How an AI-powered smart city will manage its traffic in future
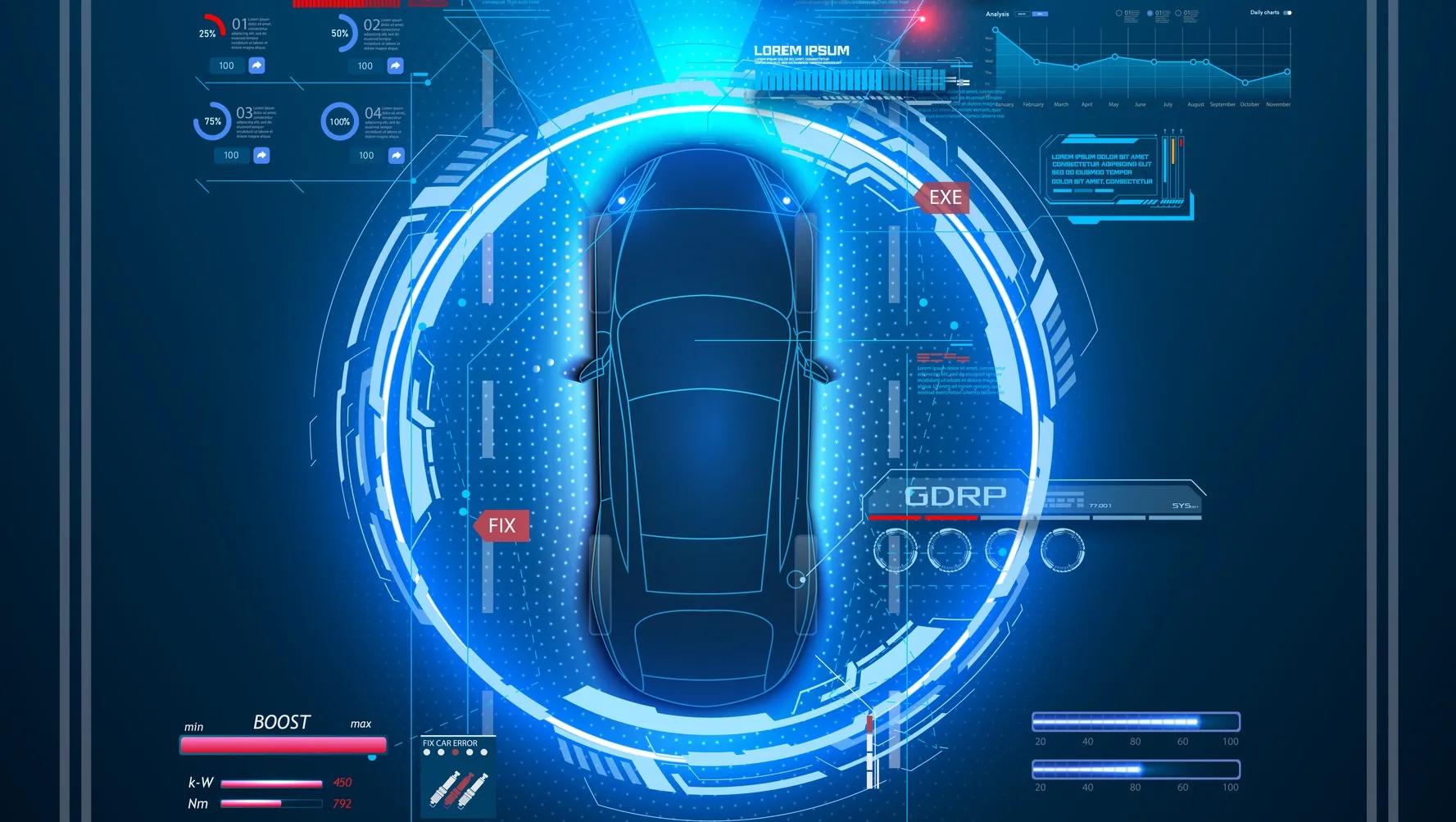
Vehicle “Idling” refers to running a vehicle’s engine when it’s not moving, such as when you’re at a red light or stuck in traffic. Idling is part of the process of driving a car and is a fairly common occurrence for most drivers. However, idling may not be the best thing for your car, fuel consumption, or the environment.
Reducing the amount of time vehicles are idling is a simple way to maintain and even increase fuel economy among vehicles. Idling also contributes to pollution and shortens engine lifespan.
