Choosing the Right Data Labeling Tool for Sports Visual Analytics: A Strategic Guide
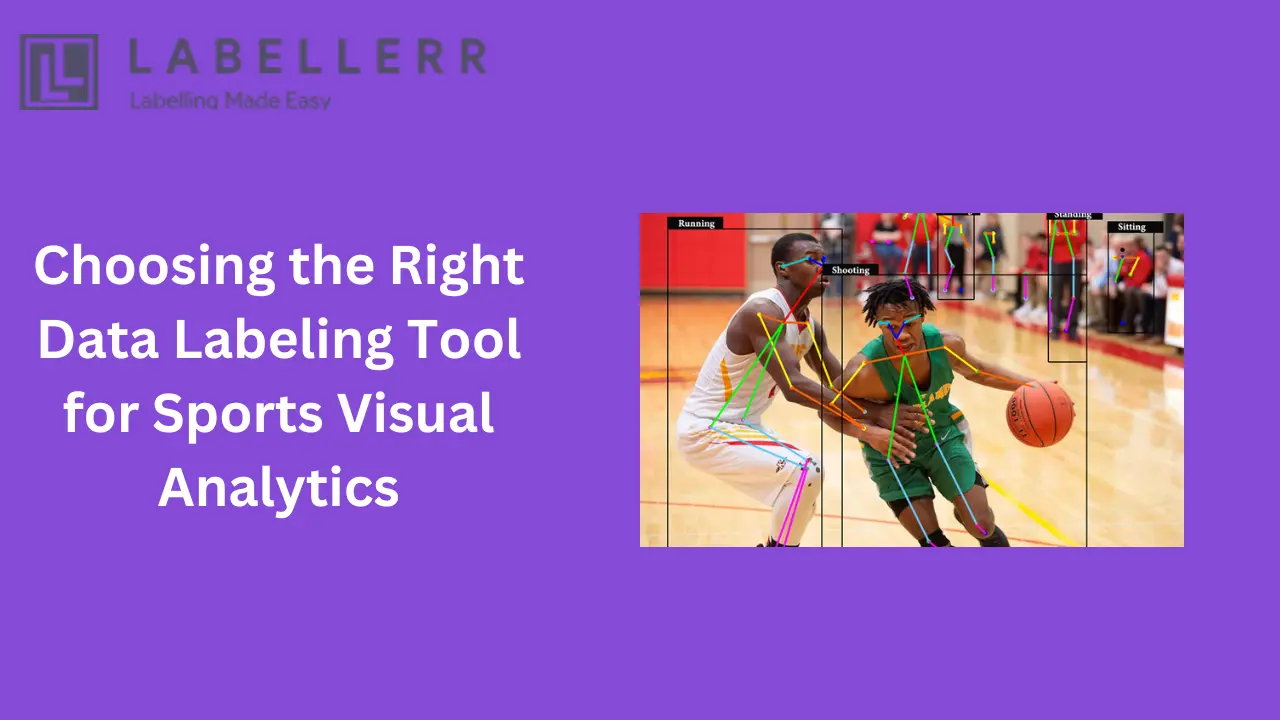
Table of Contents
- Introduction
- Understanding Your Sports Data Labeling Needs
- Key Considerations
- How Labellerr Solved Sports Data Annotation Challenge For Butterfly Positronics
- Testing and Validation
- Decision Making
- Implementation and Training
- Conclusion
- FAQ
Introduction
In the fast-evolving world of sports visual analytics, the accuracy of data labeling is paramount. Precise labeling can significantly streamline analysis, providing deeper and more actionable insights that drive performance improvements and strategic decisions. The right data labeling tool not only enhances the efficiency of this process but also ensures the quality and reliability of the derived data.
The sports industry is experiencing unprecedented growth, driven by technological advancements and a data-centric approach. Gone are the days when strategies relied solely on the intuition and expertise of coaches. Historically, athletes were fortunate if they had access to top-tier coaching.
The introduction of digital media, particularly television, allowed more athletes to study game footage and develop strategies based on their subjective understanding of what worked and what didn't. However, this approach still heavily depended on human expertise.
