Comprehensive Guide to Choosing the Best Data Labeling Software in 2025
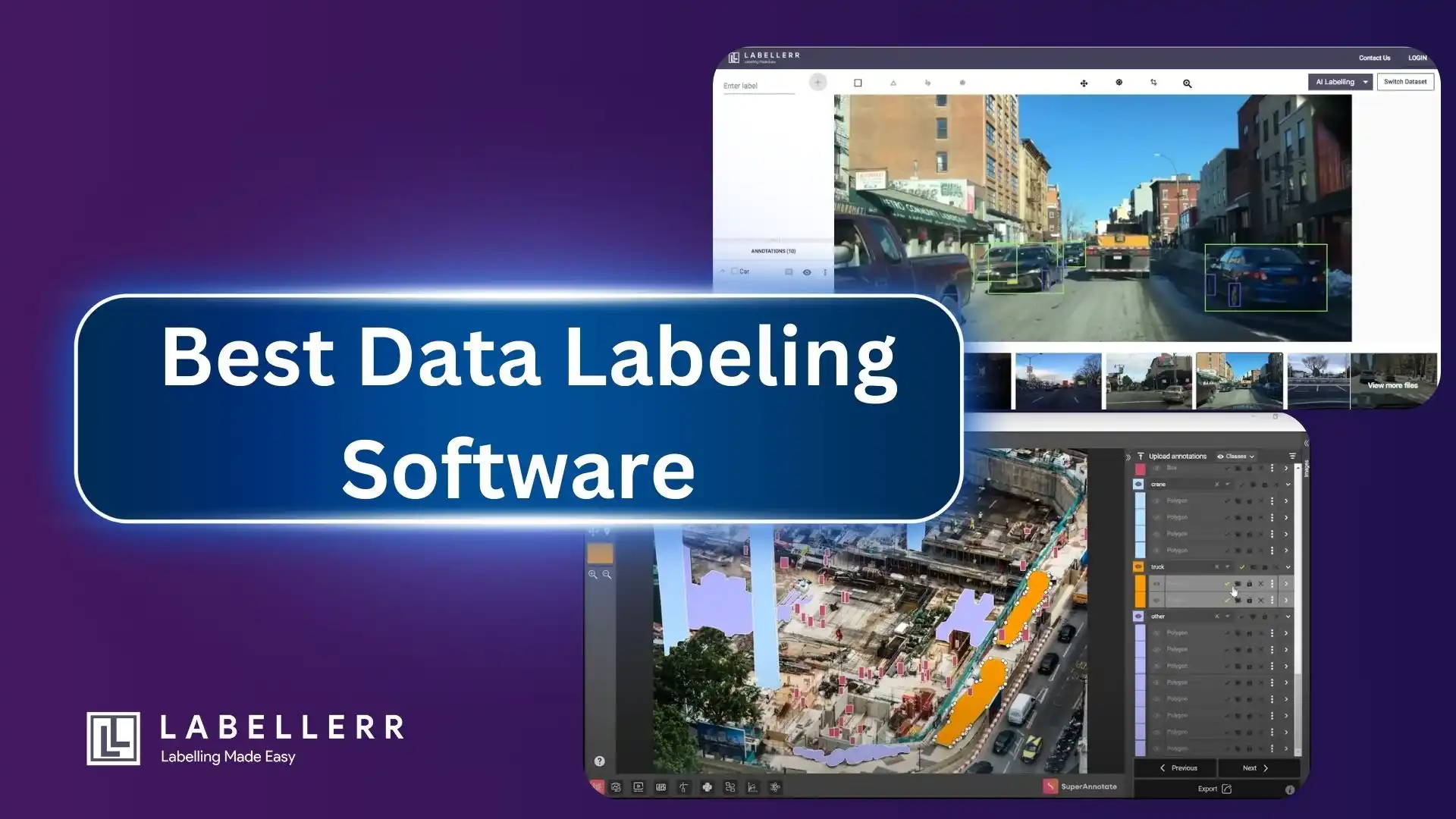
In the world of artificial intelligence (AI) and machine learning (ML), data labeling is a critical step. It determines how well your models will perform. However, many organizations struggle with data labeling inefficiencies.
Their tools are often slow, difficult to use, or lack the features needed to ensure quality and consistency. This leads to longer project timelines, higher costs, and poor model performance.
Without accurate and timely labeled data, even the most sophisticated AI models can fail.
Research shows that over 80% of AI project time is spent on data preparation, including labeling. Additionally, 57% of machine learning engineers believe poor-quality data is the biggest challenge in achieving accurate model performance.
Inefficient labeling tools often result in errors, inconsistency, and annotator burnout. In fact, studies indicate that improving the user experience (UX) of labeling tools can reduce labeling time by up to 30% and improve annotation accuracy by 20%.
