Complete Guide to Annotation Software in 2025
Discover the top annotation software in 2025, designed to streamline AI training with automation, AI-assisted labeling, and collaboration tools. Learn how these platforms improve accuracy, efficiency, and scalability for data annotation across various industries.
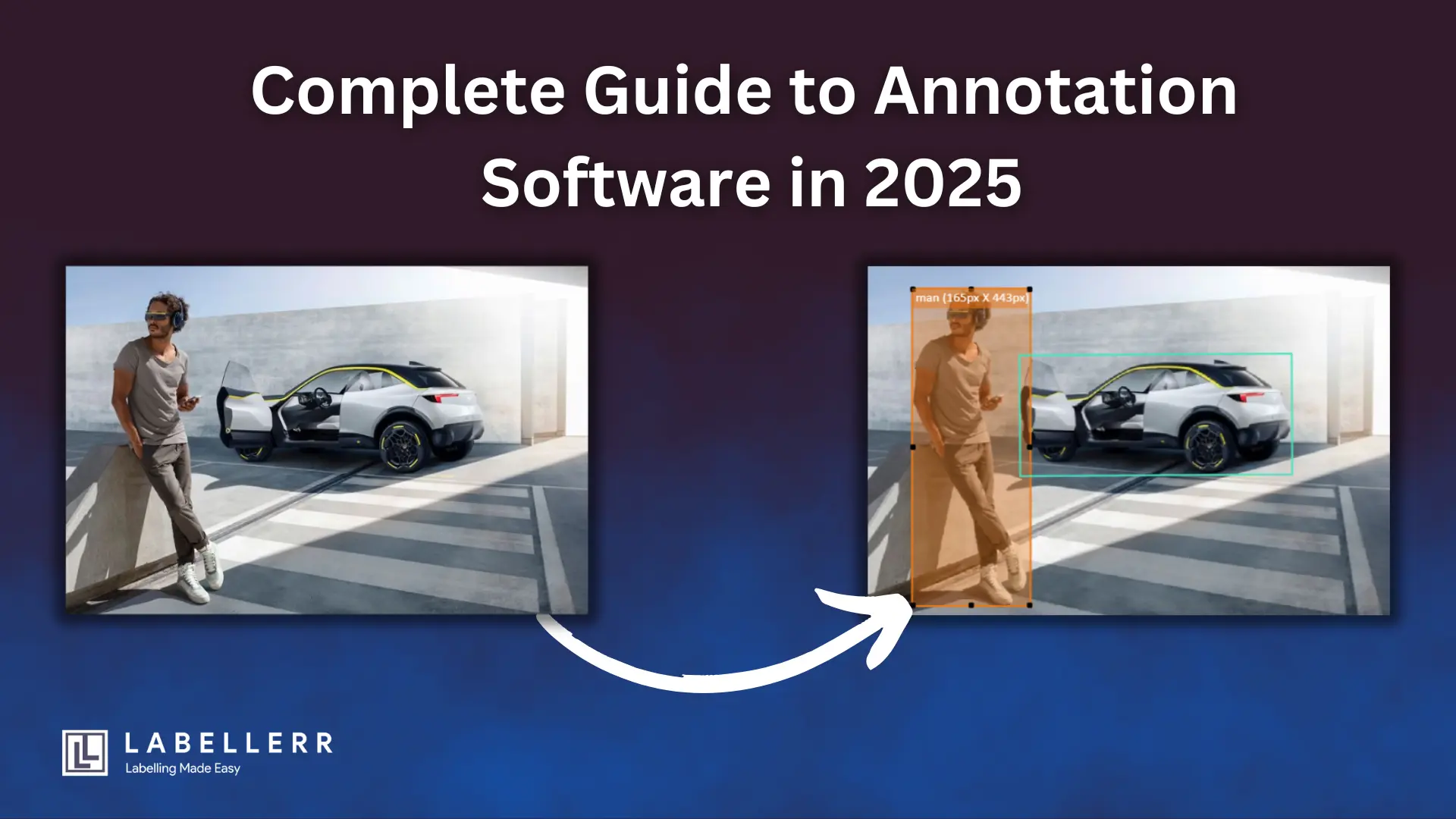
AI and machine learning models rely on high-quality training data to make accurate predictions. Without properly labeled data, AI systems struggle to understand patterns, recognize objects, or process language correctly.