Today, with the advent of computer vision and big data, sports analytics has been revolutionized. Technologies can now convert video pixels into precise, detailed data on athletes' movements, providing a deeper understanding of performance dynamics.
Consider the impact of technologies like Hawk-Eye in sports. These systems transform simple viewing into deep analytical insights, refining the essence of sports analytics. For coaches and teams, this means:
- Performance: Enhanced player and team skills through precise tracking of movements, speeds, and patterns.
- Injury Prevention: Identification and mitigation of risks by monitoring biomechanics, enabling proactive measures to prevent injuries and optimize player health.
- Strategy: Real-time tactical insights that optimize team strategies and adapt game plans based on live data.
- Scouting: Precision in uncovering talent and evaluating potential recruits.
For players, the benefits include:
- Feedback: Detailed insights into performance, helping athletes refine their skills.
- Safety: Promotion of practices that protect against injuries and enhance overall well-being.
Fans and broadcasters also reap significant advantages:
- Engagement: Enhanced viewing experiences with vivid statistics and engaging visualizations such as heat maps and performance metrics during broadcasts.
- Insight: Richer narratives and a deeper understanding of the game, enrich the overall experience.
This guide aims to help you choose the right data labeling tool to maximize these benefits, ensuring your sports visual analytics projects are both effective and efficient.
Understanding Your Sports Data Labeling Needs
Before choosing the optimal data labeling tool, a crucial first step lies in thoroughly understanding your specific needs. This introspection ensures you choose a tool that is perfectly tailored to your sports visual analytics projects. Here are key factors to consider:
1. Data
- Type of Data: What kind of sports data are you analyzing? Is it video footage, still images, or sensor data collected during training sessions? Each data type might require different labeling approaches.
- Complexity of Labeling Tasks: How intricate are the labeling tasks involved? Are you simply identifying players, or do you need to capture more nuanced actions like ball trajectories, player interactions, or specific techniques?
- Data Volume: How much data do you need to label? Is it a one-time project with a limited dataset, or do you anticipate ongoing labeling needs with high data volumes?
2. Data Acquisition and Management
- Volume and Frequency: How often do you collect data? Is it sporadic, such as during specific events, or does it follow a regular schedule, like game days or training sessions? Will onboarding new users involve processing large initial datasets followed by regular updates?
- Variety of Formats: In what formats is your data collected? Be prepared for video files, image sequences, and potentially structured sensor data.
- Data Sources: Where will your data reside? Ensure your chosen tool integrates seamlessly with your data sources, whether it's cloud storage (e.g., S3, Azure),
3. Data Characteristics
- File Size and Quality: Consider the file sizes and overall quality of your data. Factors like image resolution and video compression can impact the labeling process.
- Quality of Labels: How detailed do your labels need to be? Are you simply marking player positions, or do you require more granular labeling of specific actions, gestures, or interactions?
- Temporal Context: Does the labeling process require understanding the temporal sequence of events? For instance, tracking player movements throughout a play or identifying specific moments within a game.
4. Domain Expertise:
- Required Knowledge: Certain labeling tasks might necessitate domain-specific knowledge of the sport being analyzed. Assess the level of expertise needed by your labeling team to ensure accurate results.
By carefully considering these factors, you gain a clear understanding of your specific data labeling requirements. This self-awareness empowers you to evaluate data labeling tools effectively and select the one that perfectly aligns with your sports visual analytics projects, ultimately maximizing the value you extract from your data.
Key Considerations
Selecting the correct data labeling tool for your project requires careful consideration of several crucial factors:
- Accuracy and Consistency: Explore how each tool ensures accurate and consistent labeling, especially for intricate sports actions or events.
- Scalability: Assess the scalability of the tool to handle increasing volumes of data as your projects grow. However, the scalability of the tool isn't just about handling volumes; it is also about the vendor's process and its ability to ensure accuracy in sporadic situations.
- Customization: Look for tools that allow customization to adapt to the unique labeling requirements of sports analytics, such as recognizing player movements, game strategies, or player tracking.
- Integration: Consider how well the labeling tool integrates with your existing analytics workflow or data lakes, including compatibility with data analysis platforms and visualization tools.
- Cost and ROI: Evaluate the cost-effectiveness of each solution, considering both upfront expenses and long-term ROI in terms of time saved and insights gained.