Img: Signalized Intersection
No one likes sitting at a red light. But signalized intersections aren't just a minor nuisance for drivers; vehicles consume fuel and emit greenhouse gases while waiting for the light to change. There is ongoing research around eco-driving control strategies to reduce fuel consumption and emission levels at intersections. However, methods to devise effective control strategies across a variety of traffic settings remain elusive.
The issue may be resolved if motorists could time their trips so they arrive at the intersection when the light is green. This could be achieved by an autonomous vehicle that uses artificial intelligence to control its speed.
In a new study, MIT researchers demonstrate a machine-learning approach that can learn to control a fleet of autonomous vehicles as they approach and travel through a signalized intersection in a way that keeps traffic flowing smoothly. Using simulations, they found that their approach reduces fuel consumption upto 18% and emissions upto 25% while improving average vehicle speed by upto 20%. The technique gets the best results if all cars on the road are autonomous, but even if only 25% use their control algorithm, it still leads to around 50% fuel and emission reduction benefits.
Img: Eco-Driving Strategy at Signalized Intersection
· Problem :- Intersections can present billions of different scenarios depending on the number of lanes, how the signals operate, the number of vehicles and their speeds, the presence of pedestrians and cyclists, etc. Typical approaches for tackling intersection control problems use mathematical models to solve one simple, ideal intersection but they don't hold up in the real world.
· Solution :- The team approached the problem using a model-free technique known as deep reinforcement learning. Reinforcement learning is a trial-and-error method where the control algorithm learns to make a sequence of decisions. It is rewarded when it finds a good sequence. With deep reinforcement learning, the algorithm leverages assumptions learned by a neural network to find shortcuts to good sequences, even if there are billions of possibilities.
· Problem :- The system needs to learn a strategy that reduces fuel consumption and limits the impact on travel time. These goals can be conflicting. To reduce travel time, the car should go fast, but to reduce emissions, the car should slow down or not move at all. Thus, competing rewards can be very confusing to the learning agent.
· Solution :- The researchers employed a workaround using a technique known as reward shaping. They give the system some domain knowledge it is unable to learn on its own. In this case, they penalized the system whenever the vehicle came to a complete stop, so it would learn to avoid that action.
· Tests: Once they developed an effective control algorithm, they evaluated it using a traffic simulation platform with a single intersection. The control algorithm is applied to a fleet of connected autonomous vehicles, which can communicate with upcoming traffic lights to receive signal phase and timing information and observe their immediate surroundings. The control algorithm tells each vehicle how to accelerate and decelerate. As cars approached the crossroads, their system prevented any stop-and-go traffic. Simulations of this system outperformed a model that simulates human drivers. With this technology, fuel consumption and emissions were reduced more than with other optimization techniques.
· Tests: Down the road, the researchers want to study interaction effects between multiple intersections. They also plan to explore how different intersection set-ups (number of lanes, signals, timings, etc.) can influence travel time, emissions, and fuel consumption. In addition, they intend to study how their control system could impact safety when autonomous vehicles and human drivers share the road. While this work is still in its early stages, the team sees this approach as one that could be more feasibly implemented in the near-term.
Img: Results
· Baselines:
- V-IDM: The deterministic vanilla version of the IDM car-following model. It is a driving baseline that can produce realistic shock waves.
- N-IDM: Adds a noise sampled from a uniform distribution unif (−0.2, 0.2) to the IDM defined acceleration a. This is a driving baseline that can produce realistic shock waves and models variability in driving behaviors of humans.
- M-IDM: Developed on top of N-IDM model such that IDM parameters for each driver are sampled from respective Gaussian distributions. It represents a more diverse mix of drivers with varying levels of aggressiveness.
- Eco-CACC: A model-based trajectory optimization strategy.
Comparison of Proposed control policy with naturalistic driving and model-based control baseline :-
Img: Comparison Table
Src: https://vindulamj.github.io/eco-driving-rl/
Comparison of per vehicle fuel consumption, emission level and average speed improvement under different control strategies with 100% CAV penetration rate :-
Img: Time space diagrams of north-bound vehicle trajectories produced by a) V-IDM model, b) N-IDM model, c) M-IDM model, d) Eco-CACC model, and e) DRL model. Both Eco-CACC and DRL models demonstrate behaviors which involve reduced stopping at the intersection as compared to IDM variants. Both Eco-CACC and DRL models increase the throughput of vehicles during a green light phase by one extra vehicle as can be seen in Figure d and e. Figure f shows the speed profile of a selected vehicle under the five different control strategies.
