How Do Image Labeling Tools Enhance Image Annotation?
Image labeling tools enhance data annotation by automating tasks with AI-powered features, enabling accurate annotations like bounding boxes, polygons, and segmentation masks. They are customizable, user-friendly, and widely used in industries such as healthcare, agriculture, and autonomous driving.
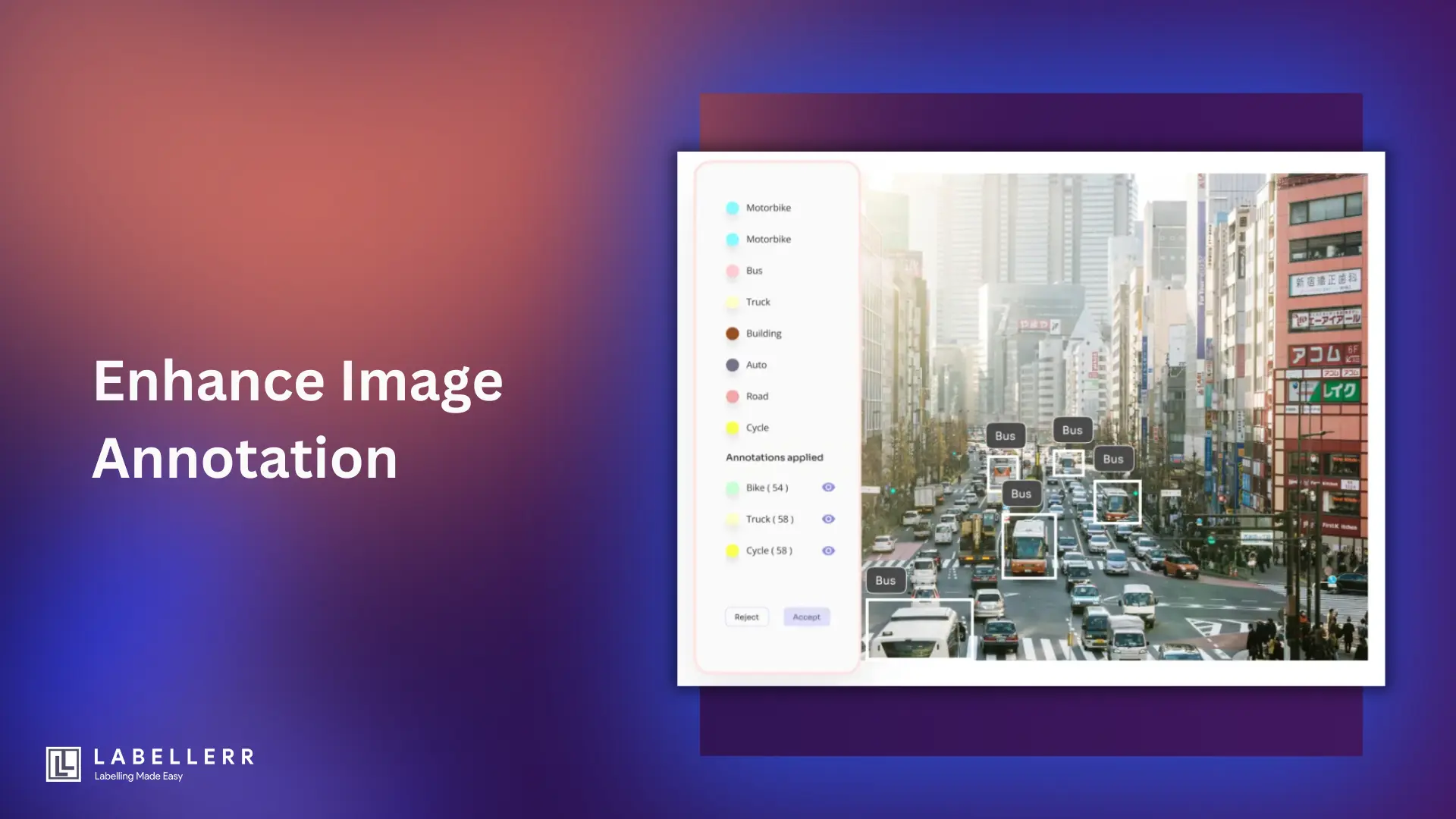
Did you know that high-quality labeled data accounts for 70-80% of the success of AI models? This fact shows how critical accurate image annotation is for training reliable and efficient AI systems.