Annotation software plays a crucial role in solving this problem by labeling and structuring raw data into meaningful datasets.
The demand for annotation software is growing rapidly as AI adoption increases across industries.
According to industry estimates, the global data annotation market is expected to reach $8.22 billion by 2028, with an annual growth rate of 26.2%.
In this guide, you will learn:
- What annotation software is and how it works.
- The different types of annotation tools and their applications.
- Key features to consider when choosing the right annotation tool.
- Real-world industry use cases where annotation software is making an impact.
- How to select the best software for your AI project.
Whether you are a startup, an enterprise, or a researcher working on AI projects, choosing the right annotation software can streamline your workflows, improve data accuracy, and accelerate model training.
This guide will help you understand, compare, and implement the best annotation software for your specific needs.
Table of Contents
- What Is Annotation Software?
- Types of Annotation Software
- Key Features to Look for in Annotation Software
- Benefits of Using Annotation Software
- Industry Use Cases of Annotation Software
- How to Choose the Right Annotation Software
- Best Annotation Software in 2024
- Future Trends in Annotation Software
- Conclusion
- FAQs
What Is Annotation Software?
Annotation software is a tool that helps label and tag images, text, videos, and audio so that AI models can learn from structured data.
It is essential for training machine learning algorithms to recognize patterns, classify objects, and make accurate predictions.
Without properly labeled data, AI systems cannot function effectively, making annotation software a key component in AI development.
How Annotation Software Works
The annotation process involves several steps that transform raw data into structured datasets for AI training:
- Uploading Raw Data:
Users upload the images, videos, text, or audio files that need to be annotated. These datasets are the foundation for training AI models. - Annotation Tools and Techniques:
The software provides a variety of tools to label the data, including:- Bounding Boxes – Drawing rectangular boxes around objects for object detection models.
- Polygons – Outlining irregular shapes for more precise labeling.
- Key Points – Marking specific locations on objects (e.g., facial landmarks).
- Segmentation – Assigning each pixel to a category for detailed image understanding.
- Manual or AI-Assisted Labeling:
- In manual annotation, human annotators carefully label the data to ensure accuracy.
- In AI-assisted annotation, machine learning models help pre-label the data, which is then refined by human reviewers. This speeds up the process and reduces human effort.
- Training AI Models:
Once labeled, the annotated data is fed into AI algorithms, allowing them to learn patterns, recognize objects, process language, or make predictions. - Quality Assurance and Validation:
To ensure accuracy and consistency, annotation software includes quality checks such as:- Inter-annotator agreement, where multiple reviewers verify the correctness of labels.
- Confidence scoring, where AI predicts the accuracy of labels for human review.
- Anomaly detection, which flags potential labeling mistakes.
Why Annotation Software Is Important
Annotation software plays a crucial role in improving AI model performance by ensuring that training data is:
✔ Accurate – Correct labels help AI make precise predictions.
✔ Consistent – Standardized annotations ensure uniform data quality.
✔ Efficient – AI-assisted labeling speeds up the process and reduces human effort.
✔ Scalable – Large datasets can be labeled quickly, supporting AI projects of all sizes.
Many leading AI companies and research teams rely on annotation software to build advanced models for applications such as autonomous driving, healthcare diagnostics, and natural language processing.
Types of Annotation Software
Different AI projects require different types of annotation software, depending on the type of data they process. The four main types of annotation software are image, text, video, and audio annotation tools, each designed to meet specific AI needs.
Image Annotation Software
Image annotation software is used in computer vision applications, where AI models learn to identify, classify, and detect objects in images. It is widely used in industries such as autonomous driving, healthcare, security, and retail.
Common Annotation Types in Image Labeling:
- Bounding Boxes – Drawing rectangular boxes around objects for object detection models (e.g., detecting cars in traffic).
