7 Best Data Annotation and Labeling Tools in Robotics and Manufacturing Industry
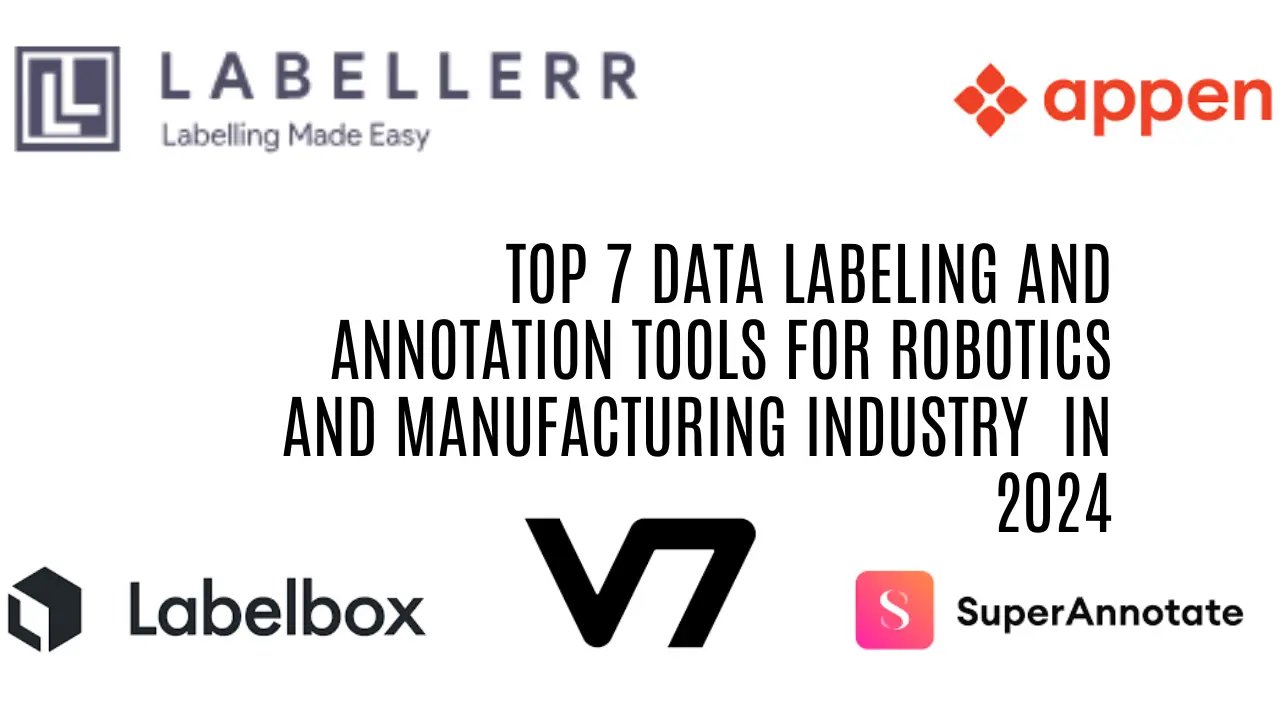
In the rapidly evolving fields of robotics and manufacturing, the integration of machine learning and artificial intelligence has become indispensable.
These technologies rely heavily on vast amounts of high-quality data to train algorithms and improve performance.
Central to this process is data labelling and annotation, a critical step that involves identifying and tagging various elements within datasets to enable machines to understand and learn from the data.
Whether it's for object detection, scene segmentation, or anomaly detection, accurate data annotation ensures that AI models can make reliable predictions and decisions.
With the increasing complexity of tasks and the need for precision, selecting the right data annotation tools is essential.
These tools not only streamline the annotation process but also enhance the quality of the labelled data, thereby boosting the overall efficacy of machine learning models.
In this blog, we will explore some of the top data labelling and annotation tools tailored for robotics and manufacturing. We will delve into their features, advantages, and how they cater to the specific needs of these industries, helping you make informed decisions in your AI implementation journey.
Table of Contents
- Top 5 Key Applications of Data Labeling in Robotics and Manufacturing
- Labellerr
- Encord
- Labelbox
- SuperAnnotate
- Kili Technology
- V7 Labs
- Appen
- Conclusion
- FAQS
Top 5 Key Applications of Data Labeling in Robotics and Manufacturing
1. Object Detection and Recognition
Identification and Localization: This enables robots to identify and accurately locate various objects within their operating environment, which is crucial for tasks such as picking, sorting, and assembly.