To overcome these challenges, choosing the right data labeling software is essential. In 2025, as AI applications become more advanced and widespread, organizations need labeling tools that are efficient, scalable, and easy to use.
The best data labeling software offers features like intuitive user interfaces, automation, error-checking, and collaboration tools.
This guide will help you understand what factors to consider, which features are must-haves, and what trends are shaping the future of data labeling.
By selecting the right tool, you can speed up your data labeling process, reduce costs, and improve the performance of your AI models.
Table of Contents
- The Importance of Choosing the Right Data Labeling Software
- Key Factors to Consider When Choosing Data Labeling Software
- Top Data Labeling Software to Consider in 2025
- Trends Shaping Data Labeling Software in 2025
- Conclusion
- FAQs
The Importance of Choosing the Right Data Labeling Software
Choosing the right data labeling software can make your AI projects faster, more accurate, and easier to manage. It’s a critical step to achieving success in machine learning.
Impact on Model Performance
The quality of labeled data directly affects how well your AI models perform. Accurate and consistent labels help models learn better and make fewer mistakes.
Poor labeling, on the other hand, leads to unreliable predictions and lower accuracy.
Choosing the right data labeling software ensures that your annotations are precise, which improves the overall performance of your machine learning models.
Efficiency and Productivity
Good data labeling software speeds up the annotation process and makes it easier for annotators to work. Features like intuitive navigation, keyboard shortcuts, and automation tools save time and reduce repetitive tasks.
Faster labeling means you can complete projects sooner and reduce costs. When annotators work with an efficient tool, they stay productive and face less frustration.
Scalability
As your AI projects grow, you need software that can handle large amounts of data and complex tasks. The right data labeling tool can process thousands of images, videos, or text files without slowing down.
It should also support advanced labeling tasks like segmentation, 3D annotations, or multi-modal data. Scalable tools help you manage bigger projects smoothly and adapt as your needs expand.
Team Collaboration
Large labeling projects often require multiple people to work together. The right software makes collaboration easy by allowing task assignments, progress tracking, and real-time feedback.
Features like comments, review workflows, and audit logs keep everyone on the same page. Effective collaboration helps teams complete projects faster and ensures high-quality results.
Key Factors to Consider When Choosing Data Labeling Software
Factor | Description |
---|---|
Ease of Use | Intuitive interface, easy navigation, and user-friendly design. Minimal learning curve for annotators and administrators. |
Annotation Types Supported | Support for bounding boxes, polygons, segmentation masks, keypoints, 3D annotations, and text labeling. Flexibility to handle images, video, audio, and text data. |
Automation and AI-Assistance | Auto-labeling, model-assisted labeling, and pre-annotations. AI-powered suggestions to reduce manual effort. |
Collaboration and Workflow Management | Multi-user support, task assignments, and review workflows. Features like comment sections, dashboards, and task tracking. |
Quality Control and Error Prevention | Real-time validation, consistency checks, and audit logs. Tools for review, feedback, and ensuring annotation quality. |
Integration and Compatibility | Works with ML frameworks (e.g., TensorFlow, PyTorch). Integration with cloud services (AWS, Azure, GCP) and existing pipelines. |
Scalability | Handles large datasets and complex annotation tasks. Performs well with high-resolution images, videos, and bulk processing. |
Security and Data Privacy | Compliance with GDPR, HIPAA, and other regulations. Options for on-premise or cloud-based deployment. |
Customization and Flexibility | Customizable workflows, labeling templates, and interfaces. Adaptable for various industries and use cases. |
Cost and Pricing Models | Options like subscription-based, pay-as-you-go, or enterprise pricing. Consider hidden costs for storage, training, or support. |
Top Data Labeling Software to Consider in 2025
1. Labellerr
Labellerr is an AI-assisted data labeling platform designed for efficiency and accuracy. It supports various annotation types, including images, video, and text.
With an intuitive interface and automation features, Labellerr helps teams streamline labeling workflows and reduce repetitive tasks. It’s ideal for organizations that need scalable and smart labeling solutions.
Key Features
- AI-assisted annotation and pre-labeling
- Intuitive and user-friendly interface
- Real-time validation and error detection
- Collaboration tools for team-based projects
Pros
- Automation saves time
- Easy-to-use interface with minimal learning curve
- Supports multiple data types
Cons
- Limited offline functionality
- Advanced features may require training
Best For
- Teams needing AI-assisted labeling
- Organizations handling multi-modal data
- Projects requiring real-time validation
G2 Review
⭐ 4.7/5 – Praised for its automation and ease of use.
2. Labelbox
Labelbox is a collaborative data labeling platform with features for project management and model-assisted labeling. It supports a range of annotation types, making it ideal for teams that need structured workflows and seamless collaboration. Labelbox integrates easily into ML pipelines, helping manage large annotation projects efficiently.