- Polygons – Outlining irregular shapes for more precise object recognition (e.g., identifying crop diseases in agriculture).
- Semantic Segmentation – Assigning every pixel in an image to a specific category (e.g., segmenting lungs in medical imaging).
- Key Points – Marking specific locations on objects (e.g., facial recognition for security systems).
Example Image Annotation Tools:
- Labellerr – AI-assisted image annotation with automation features.
- Labelbox – Scalable platform for labeling large datasets.
- CVAT – Open-source annotation tool for image and video labeling.
- SuperAnnotate – Annotation platform designed for complex computer vision tasks.
Text Annotation Software
Text annotation software is essential for Natural Language Processing (NLP) applications, where AI models learn to understand and process human language.
Industries such as customer support, healthcare, and finance use text annotation to train chatbots, analyze sentiment, and extract information from documents.
Common Annotation Types in Text Labeling:
- Named Entity Recognition (NER) – Identifying names, locations, dates, and other key entities in text (e.g., detecting company names in financial documents).
- Part-of-Speech Tagging – Labeling words as nouns, verbs, adjectives, etc., to help AI understand sentence structures.
- Relationship Mapping – Analyzing connections between words or phrases to improve AI understanding (e.g., mapping relationships between symptoms and diseases in medical texts).
Example Text Annotation Tools:
- Prodigy – AI-assisted annotation tool for training NLP models.
- LightTag – Web-based platform for collaborative text labeling.
- Doccano – Open-source text annotation tool for sentiment analysis and classification.
Video Annotation Software
Video annotation software is used in AI applications where motion tracking and sequential frame analysis are required. This includes industries like autonomous vehicles, sports analytics, security surveillance, and robotics.
Common Annotation Types in Video Labeling:
- Object Tracking – Detecting and following objects across multiple video frames (e.g., tracking pedestrians in self-driving cars).
- Frame-by-Frame Labeling – Manually annotating each frame to train AI models (e.g., recognizing suspicious activities in surveillance footage).
- Keyframe Interpolation – Annotating keyframes while allowing AI to interpolate the frames in between (e.g., motion capture in gaming and animation).
Example Video Annotation Tools:
- Labellerr – AI-powered video annotation with automation features.
- V7 Labs – Advanced video labeling platform with deep learning capabilities.
- Supervisely – Video and image annotation tool with AI-powered automation.
Audio Annotation Software
Audio annotation software is used to train AI models in speech recognition, voice assistants, and emotion detection. It is widely used in virtual assistants (e.g., Alexa, Siri), customer service automation, and voice-based security systems.
Common Annotation Types in Audio Labeling:
- Speech-to-Text Transcription – Converting spoken words into written text (e.g., AI-driven subtitles in videos).
- Speaker Diarization – Identifying and labeling different speakers in an audio file (e.g., distinguishing between customer and support agent in a call center).
- Phonetic Tagging – Labeling specific sounds and phonemes to help AI recognize different accents and speech patterns (e.g., voice recognition for multilingual AI).
Example Audio Annotation Tools:
- Sonix – AI-driven transcription and audio annotation tool.
- Audacity – Open-source audio editing and labeling software.
- Speechmatics – AI-powered speech recognition and transcription platform.
Key Features to Look for in Annotation Software
Choosing the right annotation software is essential for efficient data labeling and high-quality AI model training.
The best tools include features that improve speed, collaboration, accuracy, scalability, integration, and security. Below are the key features to consider when selecting annotation software for your AI projects.
AI-Assisted Labeling
AI-assisted labeling helps reduce manual effort by automating parts of the annotation process. This feature uses machine learning models to pre-label objects, making annotation faster and more efficient.
How It Works:
- Auto-labeling detects and tags objects automatically based on pre-trained AI models.
- Smart predictions suggest labels based on patterns in existing data.
- AI-assisted corrections refine labels to reduce errors and inconsistencies.
Benefits:
- Speeds up data annotation, reducing time-to-market for AI projects.
- Improves accuracy by minimizing human errors.
- Helps annotators focus on refining complex data points instead of repetitive tasks.
