6 Best Data Labeling and Annotation Tools For Automotive Industry
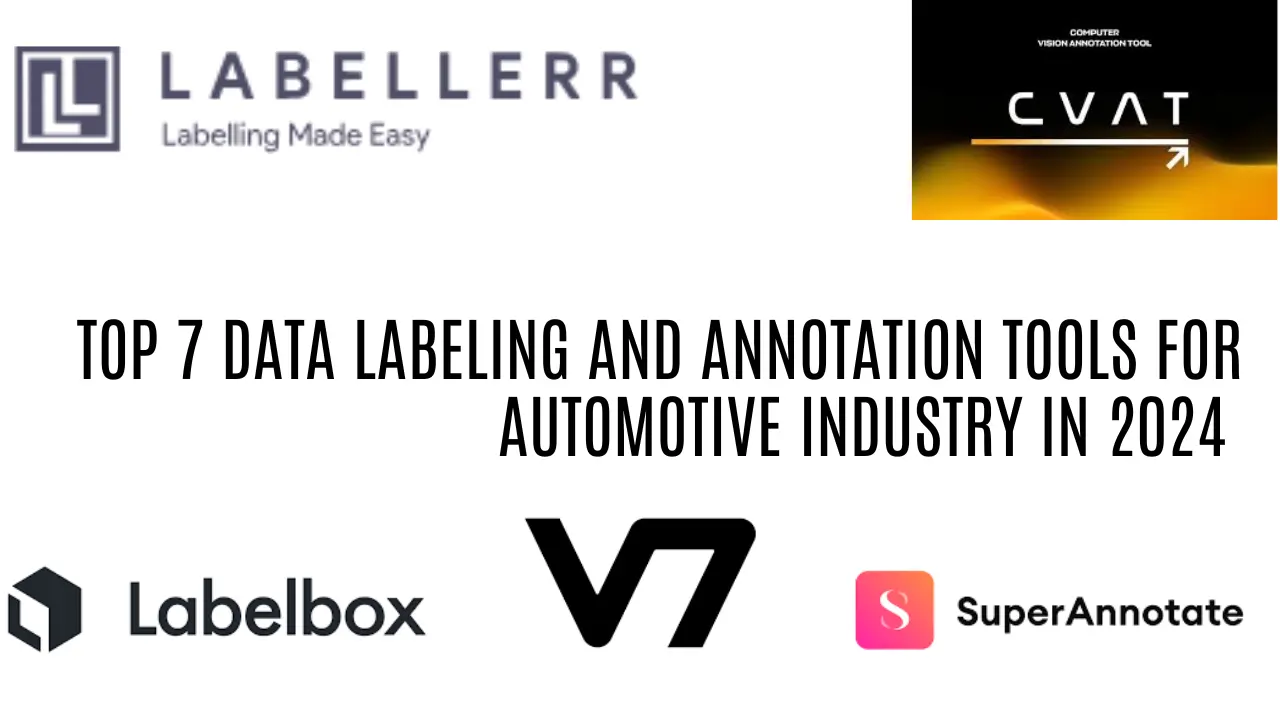
In the rapidly evolving automotive industry, the integration of artificial intelligence (AI) and machine learning (ML) is revolutionizing how vehicles operate.
From autonomous driving systems to advanced driver-assistance systems , the success of these technologies heavily depends on the availability of high-quality, annotated data.
Data labeling and annotation play a pivotal role in training these AI and ML models, ensuring they can accurately interpret and respond to various driving scenarios.
Given the complexity and safety-critical nature of automotive applications, precise and efficient data annotation is paramount. This involves labeling vast amounts of data from diverse sources, including images, videos, LiDAR, and radar sensors, to create comprehensive datasets that reflect real-world conditions.
This blog delves into the top data labeling and annotation tools specifically tailored for the automotive industry. We will explore their unique features, advantages, and how they cater to the specific needs of automotive applications.
Table of Contents
- Key Applications in the Automotive Industry
- Labellerr
- V7 Labs
- CVAT (Computer Vision Annotation Tool)
- Roboflow
- SuperAnnotate
- Labelbox
- Conclusion
- FAQs on Data Labeling and Annotation Tools for the Automotive Industry
Key Applications in the Automotive Industry
1. Car Damage Detection
Car damage detection systems leverage AI and ML to identify and assess damage to vehicles. This technology is crucial for insurance claims processing, repair estimation, and post-accident assessments, enhancing accuracy and efficiency in evaluating vehicle conditions.
Automated Damage Assessment: Quickly and accurately identifies dents, scratches, and other forms of damage using computer vision.