Key Features
- Collaboration dashboard for task management
- Model-assisted labeling for faster annotations
- Project tracking and reporting tools
- Support for various annotation types
Pros
- Excellent collaboration and workflow management
- Real-time progress tracking
- Easy integration with ML models
Cons
- Expensive for large datasets
- Performance issues with very large projects
Best For
- Teams needing collaborative workflows
- Projects requiring model-assisted labeling
- Enterprises managing large-scale labeling
G2 Review
⭐ 4.5/5 – Users appreciate its collaboration features but note the high cost.
3. SuperAnnotate
SuperAnnotate offers high-quality data labeling with strong automation, quality control, and collaboration features. It supports image, video, and text annotations, making it suitable for complex projects. Its automation tools and QA workflows ensure accurate and consistent labeling, ideal for teams that focus on quality.
Key Features
- AI-assisted labeling and automation
- Quality control workflows and validation tools
- Rich annotation tools for diverse tasks
- Team collaboration and task management
Pros
- Robust automation and QA features
- Supports multiple annotation types
- Scalable for large projects
Cons
- Learning curve for beginners
- Limited free-tier features
Best For
- Teams needing strict quality control
- Complex annotation projects
- Large-scale enterprise tasks
G2 Review
⭐ 4.6/5 – Users love the QA features but mention a learning curve.
4. Amazon SageMaker Ground Truth
Amazon SageMaker Ground Truth is a managed data labeling service integrated with AWS. It offers scalable annotation workflows, automation, and customizable templates. Ground Truth is ideal for enterprises needing large-scale labeling with seamless integration into AWS-based ML pipelines.
Key Features
- Scalable, managed annotation workflows
- Built-in automation and AI-assisted labeling
- Customizable templates for various tasks
- Integration with AWS services
Pros
- Highly scalable for large datasets
- Automation reduces manual effort
- Seamless AWS integration
Cons
- Requires familiarity with AWS
- Costs can rise with large datasets
Best For
- Enterprises using AWS services
- Projects requiring scalability
- Teams handling large datasets
G2 Review
⭐ 4.3/5 – Users appreciate scalability but note AWS complexity.
5. Scale AI
Scale AI provides high-quality data labeling services with a focus on automation and scalability. It supports a wide range of annotation tasks, including images, video, and 3D data. Scale AI is known for its human-in-the-loop approach and is ideal for enterprise-level projects that require precision and speed.
Key Features
- Human-in-the-loop annotation services
- Automation tools for faster labeling
- Quality assurance workflows
- Scalable for large datasets
Pros
- High-quality and accurate annotations
- Scales efficiently for large projects
- Strong customer support
Cons
- Premium pricing
- Less suitable for small projects
Best For
- Enterprises needing large-scale labeling
- Projects requiring high accuracy
- Teams handling complex data
G2 Review
⭐ 4.4/5 – Users praise quality but mention high cost.
6. CVAT (Computer Vision Annotation Tool)
CVAT is an open-source tool for image and video annotation. It supports a variety of annotation types and is highly customizable. CVAT is ideal for developers or teams needing a free, flexible tool for computer vision projects.
Key Features
- Support for multiple annotation types (bounding boxes, polygons, etc.)
- Extensive keyboard shortcuts for efficiency
- Customizable interface and workflows
- Open-source and community-supported
Pros
- Free and open-source
- Highly customizable
- Supports various annotation tasks
Cons
- Requires technical setup
- Not very intuitive for beginners
Best For
- Developers and technical users
- Teams needing a customizable tool
- Cost-sensitive projects
G2 Review
⭐ 4.4/5 – Users appreciate flexibility but note a learning curve.
7. VGG Image Annotator (VIA)
VGG Image Annotator (VIA) is a simple, browser-based annotation tool developed by Oxford's Visual Geometry Group. It’s lightweight, requires no installation, and works well for quick annotation tasks on small datasets.
Key Features
- Minimalist interface for simple tasks
- No installation required (browser-based)
- Exports in multiple formats (JSON, CSV)
- Lightweight and fast performance
Pros
- Easy to use and fast
- No setup needed
- Free and lightweight
Cons
- Limited features for complex tasks
- Not suitable for large-scale projects
Best For
- Quick image and video annotations
- Small datasets and simple tasks
- Users who need a lightweight tool
G2 Review
⭐ 4.2/5 – Users like simplicity but want more features.
8. Appen
Appen offers managed data labeling services with a global workforce. It supports various annotation types, including image, text, and audio. Appen is ideal for enterprises that need large-scale data labeling with quality control and project management.