Collaboration & Workflow Management
For large AI projects, annotation often involves multiple team members working on the same dataset. A good annotation tool supports teamwork and workflow management to improve efficiency.
Key Features:
- Multi-user support allows multiple annotators to work on the same dataset simultaneously.
- Role-based access control assigns different permissions (e.g., annotators, reviewers, project managers).
- Task assignment and tracking help manage workflows and deadlines.
Benefits:
- Enables seamless collaboration between remote teams.
- Reduces errors by allowing multiple reviewers to validate annotations.
- Improves efficiency with clear role distribution and progress tracking.
Data Quality & Validation
High-quality labeled data is crucial for training accurate AI models. Annotation software should have built-in quality assurance features to maintain consistency.
Key Features:
- Inter-annotator agreement (IAA): Measures how consistently different annotators label the same data.
- Confidence scoring: Assigns scores to labels based on how certain the AI model is about the annotation.
- Anomaly detection: Identifies outliers or incorrect labels for human review.
Benefits:
- Improves data accuracy by maintaining consistent labeling standards.
- Reduces errors and rework by identifying mistakes early.
- Ensures that AI models learn from clean, high-quality data.
Scalability & Performance
As AI projects grow, the ability to handle large datasets efficiently becomes critical. The right annotation tool should be scalable and capable of managing millions of data points.
Key Features:
- Cloud-based solutions: Allow teams to access and annotate data from anywhere.
- Batch processing: Enables large-scale annotation by labeling multiple data points at once.
- Optimized performance: Ensures smooth handling of high-resolution images, long videos, or large text datasets.
Benefits:
- Supports growing AI projects without performance slowdowns.
- Enables remote teams to collaborate on large-scale datasets in real time.
- Handles high-volume annotation tasks efficiently.
Integration with ML Pipelines
Seamless integration with machine learning pipelines ensures that annotated data can be used directly for training AI models.
Key Features:
- Supports common annotation formats like COCO, YOLO, and Pascal VOC for easy export.
- Compatibility with ML frameworks such as TensorFlow, PyTorch, and OpenCV.
- Cloud integration with AWS, GCP, and Azure for smooth data transfer.
Benefits:
- Eliminates manual file conversion and speeds up AI model training.
- Allows AI teams to automate data labeling, training, and validation workflows.
- Reduces setup time by ensuring compatibility with existing ML tools.
Security & Compliance
Data security is a major concern, especially for sensitive industries like healthcare, finance, and autonomous vehicles. Annotation software must comply with global privacy regulations to protect data integrity.
Key Features:
- Data encryption ensures secure storage and transfer of sensitive information.
- Access control mechanisms restrict data access to authorized users only.
- Compliance with industry regulations such as:
- GDPR (General Data Protection Regulation) – Europe
- HIPAA (Health Insurance Portability and Accountability Act) – Healthcare
- CCPA (California Consumer Privacy Act) – U.S. consumer data
Benefits:
- Protects sensitive data from cyber threats and unauthorized access.
- Ensures compliance with legal and ethical data handling standards.
- Provides audit logs for tracking data usage and access.
Benefits of Using Annotation Software
Annotation software plays a crucial role in making AI development more efficient, accurate, and scalable.
Businesses and research teams rely on these tools to speed up data labeling, reduce costs, improve data quality, and handle large datasets efficiently. Below, we explore the key benefits of using annotation software in AI projects.
Faster AI Development
AI models require large amounts of labeled data to learn patterns and make accurate predictions. Manually annotating this data can take weeks or even months, delaying the entire AI development process.
Annotation software speeds up this process by up to 70% through automation and AI assistance.
- AI-assisted labeling helps pre-label data using machine learning models, reducing manual effort.
- Automated workflows assign tasks efficiently, allowing multiple annotators to work simultaneously.
- Real-time collaboration tools enable faster reviews and corrections, preventing bottlenecks in annotation projects.
For example, in autonomous vehicle training, AI-powered annotation tools can automatically detect objects like pedestrians and traffic signs, reducing the need for manual labeling.
This allows self-driving models to be trained faster and more accurately.