Insurance Claims Processing: Facilitates faster and more precise evaluation of damage claims, reducing the need for manual inspections.
Repair Cost Estimation: Provides reliable estimates for repair costs, aiding both service providers and customers in decision-making.
2. Lane Tracking
Lane tracking systems use advanced image processing and sensor data to monitor and maintain a vehicle’s position within its lane. This application is essential for enhancing road safety and supporting autonomous driving capabilities.
Lane Departure Warning: Alerts drivers when the vehicle unintentionally drifts out of its lane, preventing potential accidents.
Lane Keeping Assistance: Actively steers the vehicle to stay within lane boundaries, ensuring continuous safe driving.
Autonomous Navigation: Enables self-driving cars to accurately follow road lanes, essential for autonomous driving technology.
3. Object Detection and Recognition
Object detection and recognition systems identify and classify various objects around the vehicle, such as pedestrians, other vehicles, traffic signs, and obstacles. This application is fundamental for the safety and efficiency of autonomous driving systems.
Pedestrian Detection: Identifies and tracks pedestrians to prevent collisions and ensure pedestrian safety.
Traffic Sign Recognition: Detects and interprets traffic signs to provide real-time information to the driver or the autonomous system.
Obstacle Avoidance: Recognizes and responds to obstacles on the road, facilitating safe navigation.
4. Driver Monitoring Systems (DMS)
Driver monitoring systems use cameras and sensors to observe the driver's behavior and state, ensuring that they remain alert and focused while driving. This application enhances driver safety and can prevent accidents caused by driver fatigue or distraction.
Fatigue Detection: Identifies signs of driver fatigue, such as drooping eyelids or yawning, and issues alerts to take a break.
Distraction Monitoring: Monitors the driver’s attention and alerts them if they are not paying attention to the road.
Biometric Identification: Uses facial recognition to authenticate the driver and adjust vehicle settings accordingly.
5. Surround View Systems
Surround view systems provide a 360-degree view around the vehicle, enhancing the driver’s situational awareness during parking and low-speed maneuvers. This application is vital for preventing collisions and improving maneuverability in tight spaces.
Parking Assistance: Offers a bird’s-eye view of the vehicle’s surroundings, aiding in precise parking.
Blind Spot Detection: Identifies objects in the vehicle’s blind spots, alerting the driver to potential hazards.
Enhanced Maneuvering: Facilitates safe navigation in crowded or narrow spaces by providing comprehensive visual information.
Top Data Labeling and Data Annotation Tools for the Automotive Industry
1) Labellerr
Labellerr stands out as a powerful tool for data labeling and annotation tasks in the automotive industry. Here's a breakdown of its key features, user reviews, and pricing:
Pros:
Feature-rich Segmentation: Perform faster segmentation with pixel perfection. Drag polygon and auto-bordering features prevent overlapping adjacent objects.
Auto-labeling: Accelerate use cases with semantic segmentation using features like SAM and active learning.
Professional Annotation Team: Handle large data volumes with fast turnaround times.
Custom SLA: Starting from 24 hours for batch completion.
24/7 Tool Support: Available for the Enterprise Plan.
Robust QA Process: Set up QA processes that include agreement between annotators, comparison based on ground truth and IOU metrics, model-assisted QA, generative AI-powered QA, and sample visual quality assurance.
Dedicated Account Manager: Manage daily/weekly output efficiently.
Data Privacy and Security Compliance: Comply with HIPAA and GDPR regulations.
Multi-tier Pricing: options based on quality measurement. The default QC process includes 1 round of annotation and 1 round of QC, customizable to match the expected output, timeline, and budget.
Cons:
Limited Format Support: This does not currently support point cloud and 3D data formats.
Pricing:
Pro Plan: Starts at $499 per month for 10-user access with 50,000 data credits included. Additional data credits can be purchased at $0.01 USD per data credit, and extra users can be subscribed to at $29 USD per user.
Enterprise Plan: Offers professional services, including tool customization and ML consultancy apart from custom data, workspace, and other limitations.
2) V7 Labs
V7 Labs offers an advanced AI-powered platform designed for efficient data labeling and annotation. It is tailored to handle complex datasets, making it ideal for the automotive industry’s diverse and demanding requirements.
Automated Annotation: Utilizes AI to automatically label objects, significantly speeding up the annotation process.
Custom Workflows: Allows for the creation of custom workflows to fit specific project needs, enhancing flexibility.
Collaboration Tools: Facilitates team collaboration with features like version control and real-time updates.
