AI-Powered Grid Monitoring: A Sustainable Solutions
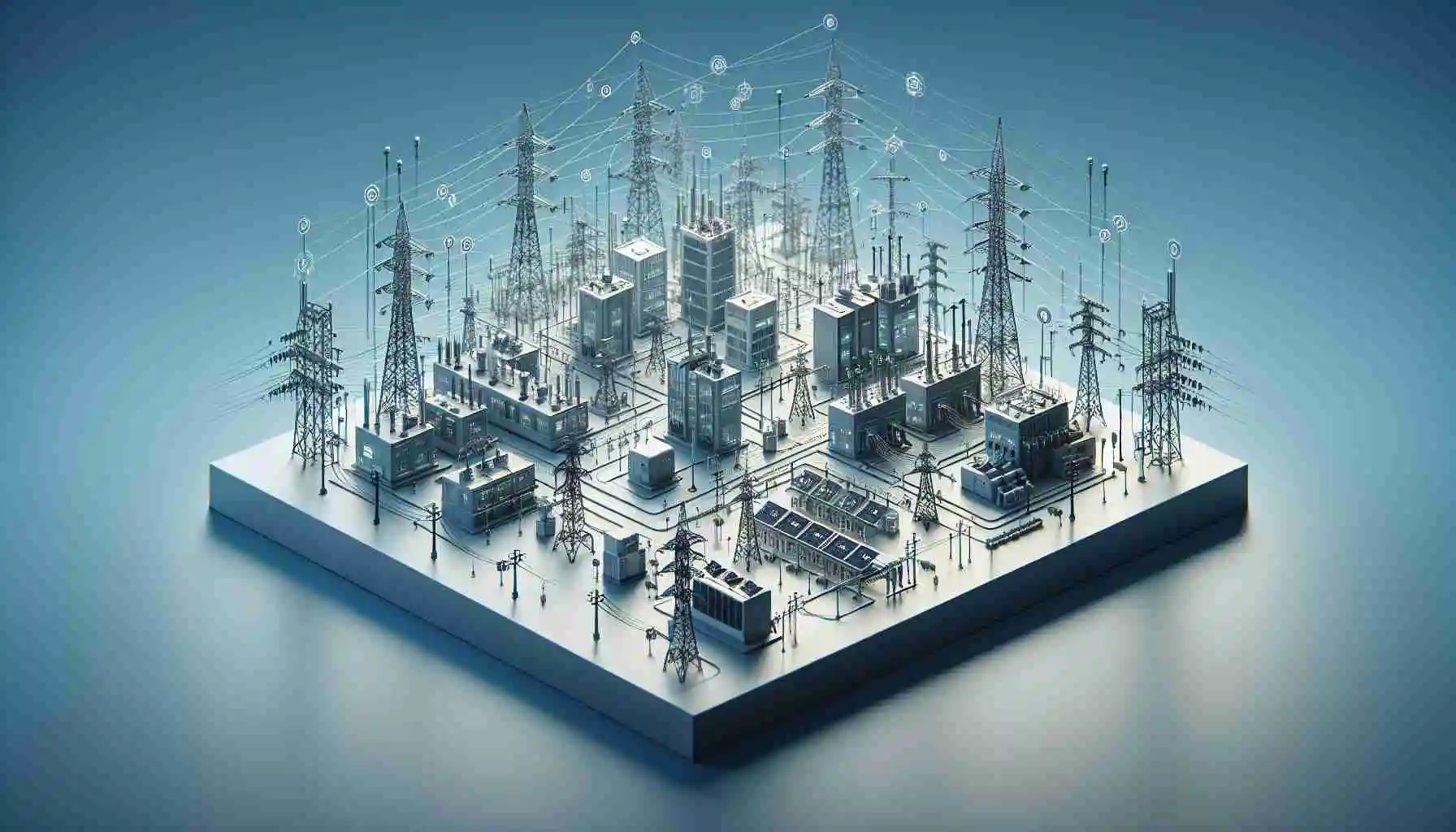
Electrical grids serve as the foundation of modern society's energy infrastructure, ensuring the reliable delivery of power to homes, businesses, and industries.
Grid monitoring systems play a crucial role in this process by continuously tracking the flow of electricity.
By doing so, they empower utilities to swiftly identify and address potential issues such as equipment failures, grid overloads, and voltage fluctuations.
Without robust grid monitoring, reducing carbon footprint and promoting renewable energy adoption would be challenging.
Harnessing AI algorithms and advanced analytics by utilities can gain deeper insights into grid performance, anticipate potential issues, and take proactive measures to enhance efficiency and resilience.
Table of Contents
- The Evolution of Grid Monitoring
- AI Solutions
- Load Forecasting and Dynamic Pricing
- Anomaly Detection and Predictive Maintenance
- Renewable Energy Integration
- Energy Theft Detection
- Security Enhancement
- Asset Management
- Demand Response Management
- Conclusion
- Frequently Asked Questions
The Evolution of Grid Monitoring
Traditional grid systems suffer from limitations in their monitoring methods.