Src: https://vindulamj.github.io/eco-driving-rl/
How well does the proposed control policy generalize to environments unseen at training time? :-
Img: Percentage improvement in terms of fuel usage, emission levels and average speed from the IDM variant baselines under different CAV penetration rates (CAVs are controlled by zero-shot transferred DRL policy).
Src: https://vindulamj.github.io/eco-driving-rl/
Google is using A.I. to reduce idling time at traffic intersections
- Poorly timed traffic lights don't just waste precious minutes, they're also bad for the environment and public health. Google has been working on a project that could use AI to make traffic lights more efficient and as a result, decrease pollution in general.
- In a “Sustainable with Google” event, the company revealed information on a research project that is using A.I. to reduce the amount of time spent sitting at traffic lights. A series of pilot projects in Israel improved the timing at intersections, enabling Google to reduce fuel consumption and intersection delays by 10% to 20%. Not only does that help local air quality, but it’s also helping folks get to work more quickly.
- Google's new project would use AI to measure and calculate traffic conditions and timing at a city's intersections, then time them more efficiently. One of the company's AI research groups has been able to accurately calculate and gather this data and train a model to optimize inefficient intersections. This has been done by calculating the relationship between traffic conditions and light timing within a reasonable level of accuracy, then building an A.I. model based on that data.
- Google has run pilots at four locations in Israel to date. Though these pilot projects were conducted in Israel, Google is looking to have another go in Rio de Janeiro and other major cities around the world. The company didn't explain how the AI would work with current systems. "It's early days but on the back of these promising results. This is one of the steps towards the vision of completely smart cities that the industry has collectively been working on for years. The idea of letting AI decide when to let vehicles stop or go can seem unsettling at best and potentially risky, but the goal of improving traffic light efficiency is a worthwhile one. Hopefully, with rigorous testing and safety measures in place, we may actually be able to reduce a significant amount of wasted fuel and exhaust-based pollution,” said a member of the team.
- The vast majority of A.I. research in the automotive sphere tends to be focused on the cars themselves, particularly in terms of autonomous driving, but applying this technology to traffic lights also has the potential to increase safety on the roads. Hopefully, smart cars will eventually be able to communicate with smart traffic lights to further improve the efficiencies at intersections around the world.
Specialized AI that Reduces Traffic and Travel Times (German Research)
- Every driver loves sailing through a series of green lights without having to stop and wait at a traffic light. There is a great necessity to speed up waiting times at intersections as conventional methods are inflexible and cannot interpret all traffic flow patterns. German researchers have found a way to reduce time spent at intersections using artificial intelligence, the KI4LSA project.
- The Technology Proposed :- The new AI method uses radar sensors and high-resolution cameras to interpret traffic patterns in real-time. They can determine the speed of traffic as well as waiting times and combine this with self-learning algorithms. Trials were conducted for the same in Lemgo, Germany. The intelligent lights are said to improve traffic flow by 10 to 15 percent. Aside from reducing commuting time, these traffic lights will reduce noise and CO2 emissions from cars idling at intersections.
Img: Testing of the technology
Src: https://www.goodnet.org/articles/specialized-ai-reduces-traffic-travel-times
- Another exciting development in this research is the K14PED project, which studies pedestrians crossing intersections. Using AI, researchers can ascertain how many people are waiting at a pedestrian crossing and whether some of these people will need extra time to cross the street. Using a 3D points cloud, they can tell if someone has disabilities or is elderly without identifying the individual. Such a needs-based system could reduce pedestrian waiting time by 30 percent and jaywalking by 25 percent.
- This technology is exciting news for pedestrians and drivers alike. It encourages safety, protects the environment.
Computer Vision system that helps keep traffic moving efficiently (ORNL)
- When cars sit in traffic or at stoplights, they waste gas. Researchers at Oak Ridge National Laboratory (ORNL) used artificial intelligence and machine learning to design a computer vision system that helps keep traffic moving efficiently through intersections and has the added benefit of minimizing fuel consumption.
- The team used three smart stoplight cameras from Gridsmart, a traffic management services company, to collect real-world data from images of vehicles as they traveled through intersections on the lab’s campus. They applied AI and ML to teach the cameras how to identify vehicle types and their estimated gas mileage and then send that data to the next intersection’s traffic light. The Gridsmart cameras have fisheye lenses, so a single camera can see horizon to horizon around an intersection. The robust and easy-to-install sensors hang in an intersection and can tell if a lane is occupied by a vehicle. They don’t, however, control the timing of the intersection lights. Because the Gridsmart cameras only supply an overhead view, rather than a ground view of vehicles, the researchers tapped a ground-based roadside sensor ORNL was using for another project so they could collect data on vehicle makes and models. They used the overhead images and ground-based data to get a dataset of vehicle classes. When combined with DOE fuel-economy estimates for different vehicle types, the ORNL Overhead Vehicle Dataset would allow them to determine when gas-guzzlers were in the intersection.