Cost Savings
One of the biggest advantages of using annotation software is the reduction in operational costs.
Traditional manual annotation requires large teams of human annotators, which increases labor expenses. Automated labeling minimizes these costs by reducing the need for human intervention.
- AI-assisted tools handle repetitive labeling tasks, cutting down on the number of human annotators required.
- Efficient data pipelines reduce delays and ensure smooth project execution, saving both time and money.
- Faster project completion leads to quicker deployment of AI solutions, maximizing return on investment (ROI).
For instance, an e-commerce company using image annotation software can automatically categorize and tag thousands of product images without hiring a large team of data labelers.
This not only saves costs but also ensures that new product listings are processed quickly.
Improved Data Quality
AI models perform best when trained on high-quality, accurately labeled data. Poor labeling can introduce bias and errors, leading to unreliable predictions.
Annotation software ensures consistent and precise labeling through AI-driven error detection and quality control mechanisms.
- Confidence scoring highlights uncertain labels, allowing human annotators to review and correct them.
- Anomaly detection automatically identifies incorrect or inconsistent annotations.
- Inter-annotator agreement tools ensure different annotators label data consistently, reducing bias.
For example, in medical imaging AI, annotation software helps label X-rays and MRIs with high precision, ensuring that AI models can accurately detect diseases like cancer or fractures.
AI-powered quality control features flag any inconsistencies, reducing the risk of misdiagnosis.
Scalable for Large Projects
AI projects often require massive datasets to train models effectively, especially in industries like healthcare, autonomous driving, and finance.
Manually handling such datasets is time-consuming and difficult to scale. Annotation software makes it possible to manage and label millions of data points efficiently.
- Cloud-based annotation tools allow teams to work on large datasets without hardware limitations.
- Automated workflows distribute tasks efficiently, ensuring large datasets are labeled quickly.
- Parallel processing capabilities allow multiple annotators or AI models to work on the data simultaneously.
For instance, in autonomous vehicle training, companies must label thousands of hours of dashcam footage and LiDAR sensor data.
Annotation software with automated object detection and real-time tracking enables companies to scale their annotation processes efficiently, making it possible to handle large datasets without sacrificing accuracy.
Industry Use Cases of Annotation Software
Annotation software is essential for training AI models across different industries. It helps structure raw data by labeling images, text, audio, and video so that machine learning algorithms can learn and make accurate predictions.
Various industries rely on high-quality labeled datasets to improve automation, decision-making, and efficiency. Below are some key industry use cases of annotation software.
Healthcare
The healthcare industry is increasingly using AI-powered solutions for medical imaging, diagnosis, and treatment planning.
However, AI models require accurately labeled medical data to function effectively. Annotation software helps doctors and researchers train AI models for disease detection, diagnosis, and patient care.
- Medical Image Annotation: AI systems rely on labeled X-rays, MRIs, and CT scans to detect diseases like cancer, fractures, and tumors.
- AI-Assisted Diagnosis: By analyzing annotated medical images, AI can assist doctors in identifying diseases faster and recommending treatment options.
- Treatment Planning: AI-powered annotation tools help map out treatment areas for radiation therapy, ensuring precision and reducing human errors.
For example, AI-powered radiology software uses annotation tools to label lung nodules in chest X-rays, helping radiologists detect early signs of lung disease. This speeds up diagnosis and improves patient outcomes.
Retail & E-Commerce
Retail and e-commerce businesses use annotation software to enhance customer experience and optimize operations. AI models trained on labeled data improve product recommendations, inventory management, and customer sentiment analysis.
- Product Image Annotation: AI-powered recommendation engines use annotated product images to suggest relevant items to shoppers based on their preferences.
- Visual Search: Annotation tools label product images with specific attributes (e.g., color, size, style) to help AI models identify similar products in searches.
- Sentiment Analysis: AI systems analyze customer reviews and social media posts to understand customer sentiment and improve marketing strategies.
For example, Amazon and Shopify use image annotation to help customers find similar products by analyzing visual patterns in product photos. AI-powered chatbots also use text annotation to understand customer queries and provide personalized responses.
