Florence-2: Vision Model Shaping the Future of AI Understanding
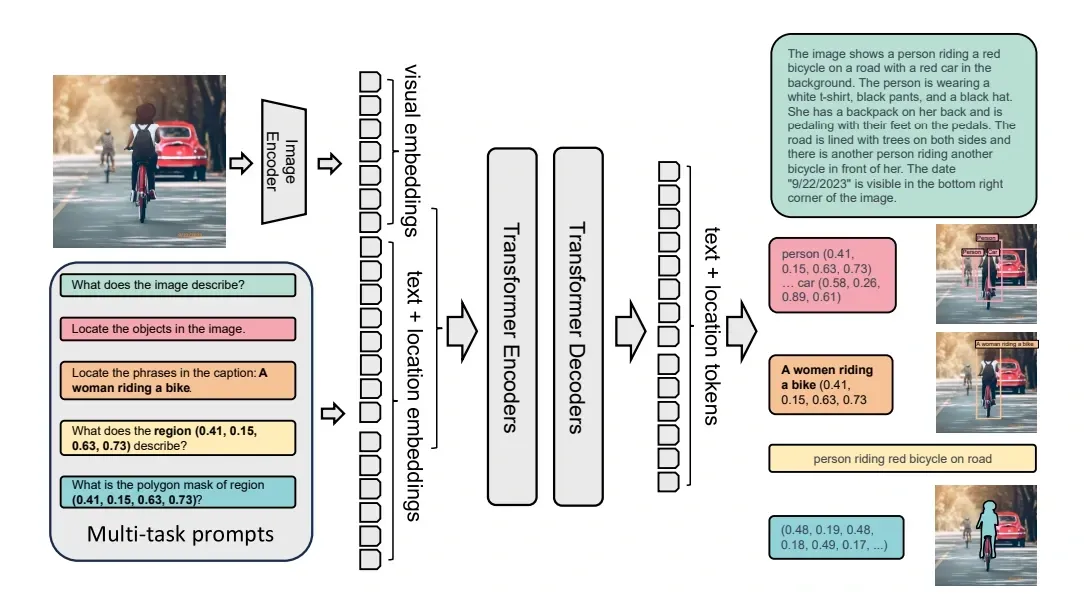
Table of Contents
- Introduction
- Florence-2: Shaping the Future of Computer Vision
- Multitask Learning for Versatility in Vision Capabilities
- Key Highlights of Florence-2's Performance
- Data Engine: Annotating the Vision Landscape
- Annotation-specific Variations
- Multitask Transfer Learning: A Quest for Superiority
- Conclusion
Introduction
In the rapidly evolving landscape of Artificial General Intelligence (AGI), the emergence of Florence-2 signifies a monumental stride forward in the realm of computer vision. Developed by a team at Azure AI, Microsoft, this state-of-the-art vision foundation model aims to redefine the way machines comprehend and interpret visual data. Let's delve into this groundbreaking advancement and explore how Florence-2 is poised to revolutionize the field of AI.
Florence-2: Shaping the Future of Computer Vision
Florence-2 represents a significant departure from conventional vision models. Unlike its predecessors, which often excelled in isolated tasks, Florence-2 adopts a multifaceted approach capable of seamlessly handling a wide array of vision and vision-language tasks. This model is specifically engineered to interpret text-based prompts, enabling it to generate precise results across diverse tasks such as captioning, object detection, grounding, and segmentation.
Overcoming Challenges in Vision Models
Traditional large-scale vision models have excelled in transfer learning but struggled when confronted with a multitude of tasks accompanied by simple instructions. Florence-2 steps in to bridge this gap by incorporating a prompt-based system adept at handling the intricate complexities of spatial hierarchy and semantic granularity in visual data.
Unlocking Florence-2's Power: The FLD-5B Dataset
At the core of Florence-2 lies the FLD-5B dataset—a colossal repository encompassing a staggering 5.4 billion comprehensive visual annotations spread across 126 million images. This dataset's development involved a meticulous iterative process of automated image annotation and model refinement. Unlike labor-intensive manual annotation, FLD-5B leverages the collective intelligence of multiple models to ensure more reliable and unbiased image understanding.