How Labellerr Solved Sports Data Annotation Challenge For Butterfly Positronics
Tyler, CEO - Butterfly Positronics was looking for an easy and faster solution for his sports image segmentation task, though after taking a trial of several tools, he found none of them were providing an easy, collaborative UI where he could validate the quality of annotations in the minimum time possible.
Impressed by Labellerr's user-friendliness and efficient workflow, significantly improved his data annotation process. The platform's intuitive interface made project setup and data labeling a smooth experience. You can read the entire case study here.
Testing and Validation
Choosing the right data labeling tool goes beyond simply evaluating features. Testing and validation are critical steps to ensure the chosen tool delivers the accuracy and efficiency you require.
Here's a systematic approach:
- Pilot Project: Develop a small-scale pilot project using representative sports data. This allows you to test each shortlisted tool in a real-world scenario specific to your needs.
- Evaluate Performance: Focus on key metrics like labeling accuracy, consistency, and completion time. Labellerr offers self-trial options with access to relevant sports data, allowing you to directly test capabilities with customer's specific use case.
- Validation: Beyond self-trials, consider conducting a formal validation process. This might involve assigning the same labeling task to different tools or human annotators to compare results and identify discrepancies.
Benefits of a Robust Testing Strategy:
A thorough testing and validation process offers several advantages:
- Reduced Risk: Identify and eliminate tools that don't meet your accuracy or efficiency requirements, minimizing the risk of investing in an unsuitable solution.
- Informed Decisions: Gain empirical data on each tool's performance, allowing you to make informed decisions based on your specific needs and project goals.
- Streamlined Workflow: By validating tool capabilities, you can identify potential bottlenecks and optimize your labeling workflow for maximum efficiency.
Labellerr as an Example:
As mentioned earlier, Labellerr provides self-trial options that allow you to connect your cloud-based sports data. This facilitates direct testing of the platform with your specific data formats and labeling requirements. Additionally, features like annotation validation and active learning contribute to overall labeling accuracy and efficiency.
Remember, the ideal data labeling tool should not only boast impressive features but also demonstrate its value through a robust testing and validation process.
Decision Making
Making the final decision on a data labeling tool for sports visual analytics involves a comprehensive evaluation of several critical factors. ML teams usually base their decision on three primary criteria that align with their specific needs and long-term objectives:
Accuracy or Quality Achieved in the POC
The primary consideration is the accuracy and quality of the results obtained during the Proof of Concept (POC). The tool must:
- Deliver precise and consistent labeling results, especially for intricate sports actions.
- Demonstrate robust performance across different scenarios and datasets.
- Show potential for maintaining high quality as the project scales.
Time Taken to Achieve Results
Although the time taken to achieve results in a POC is important, it may not be a decisive factor since POCs are typically small-scale. However, it's essential to consider:
- The tool's performance and speed in larger, more demanding projects.
- The potential for automation capabilities to significantly boost efficiency. For instance, Labellerr's automation can provide a 99x increase in speed, ensuring quick turnaround times for large datasets.
Costs
Cost considerations are crucial and should be evaluated based on the visibility and clarity of your short-term and long-term requirements:
- Short-Term Projects: If your project involves a one-time annotation of a few hundred images, compare the price per image across vendors.
- Long-Term Projects: For projects involving thousands to millions of images over the next 12 months, consider if the vendor offers processes or technologies that incrementally reduce the price per file. Automation capabilities, such as those offered by Labellerr, can also help reduce costs significantly.
Additional Factors
- Data Security and Compliance: Ensure the tool adheres to relevant data security standards and compliance requirements, such as SOC2 Type 2 certification or strict data security guidelines. The tool should also have technical capabilities to redact sensitive information to maintain user privacy.
- Quality Assurance Capabilities: The tool should offer quality assurance features tailored to sports-related use cases. This includes dedicated filters for specific rules, such as identifying injuries, tracking player speeds, or monitoring ball possession. The ability to filter data and validate for anomalies in sports contexts, like player position correlation with certain areas of the field, is also valuable.
- Team and Dataset Management: The tool should support managing multiple teams and datasets, allowing for the reuse of labeled data across different teams and projects.
