How to Choose the Right Data Labeling Company in 2025
Learn how to choose the right data labeling company by understanding key factors like quality, scalability, turnaround time, pricing, and industry expertise. Find the perfect partner to ensure accurate and efficient data labeling for your AI and machine learning projects.
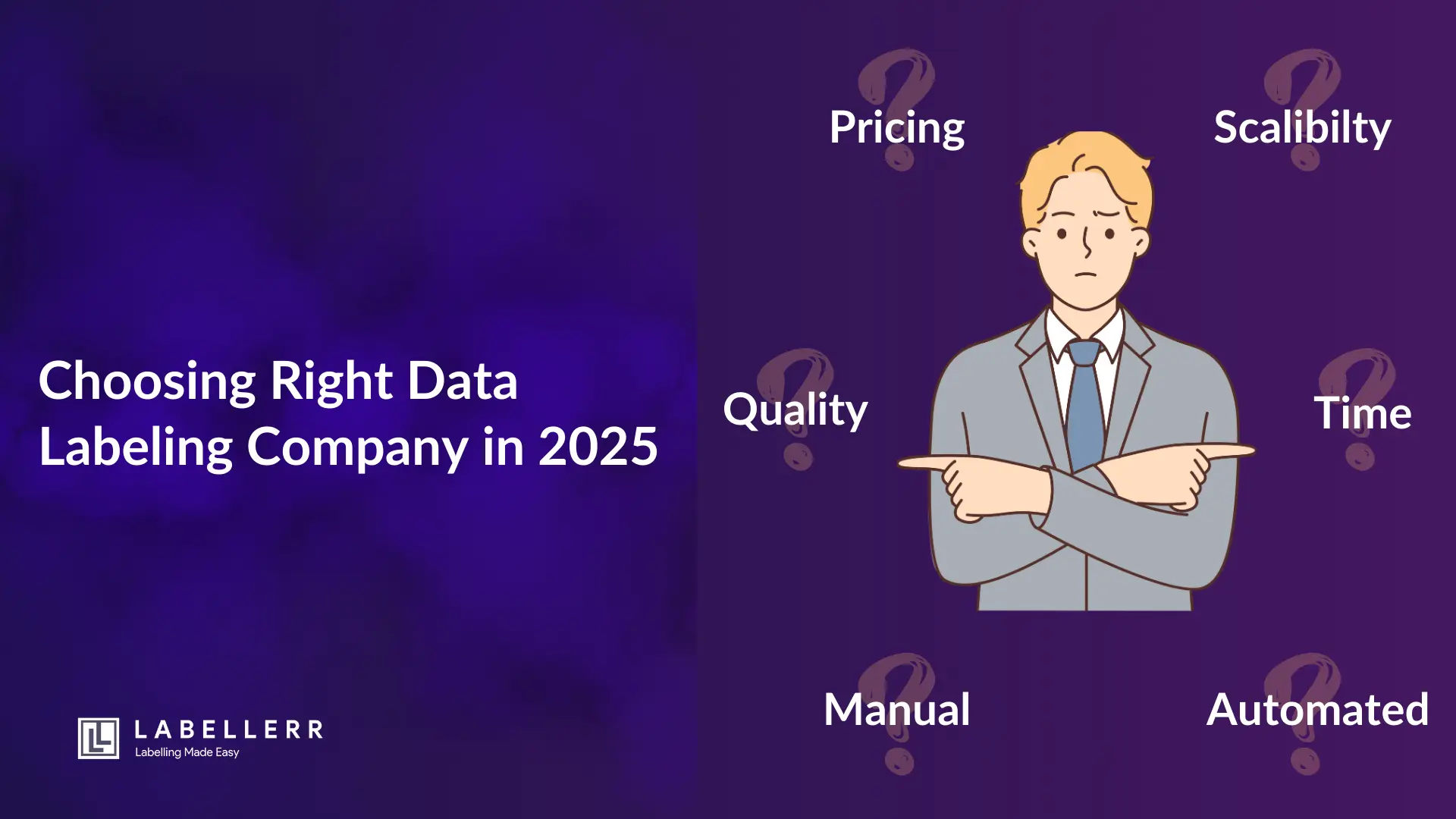
"Did you know that 70% of data labeling is outsourced to countries like India, China, and other developing regions due to their affordable labor costs?"
This fact shows how critical data labeling has become in the global AI industry, as companies seek reliable and cost-effective solutions to prepare high-quality datasets.
For businesses building AI models, choosing the right data labeling company is one of the most important decisions.
However, this process comes with its own challenges. Quality is a major concern because errors in labeling can lead to poor model performance.
Scalability is another hurdle, especially for projects with large datasets or tight deadlines. Finally, cost is a factor, as businesses need to balance affordability with the need for accurate and consistent data.
This guide is designed to help businesses navigate these challenges and make informed decisions when selecting a data labeling company. We’ll cover key factors to consider, such as quality assurance, technology, scalability, and pricing.
By the end, you’ll have a clear understanding of how to evaluate and choose a data labeling company that aligns with your project goals and ensures success.
The Role of Data Labeling Companies in AI Development
Data labeling companies play a crucial role in AI development by creating high-quality labeled datasets. These labeled datasets are the foundation of machine learning models, enabling them to learn patterns, recognize objects, and make accurate predictions.