Autonomous Vehicles
Self-driving cars rely on massive datasets of annotated images and videos to understand their surroundings. Annotation software labels road objects, helping AI models recognize and react to real-world scenarios.
- Object Detection: AI models learn to detect pedestrians, vehicles, traffic signs, and lane markings from labeled images.
- Sensor Fusion with LiDAR: Annotation software integrates camera footage, LiDAR point clouds, and radar data to help autonomous vehicles interpret depth and distance accurately.
For example, Tesla and Waymo use large-scale annotated datasets to train self-driving cars. AI algorithms process labeled road signs, lane boundaries, and obstacles to make real-time driving decisions.
Agriculture
AI-powered solutions in agriculture improve crop monitoring, pest detection, and irrigation planning. Annotation software helps label satellite images, drone footage, and soil condition reports, enabling farmers to make data-driven decisions.
- Pest Detection: AI models analyze annotated images of crops to identify pests, diseases, and nutrient deficiencies.
- Precision Farming: Annotation tools help AI monitor plant health, optimize fertilizer use, and predict yield.
- Irrigation Planning: AI-assisted labeling of soil moisture data helps optimize water usage, reducing waste and improving crop health.
For example, John Deere and Bayer use AI-powered annotation tools to detect plant diseases and analyze soil conditions, enabling farmers to improve productivity while reducing costs.
Finance & Banking
The finance industry relies on AI-driven solutions for fraud detection, document processing, and risk assessment. Annotation software helps label financial transactions, legal documents, and customer interactions, allowing AI to detect fraud and automate compliance checks.
- Fraud Detection: AI models trained on labeled financial transactions can identify suspicious activities and flag potential fraud in real-time.
- Document Processing: AI uses text annotation to classify bank statements, invoices, and legal contracts, making compliance checks faster and more efficient.
For example, banks and fintech companies use AI-powered annotation tools to scan and classify millions of transactions daily, reducing the risk of fraud and automating tedious paperwork.
How to Choose the Right Annotation Software
Choosing the right annotation software is important for ensuring high-quality labeled data for AI models. The right tool can increase efficiency, reduce costs, and improve accuracy. Below are key factors to consider before making a decision.
Identify Your Project Needs
Before selecting annotation software, you need to define what kind of data you are working with and what level of automation you require.
- What type of data needs annotation?
- Are you working with images, videos, text, or audio?
- Different annotation tools specialize in specific data types, so choosing one that supports your requirements is essential.
- Do you need AI-assisted annotation or manual labeling?
- AI-assisted annotation speeds up the process with features like pre-labeling, smart predictions, and automation.
- If your project requires highly accurate and complex labels, such as medical images or legal text, manual annotation with AI-powered quality control may be a better choice.
For example, an autonomous vehicle project requires video annotation software with object tracking and sensor fusion capabilities, while an NLP project may need text annotation tools with sentiment tagging and entity recognition.
Compare Features & Pricing
Every annotation tool comes with different features and pricing models. You should compare them based on your project’s requirements and budget.
- Look for collaboration, automation, and integration.
- If you have a team working on annotations, choose software with multi-user support, real-time collaboration, and role-based access control.
- Some tools offer AI-assisted labeling, reducing manual workload and increasing speed.
- Integration with machine learning frameworks (like TensorFlow, PyTorch) and cloud platforms (like AWS, GCP) is useful for seamless AI model training.
- Evaluate cost models: Subscription vs. Pay-per-label.
- Some tools charge a fixed subscription fee, which is best for teams that handle high volumes of data regularly.
- Other tools use a pay-per-label model, where you pay only for the number of annotations made, making it a good option for smaller projects.
For example, a large AI startup working on multiple projects may prefer a subscription-based tool like Labelbox, while a research team working on a short-term project may opt for a pay-per-use platform like SuperAnnotate.
Test the Tool Before Buying
Before making a final decision, you should test the annotation tool to check its usability, features, and compatibility with your workflow.
- Use free trials or demo versions before purchasing.
- Most annotation software providers offer trial versions or demo access to explore features.