As AI and machine learning continue to grow in 2025, the demand for precise and scalable image annotation has become more important than ever.
However, manual image annotation comes with significant challenges. It is time-consuming, prone to errors, and difficult to scale for large datasets. These issues often delay AI projects and impact the quality of the final model.
This is where image labeling tools are making a difference. These tools are transforming image annotation by automating repetitive tasks, improving accuracy, and enabling scalability. They are helping AI teams create high-quality datasets faster and more efficiently, paving the way for better-performing AI models.
But what exactly are image labeling tools lets discuss.
Table of Contents
- What Are Image Labeling Tools?
- Challenges in Manual Image Annotation
- How Image Labeling Tools Enhance Image Annotation
- Conclusion
- FAQs
What Are Image Labeling Tools?
Image labeling tools are software platforms that help you tag or label objects in images to create structured datasets. These datasets are essential for training AI and machine learning models, especially in tasks like object detection, image classification, and segmentation.
The primary function of these tools is to make the image annotation process faster and more accurate. They allow you to label images by drawing bounding boxes, polygons, or other shapes around objects. The labeled data tells AI models what to look for, helping them learn and make predictions effectively.
The basic workflow of image labeling tools includes:
- Uploading Images: Start by uploading raw images or datasets to the platform.
- Labeling Objects: Use the tool to tag or annotate objects in the images with specific labels.
- Exporting Datasets: Export the labeled images in a format that works with your machine learning pipeline, like COCO or YOLO.
Many modern image labeling tools offer advanced features like AI-assisted labeling and automation. These features use pre-trained models or machine learning algorithms to suggest labels, significantly reducing manual effort.
Some tools also include quality assurance mechanisms, cloud integration, and collaboration options, making them ideal for both small projects and large-scale AI development.
Challenges in Manual Image Annotation
Manual image annotation has several challenges that slow down AI development and increase project costs. Let’s look at the most common problems:
1. Time-Intensive Process
Manually labeling images takes a lot of time, especially when working with large datasets. Annotators need to go through each image, tag objects, and ensure accuracy, which can delay AI projects. This slow process makes it difficult to meet tight deadlines or launch products quickly.
2. High Costs
Hiring and managing a large team of annotators can become expensive, especially for startups or smaller companies. The cost includes not just salaries but also the resources needed to train teams and maintain high-quality standards. For organizations with limited budgets, manual annotation can be a significant financial burden.
3. Human Errors
Humans are prone to errors, especially when handling repetitive tasks like labeling thousands of images. Fatigue, inconsistency, and subjective decisions often lead to low-quality annotations. Inaccurate data affects the performance of AI models and requires additional time and resources to fix.
4. Scalability Issues
As datasets grow in size, managing and annotating them manually becomes nearly impossible. Large datasets with millions of images require tools that can handle the workload efficiently. Without automation, scaling up AI projects to meet industry demands is extremely challenging.
How Image Labeling Tools Enhance Image Annotation
Image labeling tools are transforming image annotation by making the process faster, more accurate, and cost-effective. They offer advanced features that solve the challenges of manual annotation, enabling teams to handle larger datasets efficiently and produce high-quality labels. Here's how these tools enhance data annotation:
Efficiency Through Automation
Image labeling tools like Labellerr use automation to handle repetitive tasks, saving a significant amount of time. Instead of manually labeling every object in an image, these tools can pre-label data or suggest annotations using AI. Annotators only need to review and refine the labels, which speeds up the entire process.