- Testimonials and Case Studies: Look for testimonials and case studies from other companies in similar domains that have successfully used the tool. Ask the vendor to present past case studies or testimonials to gain insights into their tool's effectiveness and reliability.
Implementation and Training
Implementing the chosen data labeling tool into your sports visual analytics workflow involves several best practices to ensure a smooth and effective transition.
First, it's essential to integrate the tool seamlessly with your existing systems, including data storage, processing, and analytics platforms. This might involve configuring APIs, setting up data pipelines, and ensuring compatibility with your current software stack. Clear documentation and support from the vendor can significantly ease this process.
Training team members is equally crucial for maximizing the tool's potential. Comprehensive training programs should be developed to familiarize users with the tool's features, functionalities, and best practices.
This includes hands-on sessions, instructional videos, and ongoing support to address any challenges that may arise. Effective training ensures that your team can use the tool efficiently, reducing the risk of errors and improving the overall quality of the labeled data.
Conclusion
Selecting the right data labeling tool is critical for success, with numerous solutions available in the market, it's essential to clearly understand your key requirements and the challenges you might face.
Define what you need from a data labeling tool, including accuracy, scalability, customization, and integration capabilities. Examine the vendor's past work and case studies, focusing on those most relevant to your use case, to gain valuable insights into the tool's real-world performance and reliability.
Conducting a small-scale Proof of Concept (POC) is a practical way to evaluate the tool's performance in real-world scenarios while allowing the vendor to understand your specific challenges and expectations.
Tools like Labellerr, with their automation capabilities, cloud integration, and flexibility, can offer significant advantages however, finally the choice should be based on a thorough evaluation of your unique needs and circumstances.
Selecting the right tool is not just about immediate needs but also about ensuring long-term success and scalability, optimizing your data labeling processes, and driving impactful insights in the world of sports visual analytics.
FAQ
Q1) What is data labeling, and why is it important in sports visual analytics?
Data labeling involves annotating or tagging data (such as images or videos) to make it understandable for machine learning models.
In sports visual analytics, accurate data labeling is crucial for extracting meaningful insights from video footage, such as player movements, game strategies, and performance metrics.
This helps in creating customized training plans, enhancing injury prevention strategies, and providing engaging content for fans.
Q2) What are the key factors to consider when selecting a data labeling tool for sports analytics?
When choosing a data labeling tool, consider the following factors:
- Accuracy and Consistency: The tool should deliver precise and consistent labeling results.
- Scalability: It should handle increasing data volumes without compromising performance.
- Customization: The ability to adapt to specific sports analytics requirements, such as player tracking and game strategy recognition.
- Integration: Compatibility with your existing analytics workflow, data lakes, and visualization tools.
- Cost and ROI: Evaluate the cost-effectiveness, considering both upfront expenses and long-term benefits.
Q3) How can I test the effectiveness of a data labeling tool?
Conduct a small-scale Proof of Concept (POC) to evaluate the tool’s performance in real-world scenarios. This allows you to assess its functionality, ease of use, and the accuracy and efficiency of labeling results. Many tools, like Labellerr, offer self-trial options and integration with your cloud data, facilitating a thorough evaluation with realistic datasets.
Q4) Why is scalability important in a data labeling tool?
Scalability ensures that the tool can manage increasing data volumes as your projects grow. It’s not just about handling more data but also maintaining accuracy and performance in various situations. A scalable tool can adapt to your evolving needs, whether you're dealing with a few hundred images or millions over time.
Call to Action
I invite readers to share their experiences with data labeling tools in sports analytics. Whether you've implemented a tool successfully or faced challenges, your insights can help others navigate their journey. Connect to further discuss recommendations or explore how to optimize your sports visual analytics projects. Let's collaborate to leverage the power of data labeling for impactful insights in sports analytics.
Puneet is co-founder and CEO at Labellerr.
He is working towards the vision to remove the high-quality data scarcity for model training. He loves to discuss and write about new developments in the field of AI. His areas of expertise are computer vision, data pipeline, and model evaluation for LLMs.
Connect with him over Linkedin or write an email at puneet.jindal@labellerr.com
Book our demo with one of our product specialist
Book a Demo