- Testing the tool helps you evaluate whether it meets your needs in terms of speed, accuracy, and ease of use.
- Check for customization options.
- Some tools allow you to customize annotation workflows based on your project’s complexity.
- If your AI project requires specific labeling formats, such as 3D bounding boxes for LiDAR data or multi-language text tagging, ensure the tool supports these features.
For instance, autonomous vehicle companies often test tools like Labellerr or CVAT to ensure they can handle complex object tracking and sensor fusion before committing to a paid plan.
Check Security & Compliance
Security is critical when dealing with sensitive data, especially in industries like healthcare, finance, and legal AI applications. Before choosing annotation software, ensure it meets security and compliance standards.
- Ensure the tool follows industry standards and privacy laws.
- Annotation software should comply with data privacy laws such as:
- GDPR (General Data Protection Regulation) for handling user data.
- HIPAA (Health Insurance Portability and Accountability Act) for medical data security.
- CCPA (California Consumer Privacy Act) for personal data protection.
- Annotation software should comply with data privacy laws such as:
- Check encryption and access control.
- Secure platforms encrypt data to prevent unauthorized access.
- Look for tools with role-based access, allowing only authorized team members to handle confidential data.
For example, a healthcare AI company working with patient X-rays must use an annotation tool that is HIPAA-compliant and offers data encryption and secure cloud storage.
Best Annotation Software in 2024
Choosing the right annotation software is important for training AI models efficiently. The best tools offer features like AI-assisted labeling, automation, collaboration, and scalability. Below is a comparison of some of the top annotation tools in 2024, highlighting their strengths and key features.
Labellerr
Labellerr is a powerful AI-powered annotation platform that automates data labeling using machine learning. It helps businesses speed up annotation tasks while maintaining high accuracy.
Key Features
- AI-assisted annotation: Uses pre-labeling to speed up data tagging.
- Automation: Reduces manual effort through smart predictions and auto-labeling.
- Collaboration tools: Supports multiple annotators working together.
- Integration: Works with AI frameworks like TensorFlow, PyTorch, AWS, and GCP.
Pricing
Labellerr offers custom pricing based on the project size and feature requirements. Businesses can contact the provider for a tailored plan.
Who Should Use It?
- AI startups and enterprises looking for automated annotation solutions.
- Companies handling large-scale datasets that require scalability and speed.
CVAT
CVAT (Computer Vision Annotation Tool) is a free, open-source platform developed by Intel. It is widely used for image and video annotation, making it a popular choice for researchers, students, and AI developers.
Key Features
- Multiple annotation types: Supports bounding boxes, polygons, key points, and semantic segmentation.
- Customizable workflows: Users can modify the platform to fit specific project needs.
- No-cost access: Since it is open-source, there are no subscription fees.
Pricing
CVAT is completely free to use, making it an excellent choice for those with budget constraints.
Who Should Use It?
- Researchers and academic institutions working on AI projects.
- Startups and small teams looking for cost-effective annotation software.
Labelbox
Labelbox is designed for large AI teams working on complex projects. It provides scalable data management, automation, and AI-powered annotation to streamline workflows.
Key Features
- Scalable platform: Manages millions of annotations efficiently.
- AI-assisted labeling: Improves speed and reduces human errors.
- Data quality assurance: Includes review workflows, anomaly detection, and inter-annotator agreement.
- API integration: Connects easily with ML frameworks and cloud storage.
Pricing
Labelbox follows a subscription-based pricing model, with custom plans for enterprise customers.
Who Should Use It?
- Large AI teams needing a robust and scalable annotation solution.
- Businesses that require integration with advanced AI workflows.
SuperAnnotate
SuperAnnotate is built for computer vision projects that need high-quality image and video annotations. It is used by companies working in autonomous vehicles, medical AI, and geospatial analysis.
Key Features
- AI-assisted annotation: Automates parts of the labeling process.
- Quality control tools: Helps ensure high annotation accuracy.
- Cloud-based collaboration: Allows teams to work together efficiently.
- Supports 2D and 3D annotations: Works with bounding boxes, polygons, and LiDAR data.