Automation of Repetitive Tasks: Facilitates the automation of repetitive and mundane tasks in manufacturing, increasing efficiency and reducing human error.
Enhanced Precision: Improves the precision of robotic arms and other machinery in handling components, ensuring higher quality and consistency in product manufacturing.
2. Quality Control and Inspection
Automated Visual Inspection: Uses labelled data to train systems that can detect defects, irregularities, or deviations in products, ensuring high-quality output.
Real-time Monitoring: Provides real-time monitoring and inspection of products as they move along the production line, allowing for immediate correction of any issues.
Cost Reduction: Reduces the need for manual inspection, lowering labor costs and increasing throughput while maintaining or improving quality standards.
3. Predictive Maintenance
Failure Prediction: Analyzes historical sensor data to predict equipment failures before they occur, allowing for timely maintenance.
Downtime Reduction: Minimizes unexpected equipment downtime by enabling proactive maintenance strategies, leading to continuous and efficient production.
Cost Savings: Prevents costly repairs and extends the lifespan of machinery by addressing issues before they become critical.
4. Human-Robot Interaction
Gesture Recognition: Trains robots to interpret human gestures and actions accurately, enhancing collaboration between humans and robots in shared workspaces.
Safety Improvements: Improves safety by allowing robots to recognize and appropriately respond to human presence and movements, reducing the risk of accidents.
Task Efficiency: Increases task efficiency by enabling seamless communication and coordination between human workers and robotic systems.
5. Path Planning and Navigation
Complex Environment Navigation: Equips autonomous robots with the ability to navigate through complex industrial environments safely and efficiently.
Collision Avoidance: Uses labelled data to train robots on recognizing and avoiding obstacles, ensuring smooth operation and reducing the risk of accidents.
Route Optimization: Optimizes the routes taken by autonomous robots, improving overall productivity and reducing time spent on tasks such as material transport and delivery.
Top 7 Data Labeling and Annotation Tools for Robotics and Manufacturing :
1) Labellerr
Labellerr stands out as a powerful tool for data labeling and annotation tasks in the robotics and manufacturing industries. Here's a breakdown of its key features, user reviews, and pricing:
Pros:
Feature-rich Segmentation: Perform faster segmentation with pixel perfection. Drag polygon and auto-bordering features prevent overlapping adjacent objects.
Auto-labeling: Accelerate use cases with semantic segmentation using features like SAM and active learning.
Professional Annotation Team: Handle large data volumes with fast turnaround times.
Custom SLA: Starting from 24 hours for batch completion.
24/7 Tool Support: Available for the Enterprise Plan.
Robust QA Process: Set up QA processes that include agreement between annotators, comparison based on ground truth and IOU metrics, model-assisted QA, generative AI-powered QA, and sample visual quality assurance.
Dedicated Account Manager: Manage daily/weekly output efficiently.
Data Privacy and Security Compliance: Comply with HIPAA and GDPR regulations.
Multi-tier Pricing: options based on quality measurement. The default QC process includes 1 round of annotation and 1 round of QC, customizable to match the expected output, timeline, and budget.
Cons:
Limited Format Support: This does not currently support point cloud and 3D data formats.
Pricing:
Pro Plan: Starts at $499 per month for 10-user access with 50,000 data credits included. Additional data credits can be purchased at $0.01 USD per data credit, and extra users can be subscribed to at $29 USD per user.
Enterprise Plan: Offers professional services, including tool customization and ML consultancy apart from custom data, workspace and other limitation.
2) Encord
Encord is a versatile and user-friendly data annotation platform designed to streamline the labelling process for AI and machine learning applications. It offers robust features tailored for complex data types, making it ideal for use in robotics and manufacturing.
Features:
Collaborative Annotation: Supports real-time collaboration among multiple annotators, improving efficiency and consistency.
Advanced Automation: Features tools like automated labelling and AI-assisted annotation to speed up the labelling process.
Quality Control: Includes comprehensive quality assurance workflows to ensure high accuracy in annotations.
Scalable Infrastructure: Designed to handle large volumes of data efficiently, making it suitable for industrial-scale projects.
3) Labelbox
Labelbox is a powerful data labelling platform that provides a seamless environment for annotating diverse datasets. It is particularly well-suited for complex AI projects in robotics and manufacturing, offering extensive customization and integration capabilities.
Features:
Custom Workflows: Allows the creation of custom labelling workflows tailored to specific project needs.