These methods depend on manual inspections and intermittent checks, resulting in incomplete data collection and delayed problem-solving.
Moreover, they lack the necessary sophistication to analyze extensive data swiftly, impeding their predictive capabilities.
One recent tragedy highlighting the failure of a conventional grid monitoring system occurred during the severe winter storm that hit Texas in February 2021.
The extreme weather led to widespread power outages across the state, leaving millions without electricity for days in freezing temperatures.
The grid infrastructure, designed for typical weather conditions, failed to withstand the unprecedented demand for power.
Unlike traditional grids, which operate in a one-way flow of electricity from power plants to consumers, smart grids enable bidirectional communication and real-time data exchange between grid components, including power generators, transmission lines, distribution systems, and end-user devices.
AI solutions
By leveraging AI technologies, grid monitoring systems can overcome these limitations and revolutionize the energy sector.
Applications of AI in Grid Monitoring are :
- Predictive maintenance
- Load forecasting
- Renewable energy integration
- Energy theft detection
- Cybersecurity
- Asset management
- Grid optimization
- Demand response management
Load Forecasting and Dynamic Pricing
Time Series Analysis and other machine learning methods utilize historical data and real-time information for load forecasting, enabling grid operators to optimize resource allocation and prevent overloads.
Reinforcement learning based systems can adjust electricity prices in real-time according to supply and demand conditions, promoting efficient energy usage and grid balancing.
Deep Q network and deep deterministic policy gradient are popular algorithms of DRL in smart grid systems.
Anomaly Detection and Predictive Maintenance
Neural networks excel at detecting anomalies in the grid, swiftly spotting issues like voltage fluctuations or irregular energy usage patterns.
This identification enables timely intervention to address emerging problems.
Moreover, by analyzing sensor data, AI can forecast equipment failures, empowering proactive maintenance strategies that prevent downtime and minimize expenses.
Renewable energy integration
Renewable energy integration involves the seamless incorporation of renewable energy sources, such as solar and wind power, into existing power grids.
This process is crucial for transitioning to a sustainable energy future by reducing reliance on fossil fuels and mitigating climate change.
AI optimizes the integration of renewable energy sources into the grid by forecasting generation patterns and managing fluctuations to ensure stability and efficiency.
Genetic algorithms and simulated annealing optimize the scheduling and dispatch of renewable energy resources to minimize costs and maximize grid stability.
Energy theft detection
Energy theft detection is aimed at identifying and mitigating instances of unauthorized consumption or tampering with electricity meters.
Analyzing consumption patterns to detect anomalies indicative of energy theft, helping utilities reduce revenue losses and maintain fair billing practices.
Graph-based algorithms analyze the network of energy consumption connections to identify suspicious relationships or connections between meters, helping utilities pinpoint potential instances of collusion or meter tampering.
Security Enhancement
Smart systems incorporate AI-powered security encryption techniques to detect and respond to cybersecurity threats in the grid.
These algorithms continuously monitor grid data, identifying suspicious activities and potential vulnerabilities, ensuring the integrity and resilience of the infrastructure.
Asset management
Asset management is focused on optimizing the lifespan, performance, and investment decisions related to critical infrastructure components such as transformers, substations, and transmission lines.
AI-driven analytics platforms analyze asset performance data to identify inefficiencies, optimize operational parameters, and extend the lifespan of critical infrastructure components.
Demand response management
AI enables utilities to implement demand response programs by predicting consumer behavior and adjusting energy usage patterns to match supply, reducing peak demand and enhancing grid stability.
Smart grid adjust demand response measures in real-time and dynamically, such as load shedding or demand curtailment, to maintain grid stability during periods of peak demand or supply constraints.
Conclusion
Artificial Intelligence-powered grid monitoring provides a critical answer while we work to achieve sustainability while understanding the complexity of contemporary energy networks.
Utilities can improve grid resilience, streamline operations, and clear the path for a more sustainable energy future by utilizing AI.
Frequently Asked Questions
How AI is used in smart grid?
AI in smart grids optimizes energy distribution by analyzing data from sensors and meters to predict demand, prevent outages, and manage renewable energy sources efficiently.
How are power grids monitored?
Power grids are monitored using sensors, meters, and SCADA systems to track electricity flow, detect faults, and ensure reliability. Data is collected and analyzed in real-time to manage supply and demand.
What is smart grid monitoring?
Smart grid monitoring involves using advanced technology to gather and analyze data on electricity usage, generation, and distribution. It helps utilities identify inefficiencies, predict failures, and improve overall grid performance for better energy management.
What is the use of AI in microgrid?
AI in microgrids enables autonomous operation by optimizing energy generation, storage, and distribution based on demand and available resources. It can predict energy needs, balance loads, and integrate renewable sources efficiently, enhancing reliability and resilience in isolated or decentralized energy systems.
Book our demo with one of our product specialist
Book a Demo