Pricing
SuperAnnotate offers custom pricing, depending on the project size and feature requirements.
Who Should Use It?
- Companies in autonomous vehicles, healthcare AI, and security.
- Businesses that need high-quality annotations for large datasets.
Future Trends in Annotation Software
The future of annotation software is rapidly evolving as AI and automation improve. New advancements will make data labeling faster, more accurate, and scalable for AI projects. Below are three key trends shaping the future of annotation software.
AI-Driven Quality Control
AI is making data labeling more accurate and efficient by automatically detecting and correcting errors. Traditionally, human reviewers had to check labeled data manually, which was time-consuming and expensive. AI-driven quality control will solve this by:
- Identifying Mistakes Automatically: AI models will scan annotations to detect mislabeling, missing labels, or incorrect boundaries.
- Confidence Scoring: The software will assign confidence scores to labeled data and flag low-confidence annotations for human review.
- Ensuring Consistency: AI will compare annotations across datasets to maintain uniform labeling standards.
Example:
In healthcare, AI-assisted quality control can help doctors verify medical image annotations, ensuring accurate disease detection in X-rays and MRIs. This will improve diagnosis and treatment planning.
Real-Time Annotation
With IoT devices, autonomous systems, and smart applications, the need for real-time annotation is increasing. Instead of labeling static datasets, AI models will label live data streams from sensors, cameras, and connected devices.
- Faster Decision-Making: AI-powered annotation tools will label incoming data instantly, allowing AI models to respond in real time.
- Enhanced Automation: Autonomous systems such as self-driving cars and industrial robots will use real-time labeling to detect objects and navigate environments safely.
- Dynamic Data Updates: AI models will continuously learn and improve as they process freshly labeled data from live sources.
Example:
In self-driving technology, real-time annotation will help AI recognize objects, pedestrians, and road signs as they appear, improving navigation safety and efficiency.
Synthetic Data Generation
AI models need vast amounts of training data, but collecting and annotating real-world data is expensive and time-consuming. Synthetic data generation is solving this issue by allowing AI to create virtual labeled datasets for training.
- Expanding Training Datasets: AI can generate synthetic images, text, and videos to train machine learning models without human annotation.
- Reducing Annotation Costs: Companies will rely less on costly manual labeling, making AI training more affordable.
- Handling Rare Scenarios: AI can simulate edge cases that rarely appear in real-world data, improving model accuracy in unexpected situations.
Example:
In manufacturing, AI-generated synthetic defect images can help train quality control models to detect faulty products even when real defective samples are scarce.
Conclusion
Choosing the right annotation software is crucial for building accurate and efficient AI models. A good tool helps businesses label data faster, maintain high quality, and scale operations efficiently.
AI-powered annotation software reduces manual effort, improves consistency, and ensures better training data for machine learning models. Whether you work with images, text, video, or audio, selecting the right tool will save time, cut costs, and improve AI performance.
Looking for a powerful annotation solution?
Try Labellerr today for AI-assisted, scalable, and high-quality data annotation!
FAQs
What is annotation software used for?
Annotation software is used to label and structure data for AI training. It helps machine learning models recognize objects, patterns, and relationships.
What features should I look for in annotation software?
Look for AI assistance, automation, collaboration tools, quality control, and security compliance.
Can AI fully automate data annotation?
AI can assist with annotation, but human review is still needed for complex cases like medical imaging or sentiment analysis.

Simplify Your Data Annotation Workflow With Proven Strategies
.png)
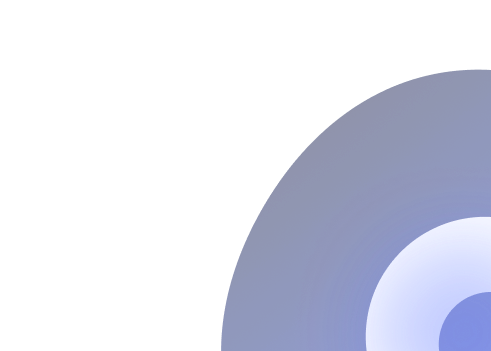