For example, an AI startup reduced its annotation time by 70% using Labellerr’s automation features. This allowed them to focus on other aspects of their project, like model development and deployment. By automating routine tasks, these tools help teams meet tight deadlines and launch products faster.
Improved Accuracy and Consistency
Manual annotation is prone to human errors like inconsistency or fatigue. Image labeling tools address these problems by incorporating AI-assisted features and quality control mechanisms.
For instance, these tools use confidence scores to indicate how certain the system is about a label. Low-confidence labels can be flagged for human review, ensuring better accuracy.
Additionally, features like anomaly detection help identify incorrect or inconsistent labels, further reducing errors. With these built-in checks, image labeling tools ensure that datasets remain consistent, which is critical for training reliable AI models.
This level of accuracy is especially important in industries like healthcare or autonomous vehicles, where errors can have significant consequences.
Scalability for Large Datasets
Handling large datasets with thousands or even millions of images is a significant challenge for manual annotation. Image labeling tools are built to scale and can process massive datasets efficiently.
Many of these tools are cloud-based, which means they integrate seamlessly with machine learning pipelines and cloud storage platforms like AWS or Google Drive.
This integration allows teams to upload, annotate, and export large datasets without interruptions. For example, an organization working on autonomous vehicles can label road scenes at scale, enabling faster training and testing of their AI models. With these tools, scalability is no longer a bottleneck for growing AI projects.
Cost-Effectiveness
One of the biggest advantages of image labeling tools is their ability to reduce labor costs. Manual annotation often requires hiring large teams, which can take up a significant portion of an AI project’s budget.
Automating most of the annotation process reduces the need for extensive human involvement, saving both time and money.
For instance, manual annotation can account for up to 25% of a project’s total cost. By using automation, companies can cut these costs by nearly half. The savings can then be redirected to other critical areas like model improvement or deployment.
Image labeling tools make high-quality annotation more affordable, especially for startups and smaller organizations.
By automating tasks, improving accuracy, enabling scalability, and reducing costs, image labeling tools like Labellerr are revolutionizing image annotation. These tools not only streamline the process but also ensure that AI projects are completed on time and within budget.
Whether for healthcare, retail, or autonomous vehicles, these tools are essential for creating high-quality datasets that drive AI success.
Conclusion
Image labeling tools are essential for improving image annotation, which is the backbone of AI and machine learning. These tools save time, reduce errors, and make it easier to scale projects.
By automating repetitive tasks and offering advanced features like AI-assisted labeling and quality checks, tools like Labellerr ensure consistent and accurate datasets. This improves the performance of AI models and helps teams focus on delivering impactful results.
Manual annotation is time-consuming, costly, and prone to human errors, but image labeling tools solve these challenges effectively. They enable businesses to handle large datasets efficiently and reduce the overall cost of AI development.
Whether it’s healthcare, retail, or autonomous vehicles, these tools are transforming how data is prepared for machine learning.
If you’re ready to streamline your image annotation workflows, start exploring our tool today. It is designed to make the annotation process faster, more reliable, and cost-effective.
Visit our website or check out our guides to learn how Labellerr can help you enhance your AI projects and achieve success.
FAQs
What are image labeling tools?
Image labeling tools are software applications used to annotate images with tags, labels, or bounding boxes, creating datasets for machine learning.
How do AI-powered image labeling tools improve accuracy?
AI-powered tools suggest annotations automatically, reducing manual effort and ensuring higher consistency and precision in labeled data.
Can image labeling tools handle complex annotations?
Yes, many tools support advanced annotations like semantic segmentation, polygons, 3D bounding boxes, and keypoint detection.
Are image labeling tools customizable for specific projects?
Most tools allow customization for project-specific needs, such as unique label sets, annotation formats, and automated workflows.
What industries benefit from image labeling tools?
Industries like autonomous driving, healthcare, retail, agriculture, and surveillance rely on these tools for AI model training and deployment.
Book our demo with one of our product specialist
Book a Demo