Quality Assurance: Provides tools for thorough quality checks to ensure the accuracy and consistency of annotations.
3) CVAT (Computer Vision Annotation Tool)
CVAT is an open-source annotation tool developed by Intel, widely used for annotating video and image data. It is known for its robustness and flexibility, catering to the precise needs of automotive data annotation.
Wide Range of Annotation Types: Supports various annotation types such as bounding boxes, polygons, and polylines, essential for different automotive applications.
Scalability: Capable of handling large-scale annotation projects, making it suitable for extensive datasets in the automotive industry.
Integration Capabilities: Easily integrates with other tools and platforms, enhancing its utility in diverse workflows.
User-Friendly Interface: Designed with a user-friendly interface that simplifies the annotation process, even for complex tasks.
4) Roboflow
Roboflow is a versatile data labeling and annotation tool that offers robust features for creating high-quality datasets. It is particularly effective for automotive industry applications requiring detailed and accurate annotations.
Automated Preprocessing: Provides automated preprocessing steps such as image augmentation and resizing, which improve the quality and diversity of the dataset.
Annotation Management: Offers powerful annotation management features, including version control and collaborative editing, to streamline the annotation workflow.
Dataset Generation: Simplifies the generation of datasets for training machine learning models, ensuring consistency and accuracy.
Integration with ML Frameworks: Easily integrates with popular machine learning frameworks, facilitating seamless transitions from annotation to model training.
5) SuperAnnotate
SuperAnnotate is a comprehensive data annotation platform that combines advanced tools with collaboration features. It is designed to streamline the annotation process for large and complex datasets, making it highly suitable for the automotive industry.
AI-Powered Annotation: Leverages AI to assist with annotations, significantly reducing the time and effort required for manual labeling.
Collaborative Workspace: Provides a collaborative environment where multiple annotators and reviewers can work together, enhancing productivity and accuracy.
Quality Control: Includes robust quality control mechanisms to ensure high annotation standards, critical for safety-sensitive automotive applications.
Integration and Scalability: Easily integrates with existing workflows and scales to handle large datasets, making it versatile for various automotive projects.
6) Labelbox
Labelbox offers a highly scalable data labeling platform that is designed to manage large volumes of data efficiently. Its intuitive interface and powerful features make it a popular choice for automotive data annotation tasks.
Customizable Workflows: Allows users to create and customize annotation workflows to fit specific project needs, ensuring flexibility and efficiency.
Collaborative Annotation: Supports collaborative annotation, enabling teams to work together in real time, which is essential for large-scale automotive projects.
Quality Assurance Tools: Provides comprehensive quality assurance tools to maintain high annotation standards, including review and feedback mechanisms.
Data Management: Facilitates efficient data management with features like dataset versioning, analytics, and integration with ML frameworks, streamlining the overall workflow.
Conclusion:
The integration of AI and ML technologies in the automotive industry is transforming how vehicles operate, from enhancing safety features to enabling fully autonomous driving.
Central to these advancements is the quality of data used to train these sophisticated models, making data labeling and annotation a critical component of the development process.
Choosing the right data annotation tool can significantly impact the efficiency and accuracy of creating high-quality datasets. Tools like Labellerr, V7 Labs, CVAT, Roboflow, SuperAnnotate, and Labelbox each offer unique features tailored to meet the demanding needs of the automotive industry.
FAQs on Data Labeling and Annotation Tools for the Automotive Industry
1. What is data labeling and why is it important in the automotive industry?
Data labeling involves annotating data, such as images and videos, to identify and tag various elements within the data. In the automotive industry, accurate data labeling is crucial because it enables AI and ML models to learn and recognize various objects and scenarios, which is essential for applications like autonomous driving, lane tracking, and object detection.
2. What are some key features to look for in a data annotation tool for automotive applications?
Key features to look for include:
Automated Annotation: AI-assisted labeling to speed up the annotation process.
Custom Workflows: Flexibility to create project-specific workflows.
Collaboration Tools: Features that support team collaboration and real-time updates.
Quality Control: Robust mechanisms to ensure the accuracy and consistency of annotations.
Integration: Compatibility with other tools and ML frameworks.
3. How do automated annotation tools benefit the automotive industry?
Automated annotation tools benefit the automotive industry by:
Increasing Efficiency: Reducing the time and effort required for manual labeling.
Improving Accuracy: Leveraging AI to ensure consistent and precise annotations.
Scaling Projects: Handling large datasets efficiently, which is common in automotive applications.
Book our demo with one of our product specialist
Book a Demo