Integration and APIs: Easily integrates with other tools and platforms through robust APIs, enabling a smooth data pipeline.
Data Management: Offers advanced data management features, including version control and data augmentation.
Annotation Types: Supports a wide range of annotation types such as bounding boxes, polygons, and semantic segmentation, catering to various application needs.
4) SuperAnnotate
SuperAnnotate is an innovative data annotation tool designed to enhance the accuracy and efficiency of labelling tasks. It combines intuitive user interfaces with powerful features, making it an excellent choice for robotics and manufacturing applications.
Features:
User-Friendly Interface: Features an intuitive and easy-to-use interface that reduces the learning curve for annotators.
AI-Powered Tools: Utilizes AI-powered pre-annotation tools to automate and accelerate the labelling process.
Quality Assurance: Implements robust quality assurance mechanisms to maintain high standards of data accuracy.
Collaboration and Scalability: Supports collaboration among large teams and can scale to meet the demands of extensive labelling projects.
5) Kili Technology
Kili Technology is an advanced data annotation platform designed to improve the efficiency and accuracy of labelling tasks. It offers comprehensive features that support a wide range of data types and annotation needs, making it suitable for robotics and manufacturing industries.
Features:
Multimodal Data Support: Supports various data types including images, video, text, and audio, enabling versatile applications.
Integrated AI Assistance: Features AI-assisted labelling tools to automate repetitive tasks and enhance annotation speed.
Quality Management: Provides tools for rigorous quality checks and validation processes to ensure high-quality annotations.
Flexible Workflow: Allows the customization of annotation workflows to match the specific requirements of different projects.
6) V7 Labs
V7 Labs offers a robust and intuitive data annotation platform that excels in handling complex datasets required for machine learning in robotics and manufacturing. Its advanced features focus on enhancing productivity and accuracy.
Features:
AI-Driven Annotations: Utilizes AI to automate labelling and reduce manual workload, increasing efficiency.
Collaborative Tools: Facilitates teamwork with collaborative features, allowing multiple users to work simultaneously on the same project.
Customizable Interface: Offers a highly customizable user interface to fit various annotation needs and preferences.
Rich Annotation Types: Supports multiple annotation types, including 2D and 3D annotations, enabling detailed labelling for complex data.
7) Appen
Appen is a comprehensive data annotation and AI training data provider that caters to a wide range of industries, including robotics and manufacturing. Known for its scalability and quality, Appen helps businesses build high-quality datasets for their AI models.
Features:
Global Workforce: Leverages a vast, diverse, and scalable global workforce to handle large-scale annotation projects.
Expertise in Quality: Focuses on delivering high-quality annotations through rigorous quality assurance processes.
Versatile Annotations: Supports a variety of annotation types and data formats, making it adaptable to different project requirements.
End-to-End Solutions: Provides end-to-end data solutions, from collection and annotation to validation and deployment, ensuring a seamless workflow.
Conclusion
In conclusion, the field of robotics and manufacturing relies heavily on accurate data labelling and annotation to fuel the advancement of AI technologies.
The selection of the right annotation tool is crucial for optimizing efficiency, ensuring data accuracy, and ultimately driving success in AI projects within these industries.
Each of the discussed tools, whether it's Labellerr, Labelbox, SuperAnnotate, or Appen, offers unique features and capabilities tailored to the specific needs of robotics and manufacturing applications.
From advanced automation and AI assistance to comprehensive quality control and scalability, these tools empower businesses to harness the full potential of their data and accelerate innovation in robotics and manufacturing.
FAQS
Q1: What are data labelling and annotation in the context of robotics and manufacturing?
Data labelling and annotation involve identifying and tagging elements within datasets to train AI models. In robotics and manufacturing, this process is crucial for tasks such as object detection, scene segmentation, quality control, and predictive maintenance.
Q2: Why is data labelling important for robotics and manufacturing?
Data labelling is essential because it provides the necessary information for AI models to learn and make accurate predictions. High-quality annotations ensure that robots can perform tasks like object recognition, navigation, and human-robot interaction effectively and efficiently.
Q3: What are some key features to look for in a data annotation tool for robotics and manufacturing?
Important features include:
Collaborative capabilities to allow multiple users to work simultaneously.
AI-assisted automation to speed up the annotation process.
Quality assurance tools to ensure high accuracy in annotations.
Support for various data types such as images, video, text, and 3D data.
Book our demo with one of our product specialist
Book a Demo