Key Features
- Managed workforce for labeling tasks
- Quality control and review workflows
- Support for diverse data types
- Scalable for enterprise projects
Pros
- Access to a global workforce
- High-quality annotations
- Good for complex datasets
Cons
- High cost for large projects
- Limited customization options
Best For
- Enterprises needing managed services
- Projects with diverse data types
- Large-scale labeling tasks
G2 Review
⭐ 4.2/5 – Users praise workforce quality but note high costs.
9. Dataloop
Dataloop is an end-to-end platform for data labeling, automation, and AI pipeline management. It supports various annotation types and integrates seamlessly with ML workflows, making it ideal for teams managing the full AI lifecycle.
Key Features
- Automation tools for labeling
- Workflow management and collaboration
- Integration with ML pipelines
- Supports multiple data types
Pros
- Strong automation features
- Flexible and customizable workflows
- Supports end-to-end AI management
Cons
- Steep learning curve for advanced features
- Can be costly for large projects
Best For
- Teams managing AI pipelines
- Projects needing automation
- Enterprises with complex workflows
G2 Review
⭐ 4.5/5 – Users like automation but mention complexity.
Trends Shaping Data Labeling Software in 2025
1. Increased Use of AI and Automation
In 2025, more data labeling tools will integrate AI-powered features to make the annotation process faster and more accurate. Expect to see:
- AI-Assisted Labeling: Tools that automatically generate labels for annotators to review and correct.
- Real-Time Suggestions: AI-driven recommendations that speed up repetitive tasks like labeling similar objects.
- Smart Pre-Annotations: Pre-labeled datasets using AI models to reduce initial manual effort.
2. Focus on Quality and Validation
High-quality labeled data is critical for effective AI models. Tools in 2025 will focus heavily on improving data quality through:
- Advanced Error Detection: Real-time checks to catch common mistakes like overlapping labels or incorrect categories.
- Validation Rules: Built-in rules to ensure annotations meet specific guidelines.
- Automated Quality Control: Features that allow for multi-step review workflows and automated feedback.
3. Scalability for Big Data
As datasets grow, data labeling tools need to keep up. Trends include:
- Optimized Performance: Tools designed to process large datasets, high-resolution images, and long videos without lag.
- Batch Processing: Ability to label and export large volumes of data at once.
- Cloud Scalability: Solutions that leverage cloud infrastructure for large-scale labeling projects.
4. Integration with ML Ops Pipelines
Seamless integration with machine learning operations (MLOps) will become more common. Expect tools to offer:
- End-to-End Workflow Support: From data labeling to model training and deployment.
- API Integrations: Connect with popular ML frameworks like TensorFlow and PyTorch.
- Version Control: Track changes in datasets and models over time.
5. Data Privacy and Security
With stricter regulations on data privacy, labeling tools in 2025 will offer:
- Compliance Features: Support for GDPR, HIPAA, and other regulations.
- Data Encryption: Protect data during storage and transfer.
- On-Premise Options: Solutions that let organizations host data labeling tools on their own infrastructure.
6. Support for Multi-Modal Data
AI applications increasingly require labeling of different data types. Tools will evolve to handle:
- Images, Text, Audio, and Video: Unified platforms that support all major data types.
- Synchronized Annotation: Labeling across different formats simultaneously (e.g., video frames with audio transcription).
Conclusion
Choosing the right data labeling software is a crucial step in ensuring the success of your AI and machine learning projects. The quality of your labeled data directly impacts model accuracy, efficiency, and overall outcomes.
A well-chosen tool can streamline workflows, reduce errors, and save valuable time and resources. By investing in software with features like AI-assisted automation, intuitive interfaces, quality control mechanisms, and collaboration tools, you can boost efficiency, enhance accuracy, and handle large datasets with ease.
As the landscape of AI continues to evolve, staying aware of trends like increased automation, stronger data privacy measures, and support for multi-modal data will help you make informed decisions.
Carefully evaluate your project requirements, prioritize essential features, and test tools through demos and user feedback before making a final choice.
In 2025, the right data labeling software will not just be a tool — it will be a strategic asset that helps you stay ahead of the competition, deliver high-quality AI models, and achieve your business goals efficiently.
FAQs
Q1: What is the best data labeling software for small teams?
Labellerr is a great choice for small teams. Its flexible pricing makes it affordable, and the easy interface saves time.
Q2: How does data labeling software improve machine learning models?
Properly labeled data ensures machine learning models understand patterns better, improving accuracy in predictions.
Q3: Can I use open-source tools like Label Studio instead of paid solutions?
Open-source tools like Label Studio are great for tech-savvy users. However, tools like Labellerr offer automation and strong support, which save time and effort.
Q4: What industries benefit most from data labeling software?
Many industries, including healthcare, retail, finance, and autonomous driving, use labeled data to power AI projects.
Book our demo with one of our product specialist
Book a Demo