Multitask Learning for Versatility in Vision Capabilities
Florence-2 embraces a multitask learning approach, leveraging the vast FLD-5B dataset to train a sequence-to-sequence architecture. This unique design integrates an image encoder and a multi-modal encoder-decoder, empowering Florence-2 to tackle diverse vision tasks without the need for task-specific architectural modifications. Operating on textual prompts akin to Large Language Models (LLMs), Florence-2 learns from a uniform set of parameters.
Key Highlights of Florence-2's Performance
1.State-of-the-Art Zero-Shot Performance
Florence-2 achieves exceptional zero-shot performance in tasks such as captioning, visual grounding, and referring expression comprehension.
2. Competitive Fine-Tuning
Despite its compact size, fine-tuned Florence-2 competes head-to-head with larger specialist models, setting new benchmarks.
3. Enhanced Downstream Task Performance
The pre-trained Florence-2 backbone elevates performance in COCO object detection, instance segmentation, and ADE20K semantic segmentation, surpassing both supervised and self-supervised models.
4. Rethinking Vision Model Pre-training
Florence-2 ventures into three predominant pre-training paradigms—supervised, self-supervised, and weakly supervised—to overcome limitations inherent in single-task learning frameworks. By integrating innovative pre-training strategies that amalgamate textual and visual semantics, Florence-2 strides towards comprehensive image understanding.
Data Engine: Annotating the Vision Landscape
The creation of the FLD-5B dataset involves meticulous image collection from diverse sources, followed by a rigorous annotation process. The dataset encompasses annotations for text, region-text pairs, and text-phrase-region triplets, each undergoing phases of initial annotation, data filtering, and iterative refinement. This meticulous approach ensures the dataset's accuracy and quality.
Annotation-specific Variations
- Text Annotations: Florence-2 categorizes images into brief, detailed, and more detailed text descriptions, leveraging specialist models and large language models to generate comprehensive textual annotations.
- Region-Text Pairs: Descriptive textual annotations for semantic regions are obtained through a combination of object detection models, text recognition APIs, and iterative refinement techniques.
- Text-Phrase-Region Triplets: This annotation type comprises descriptive text, noun phrases related to image objects, and region annotations. Grounding DINO and SAM models aid in generating precise annotations for objects and their corresponding text.
Experimentation Overview
The experiments conducted to assess Florence-2's performance were divided into three main segments:
Zero-Shot Evaluation
Florence-2's inherent ability to handle multiple tasks without specific fine-tuning using a single generalist model was evaluated. Tasks included COCO captioning, region-level grounding, and referring expression comprehension.
Generalist Model Adaptability
Florence-2's adaptability was showcased by further training a single generalist model with additional supervised data across a wide range of tasks, achieving competitive state-of-the-art performance.
Downstream Task Performance
The performance of the learned visual representation was assessed in downstream tasks to demonstrate the superiority of Florence-2's pre-training methodology over previous approaches.
Experimentation Setup
1. Model Variants
Florence-2 comprised two model variants: Florence-2-B (232 million parameters) and Florence-2-L (771 million parameters). The models were initialized with weights from UniCL and BART architectures.
2. Training Methodology
Training employed the AdamW optimizer with cosine learning rate decay, leveraging Deepspeed and mixed precision for enhanced efficiency. The models were trained with mini-batch sizes and varying image sizes until reaching billions of effective training samples.
3. Zero-Shot Evaluation Across Tasks
Florence-2-L showcased remarkable zero-shot performance, achieving impressive scores across various tasks:
- COCO Caption Benchmark: Florence-2-L attained a CIDEr score of 135.6, outperforming models with significantly more parameters.
- Grounding and Referring Expression Tasks: Significant improvements were observed in tasks like Flickr30k Recall@1 and Refcoco referring expression segmentation.
- Generalist Model with Public Supervised Data
Florence-2 demonstrated strong performance across region-level and pixel-level tasks without specialized designs, outperforming other methods like PolyFormer and UNINEXT.
Downstream Tasks Fine-Tuning
- Object Detection and Segmentation
Florence-2's performance in COCO object detection and instance segmentation surpassed previous state-of-the-art models like ConvNext v2-B and ViT-B, showcasing higher training efficiency. - Semantic Segmentation
In ADE20k semantic segmentation, Florence-2 exhibited significant improvements over BEiT-pretrained models while maintaining a higher level of efficiency.