- The basic gist of it is: If you can use their imagery to detect the make and model, you have a pretty good estimate of what the vehicle’s fuel consumption is. Then the second part is using that information to control the traffic lights to save energy without costing mobility.
- Between the yearlong project’s start in February 2018 and September 2018, the dataset grew to about 12,600 vehicles spanning 474 classifications, but the team said that wasn’t enough to train a deep learning network that would automatically detect and classify vehicle types. Without the time to collect more data on their own, the researchers turned to a publicly available dataset collected by Stanford University researcher Timnit Gebru that identified 22 million cars from Google Street View images and classified them into 2,600-plus categories.
- To run a simulation of a citywide traffic grid, Karnowski and his team used an open source package called SUMO to model traffic systems. They then added the ability to train an ML algorithm to affect the lights with a detection system that was informed by their experimentation with the Gridsmart and open source data. They used an ML approach called reinforcement learning to teach the system to change the traffic lights to keep high fuel consumption vehicles moving.
- “What’s interesting about it is you basically set up a system of rewards and penalties, and then you let the computer try different things until it learns to get the rewards and minimize the penalties, like learning to win at a game. In this case, the game was training the traffic light to keep traffic moving and save energy at the same time. Although the team didn’t do a complete round of simulations, in at least one scenario they estimated 25% fuel savings in the case of large trucks moving in one direction. It learned how to preferentially treat the large trucks because they are heavy fuel consumers, without simply leaving a lane light green for longer as you don’t want to have people waiting too long. Generally speaking, it would improve traffic flow and help with the economics of transportation,” said the team.
Pittsburgh Research (SURTRAC)
· In Pittsburgh researchers are already working with city managers on a similar approach that has been operating in the city since 2012. An adaptive traffic control system developed by researchers at the Robotics Institute, Carnegie Mellon University, has been rolled out around the city by a company called Rapid Flow Tech. The programme, called SURTRAC, uses cameras and radar to analyse traffic in real time and predict its movement. This technology is being used at approximately a third of the intersections in Pittsburgh and since launching, it has reduced wait times at intersections by up to 40%, according to the company. It also claims that journey times in the city have fallen by 25% while vehicle emissions have dropped by up to 20%. The system uses video feeds to automatically detect the number of road users, including pedestrians, and types of vehicles that are at an intersection. The AI software then processes this information second by second to come up with the best way to move traffic through the intersection, changing traffic lights depending on the most optimal way of keeping traffic moving. Decisions can be made autonomously, and shared with neighbouring intersections to help them understand what is coming their way.
· Surtrac’s sensors identify approaching vehicles, calculate their speed and trajectory, and adjust a traffic signal’s timing schedule as needed. A Surtrac-equipped intersection then distributes the timing and vehicle information it collects to other nearby “smart” intersections, which can use that information, along with their own data, to adjust their own signals. That allows traffic throughout the system to be managed in real time based on the volume that’s actually there, not the volume that’s only predicted to be there. Each intersection makes decisions autonomously and communicates with the rest of the traffic signal network to make the flow of moving cars more efficient. In this case, the aim was to reduce vehicle waiting times at traffic-light intersections and optimise traffic flow.
Img: A programme called SURTRAC uses cameras and radar to analyse traffic in real time and predict its movement.
Src: https://youtu.be/mGC__zA0ZZA
· Pedestrians with disabilities could see a particular benefit from smart traffic systems like Surtrac. With intersections outfitted with cameras, motion sensors and artificial intelligence software, people in wheelchairs or using other assistive devices could be detected before they arrive at a crosswalk, their travel time could be estimated, and the timing system could make sure they have the right of way. Others could see advantages, too. “In principle, if a pedestrian is running a few seconds behind or longer, we could hold the light longer to keep a bus there,” says a member of the team. Smart traffic systems could also prove more adaptable to abnormal situations like inclement weather. Rain and snow cause significant traffic slowdowns, and pre-programmed timed traffic lights typically don’t accommodate for that. Smart traffic management systems, however, could be fed weather data, or collect it themselves, and change their timing accordingly. They could also clear the way for emergency services by accelerating certain lanes of traffic or blocking cars from entering an intersection before first responders pass.
To know more such amazing content, stay tuned with us!
Book our demo with one of our product specialist
Book a Demo