How to Choose the Right Image Labeling Tool in 2025
Discover how to select the perfect image labeling tool in 2025. Learn about essential features, top tools, and best practices to ensure accurate, efficient labeling for your AI projects. Make informed decisions and boost your model's performance with the right tool.
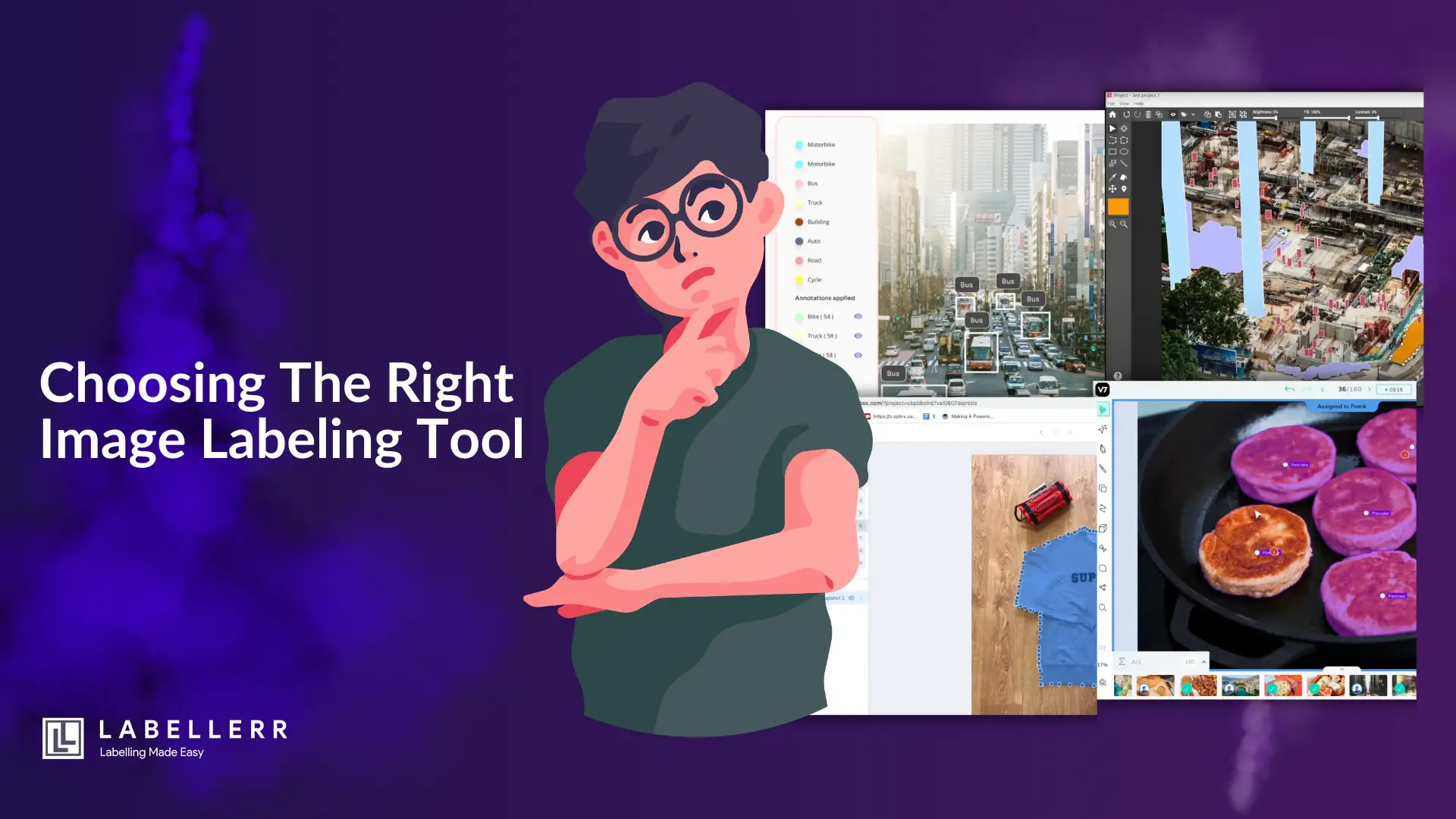
Did you know that a report from stated data preparation, including image labeling, accounts for up to 80% of the time spent on AI projects?
This highlights the critical role image labeling tool play in building accurate and reliable AI models.
These tools are essential for creating high-quality datasets, which are the foundation of every successful AI project.
However, among image labeling tools available it can be challenging choose one. Teams often face issues like compatibility with their existing workflows, scalability for large datasets, and the cost of implementing these tools.
Without the right image labeling tool, these challenges can slow down projects, increase costs, and impact the quality of the data, ultimately affecting the performance of AI models.
This article will guide you through the process of evaluating and selecting the best image labeling tool for your needs.
By the end, you’ll have a clear understanding of what to look for and how to choose a tool that aligns with your project goals and requirements.
Table of Contents
- Key Features to Look for in an Image Labeling Tool
- Tips for Making the Final Decision
- Conclusion
- FAQs
Key Features to Look for in an Image Labeling Tool
When choosing an image labeling tool, it’s important to evaluate its features to ensure it meets your project’s needs. Here are the key features to look for:
Annotation Types Supported
The tool should support various annotation types to align with different AI use cases:
- Bounding Boxes: For object detection, such as identifying cars or pedestrians in autonomous driving.
- Polygons: For irregularly shaped objects, like buildings in satellite imagery or leaves in agriculture.
- Semantic Segmentation: For assigning labels to every pixel in an image, useful in medical imaging or robotics.