Multitask Transfer Learning: A Quest for Superiority
Here the comparison between three pre-trained models is made —Image-level, Image-Region, and Image-Region-Pixel—each having distinct combinations of pre-training tasks, from image-level to pixel-level granularity. The objective was to ascertain the most effective model for transfer learning across diverse computer vision tasks.
Model Comparison and Downstream Task Performance
The Image-Region-Pixel Model, pre-trained on tasks spanning image, region, and pixel levels, consistently outperformed its counterparts across four downstream tasks:
(i) COCO Caption Task
Initially lagging behind, the Image-Region-Pixel Model eventually achieved competitive performance comparable to other models.
(ii) COCO Object Detection Task
Outperformed the Image-level Model significantly and nearly matched the performance of the Image-Region Model.
(iii) Flickr30k Grounding Task
Showed robust performance comparable to the Image-Region Model and notably superior to the Image-level Model.
(iv) RefCOCO Referring Segmentation Task
Clearly outperformed both Image-level and Image-Region Models, showcasing the highest performance among all models.
Scaling Model Capacity and Data: Unraveling Key Patterns
Model Scaling Impact
Comparing base and large models (with varying parameters), the larger model consistently outperformed the base model across multiple tasks. This indicates the significance of model capacity in achieving superior performance.
Data Scaling Impact
Here we will discuss the effect of different pre-training dataset sizes on downstream task performance. Larger pre-training data sizes generally led to improved performance across various tasks, underscoring the importance of extensive data for enhancing model efficacy.
Efficient Annotation Generation for Enhanced Learning
The study employed model-generated annotations, significantly reducing reliance on human annotations. This approach not only accelerated pre-training but also facilitated a sustainable and scalable process for developing effective models.
Vision Encoder and Multi-modality Encoder-Decoder
Freezing the vision encoder degraded performance in tasks requiring region and pixel-level understanding, emphasizing the importance of unfreezing to facilitate learning in diverse tasks.
Language pre-training weights slightly benefited tasks demanding text understanding but had minimal impact on purely vision-centric tasks.
Conclusion
Florence-2 stands as a pioneering advancement in vision models, ushering in a new era of comprehensive and adaptable AI systems. With its unparalleled capabilities and innovative design, powered by the extensive FLD-5B dataset, Florence-2 marks a significant milestone in the pursuit of universal representation in computer vision.
Florence-2's experiments demonstrated its prowess in handling diverse vision tasks, showcasing competitive performance while maintaining a compact size. Its efficient training methodology and superior performance in downstream tasks position it as a valuable asset in AI research and applications.
The research findings underscore the efficacy of multitask transfer learning, particularly with models pre-trained across various task levels. Larger model capacities and datasets contribute significantly to improved performance across diverse tasks.
Frequently Asked Questions
1.What can a Florence model be used for?
Utilizing universal visual-language representations sourced from extensive image-text data on the web, the Florence model seamlessly adjusts to diverse computer vision tasks. These tasks encompass classification, retrieval, object detection, visual question answering (VQA), image captioning, video retrieval, and action recognition.
2. What is Azure Florence-vision & language?
Computers have the potential to replicate this capability by identifying the most comparable images for a given text query (or vice versa) and articulating the contents of an image using natural language. The introduction of Azure Florence-Vision and Language, abbreviated as Florence-VL, is geared toward realizing this objective. Aim with Florence-VL is to create novel foundational models that empower Multimodal Intelligence.
3. What is Project Florence?
Project Florence represents Microsoft's pioneering venture into the domain of AI Cognitive Services, dedicated to pushing the boundaries of computer vision technologies and crafting an innovative framework for visual recognition.
In this initiative, Microsoft aims to elevate the current standards of artificial intelligence by focusing on the development of cutting-edge capabilities in visual perception. Among the five human senses, vision stands out as the most relied upon, and Project Florence seeks to harness the power of advanced AI to emulate and enhance this crucial sensory function.
By leveraging state-of-the-art technology and research, Microsoft is dedicated to refining and advancing the field of computer vision, thereby revolutionizing the way we perceive, interpret, and interact with the visual world.
Book our demo with one of our product specialist
Book a Demo