potential Areas for Image Annotation in Machine Learning
1. Detect objects of interest in the image
In machine learning or AI, you must teach the computer to recognize the numerous kinds of objects that can be seen in the outside world. Such things cannot be detected by autonomous vehicles, robotics, or flying machines unless they have been educated to do so. Machines can recognize objects of interest from annotated photos.
To help computer vision software recognize various types of objects, bounding box image annotation is a technique utilized. It can be used to create AI-enabled models for the retail, automotive, and a variety of other industries.
2. Classify the various objects in the image
Not only is object detection the goal of picture annotation, but object classification also accomplishes the same thing. It is actually conceivable for an image to contain multiple different categories of objects, making it impossible for a machine to categorize them.
If a dog and a man are present in the same photograph, for instance, both must be identified as distinct things when presented to AI models in real-world settings. Therefore, the technique utilized to categorize such things is image annotation. However, computer vision is employed to train the AI models and uses the image annotation.
3. Recognize various object classes
Comparable to this, because of similar dimensions, it is challenging to distinguish between different types of objects in a single image. In these situations, both object recognition and classification are necessary at the ground level.
And the best method for classifying objects into a single class and making it simpler for machines to distinguish between different kinds of objects is semantic segmentation. This method allows objects to be defined with nested classifications for computer vision, which is also highly useful for comparable attributes.
4. Supervised machine learning training
An additional benefit of image annotation is that it facilitates the creation of label data sets for AI and machine learning. AI and machine learning: Its history to its future: read here
Annotated pictures must enable computers to quickly and accurately discover and classify these objects for supervised machine learning.
There are two different types of algorithms utilised in supervised machine learning. Its initial classification aids in placing it in the appropriate category. Second, regression, which uses historical data to forecast a value.
5. Used as training data and model validation
The ability to assess AI or ML models while they are being developed and put to the test for their accuracy is one of the most significant goals of picture annotation. In order to determine whether a model is capable of accurately detecting, recognizing, classifying, and predicting objects, annotated images are used.
The skilled engineers and annotators validate the machine learning model during this procedure. The model won't be able to detect the objects if annotated photographs aren't available, and this method also aids in assessing the caliber of data labeling services.
The algorithm won't be able to relate photos from its database or past experience gathered from machine learning if they are not correctly labelled.
As a result, image annotation is important for the development of AI and machine learning. To make sure your model is receiving the proper training, you need also take into account the quality of the machine learning training data.
Addressing Key Challenges in Machine Learning Image Annotation?
Although image annotation offers numerous advantages, it also presents several significant obstacles that ML engineers and data science teams must contend with.
Opting for the Right Annotation Tools
To enable machine learning algorithms to identify elements within digital images akin to human perception, organizations need to make informed decisions about which data attributes to use for labeling. This necessitates the careful selection of digital annotation tools, along with the cultivation of a workforce capable of utilizing these tools effectively.
Balancing Automated and Human Annotation
The choice between employing human annotators or automated tools in image annotation is a crucial decision. While human involvement may extend the time and incur additional expenses associated with hiring skilled personnel, computerized annotation tools offer a superior level of precision and consistency.
Ensuring Data Output Quality
The success of machine learning models heavily relies on the quality of the data they receive. Trustworthy data quality is imperative for these models to make accurate predictions. The interpretability of subjective data by digital annotators can be challenging, especially when considering geographical variations.
Conclusion
In conclusion, the growing interest of computer engineers in artificial intelligence (AI) and machine learning (ML) is driving the expansion of these technologies into new industries and the enhancement of existing fields. Training data availability is a fundamental requirement for improving AI performance, and image annotation plays a crucial role in generating this data for visual perception models.
Image annotation, the process of labeling specific details in images, is essential for various applications in machine learning, including image classification, object detection, and image segmentation. It enables supervised machine learning and the creation of labeled datasets for training and testing machine learning models.
Image annotation is indispensable in commercial AI products, spanning industries like insurance, transportation, and healthcare. It involves the technical task of adding descriptive labels to individual images or image sequences, catering to specific model objectives, from single-image labels to detailed pixel-level annotations.
This process finds application in various areas, such as:
- Detecting objects in images to train machines to recognize and interact with the real world.
- Classifying objects within images, even when multiple categories coexist.
- Recognizing different object classes with complex attributes.
- Facilitating supervised machine learning by providing labeled datasets.
- Using annotated images for training and validating AI and ML models, ensuring their accuracy and performance.
Despite its advantages, image annotation also presents challenges, such as selecting the right annotation tools, striking a balance between human and automated annotation, and ensuring data quality, particularly in dealing with subjective data and geographical variations.
In the dynamic landscape of AI and ML, staying updated with the latest data and technology developments is vital for continued progress and success. Image annotation remains a critical element in advancing these fields, contributing to their growth and effectiveness across various domains.
Frequently Asked Questions
1. Why is Image Annotation Used in Computer Vision?
What is the function of image annotation? Within the domains of machine learning and deep learning, image annotation refers to the procedure of applying textual or tool-based labels to an image to convey the specific data attributes that you intend for your model to identify autonomously. Annotated images serve the purpose of enriching a dataset with metadata.
2. What Are the Advantages of Image Annotation?
Image and video annotation can be leveraged for various essential applications that businesses can derive significant benefits from, including:
- Effortless Object Detection
- Precise Object Tracking
- Accurate Object Localization
- Person Identification
- Enhanced Product Discovery
To keep yourself updated with the latest data and technology information, stay in touch!
Book our demo with one of our product specialist
Book a Demo