- Key Points: For marking specific locations, like facial landmarks or points on plant stems.
- 3D Cuboids: For labeling objects in 3D, such as depth perception in autonomous vehicles.
Ensure the tool offers flexibility to use the most appropriate annotation type for your project.
Automation Capabilities
Look for image labeling tool with automation features to speed up the annotation process:
- Auto-labeling: Automatically labels objects based on pre-defined criteria.
- Pre-labeling: Uses AI to generate initial labels for manual review and adjustments.
- Smart Predictions: Suggests annotations based on patterns in existing data.
These features save time and reduce manual effort, especially for large datasets.
Quality Assurance Mechanisms
High-quality annotations are critical for AI model performance. Choose an image labeling tool with features that ensure consistent and accurate labeling:
- Confidence Scoring: Flags low-confidence annotations for review.
- Inter-Annotator Agreement: Ensures consistency across different annotators.
- Anomaly Detection: Identifies errors or inconsistencies in the annotations.
The tool should also allow multiple reviewers to validate annotations and maintain high data quality.
Scalability
Select an image labeling tool that can handle your current dataset size and scale for future needs:
- Cloud-Based Solutions: Allow you to manage large datasets without needing additional hardware.
- On-Premises Solutions: Suitable for projects requiring local storage due to security or compliance reasons.
Scalability is crucial for long-term projects or datasets that grow over time.
Collaboration Features
Efficient teamwork is crucial for large annotation projects. Look for collaboration-friendly features in an image labeling tool:
- Real-Time Feedback: Allows annotators and reviewers to communicate and correct errors quickly.
- Guideline Sharing: Ensures everyone follows consistent labeling rules.
Collaboration features improve efficiency and ensure consistent annotations across large teams.
Integration with Machine Learning Pipelines
A good image labeling tool should integrate easily with your machine learning workflows:
- Compatibility with Formats: Supports popular data formats like COCO, YOLO, and Pascal VOC.
- Cloud Integration: Offers direct connections to platforms like AWS, GCP, or Azure for seamless data transfer.
This integration reduces friction and speeds up the transition from annotation to model training.
Cost and Licensing Models
Evaluate the tool’s cost and licensing options based on your budget:
- Free Tools: May lack advanced features but are suitable for small projects or beginners.
- Paid Tools: Offer advanced features, scalability, and support.
- Subscription-Based: Regular payments for continued access.
- One-Time Purchase: A fixed fee for permanent access.
- Usage-Based Pricing: Charges based on the amount of data or time used.
Choose a pricing model that fits your project size and long-term requirements.
Tips for Making the Final Decision
Choosing the right image labeling tool, requires careful consideration of your project’s needs and priorities. Here are some tips to help you make the best choice:
Balance Cost, Features, and Scalability
Consider your budget, but don’t focus only on the cost. Balance it with the features the tool offers and its ability to scale as your project grows.
- Cost: Ensure the tool fits your budget while providing value for the price. Look for flexible pricing models, such as pay-as-you-go or subscriptions.
- Features: Prioritize essential features like automation, multiple annotation types, and integration with ML pipelines.
- Scalability: Choose a tool that can handle both your current dataset and potential future expansions, especially if you plan to work on larger projects or new use cases.
Prioritize Tools That Save Time and Improve Accuracy
Efficiency and accuracy are key to successful AI projects. Focus on tools that:
- Save Time: Look for automation features like pre-labeling and smart predictions to reduce manual effort.
- Ensure Accuracy: Choose tools with quality assurance mechanisms like confidence scoring and anomaly detection to maintain data consistency.
These features will streamline your workflows and enhance the reliability of your AI model.
Consider Vendor Support and Community Resources
The level of support you receive from the tool’s vendor can greatly impact your experience:
- Vendor Support: Ensure the provider offers responsive customer support, training resources, and troubleshooting assistance. This is especially important if you’re using the tool for large or complex projects.
- Community Resources: Check for an active user community, forums, or online tutorials. These resources can provide valuable tips and solutions from other users who’ve faced similar challenges.
Strong support and community engagement ensure a smoother experience and help you maximize the tool’s potential.
Conclusion
Choosing the right image labeling tool is essential for the success of any AI project. Start by understanding your project’s needs, including the type of annotations required, dataset size, and accuracy goals.
Evaluate tools based on their features, such as automation, scalability, and integration with machine learning pipelines. Don’t forget to involve your team and test tools through trials to make an informed decision.
By selecting a tool that saves time, improves accuracy, and fits your budget, you can streamline your data annotation workflow and create high-quality datasets that enhance AI model performance.
Ready to take your data annotation process to the next level? Explore tools like Labellerr to experience efficient, accurate, and scalable image labeling solutions tailored to your needs.
FAQs
1. What are the challenges while choosing the right image labeling tool?
Choosing the right image labeling tool can be challenging due to the vast number of options available, varying features, and different levels of automation and integration. Ensuring the tool meets your project's specific needs, like handling complex datasets or providing quality control features, can add to the difficulty.
2. Should you go with a free or paid image labeling tool?
The decision between a free or paid image labeling tool depends on your project's scale and requirements. Free tools are often suitable for smaller projects or those with limited budgets. However, paid tools usually offer more advanced features, better support, and scalability, making them ideal for larger, more complex projects.
3. What should you look for when choosing an image labeling tool?
When choosing an image labeling tool, look for key features such as ease of use, automation capabilities, support for different annotation types, quality control mechanisms, integration options with your existing workflow, and scalability to handle large datasets. These factors ensure efficient and accurate labeling processes
Book our demo with one of our product specialist
Book a Demo