Without proper labeling, AI models struggle to perform effectively in real-world applications.
What Do Data Labeling Companies Do?
Data labeling companies specialize in annotating raw data such as images, videos, text, or audio. They use advanced tools and techniques to add annotations like bounding boxes, polygons, or text tags, making the data usable for training AI models.
These companies ensure that the annotations are accurate, consistent, and aligned with the specific requirements of the AI project.
For example, in an autonomous vehicle project, a data labeling company might annotate road scenes by marking vehicles, pedestrians, traffic signs, and lane markings. These labeled datasets allow the AI model to learn and navigate real-world scenarios safely.
Why Partner with a Data Labeling Company?
- Expertise in Handling Complex Datasets:
Data labeling companies have teams of skilled annotators who are trained to handle complex and large-scale datasets. They understand domain-specific requirements, making them ideal for projects in industries like healthcare, retail, or autonomous vehicles. - Access to Advanced Annotation Tools:
These companies use state-of-the-art annotation platforms with features like AI-assisted labeling, automated quality checks, and seamless integration with machine learning workflows.This technology speeds up the labeling process and ensures consistent quality. - Scalability:
Data labeling companies are equipped to handle projects of any size. Whether you need to label a small dataset or millions of images, they can scale their resources to meet your needs. This flexibility is especially useful for startups and businesses that need to adapt quickly to project changes or market demands.
Key Benefits of Partnering with a Data Labeling Company
- Improved Model Performance:
High-quality labeled data ensures that AI models learn accurately and deliver reliable results. Proper annotations reduce errors during training, leading to better model performance in real-world applications. - Faster Project Timelines:
By outsourcing data labeling to experts, businesses can focus on other critical tasks like model development and deployment. This speeds up the overall timeline and allows companies to bring their products to market faster. - Reduced In-House Workload:
Partnering with a data labeling company eliminates the need for businesses to manage large teams of annotators in-house. This reduces operational complexity and lets internal teams focus on core activities.
Data labeling companies are essential partners in AI development, providing the expertise, tools, and scalability needed to create high-quality datasets.
By outsourcing to a reliable partner, businesses can improve their AI models, meet tight deadlines, and reduce internal workload, paving the way for successful AI projects.
Key Criteria for Choosing the Right Data Labeling Company
Choosing the right data labeling company is a critical step in ensuring the success of your AI projects. The quality and reliability of labeled data directly impact the performance of your AI model.
The right partner ensures that your data is labeled accurately, consistently, and efficiently. Here are the key criteria to consider:
Quality Assurance Mechanisms
Why Accuracy Matters:
Accuracy is the foundation of high-quality labeled data. Without it, AI models may produce incorrect results, leading to poor performance. A reliable data labeling company should prioritize quality assurance through well-defined processes.
Key Processes for Quality Assurance:
- Inter-Annotator Agreement: This process involves multiple annotators working on the same data sample to ensure consistency. The higher the agreement between annotators, the more reliable the labels.
- Anomaly Detection: This feature automatically identifies inconsistencies or errors in the annotations, allowing teams to fix them before they affect the model.
- Confidence Scoring: Confidence scores help prioritize data for review by flagging low-confidence labels. This ensures high-quality annotations while minimizing errors.
Scalability and Flexibility
A good data labeling company should have the capacity to handle both small-scale and large-scale projects. AI projects often involve massive datasets, and the ability to scale efficiently is essential.
- Handling Large Datasets:
As your AI project grows, so does the volume of data that needs to be labeled. A good data labeling company should be able to scale resources to meet your needs, whether it’s a few hundred images or millions of data points. - Support for Various Data Types:
They should be able to handle diverse data types, including:- Images: For object detection or segmentation.
- Videos: For tasks like action recognition or object tracking.
- Text: For natural language processing tasks like sentiment analysis.
- Audio: For speech recognition or transcription.
- Meeting Tight Deadlines:
Flexibility to adapt to your project timeline is crucial. The company should be able to scale up resources when needed to meet deadlines without delays.
Expertise and Industry Knowledge
Different industries have unique data annotation needs. A data labeling company with experience in your domain will better understand your requirements and deliver tailored solutions.
- Experience in Specific Industries:
Look for companies with proven experience in your industry. For example:- Healthcare: Annotating medical images like X-rays or MRIs for disease detection.
- Autonomous Vehicles: Labeling road scenes with objects like vehicles, pedestrians, and traffic signs.
- Retail: Tagging product images for recommendation systems or visual search engines.
- Handling Domain-Specific Needs:
The company should have teams skilled in understanding and labeling complex datasets specific to your industry. For example, labeling microscopic cells in healthcare requires more precision than tagging objects in a retail catalog.
Technology and Tools
The technology and tools a data labeling company uses are critical for ensuring efficiency, accuracy, and seamless integration into your AI development process. Advanced tools can significantly reduce manual effort, save time, and improve annotation quality.
- AI-Assisted Annotation and Automation:
Data labeling companies that use AI-assisted tools automate repetitive and time-consuming tasks, such as drawing bounding boxes, segmenting objects, or labeling key points. Automation enables annotators to focus on more complex cases and improves overall efficiency.- Example: AI-assisted pre-labeling suggests initial annotations, which annotators can quickly review and adjust. This reduces manual effort by up to 70%.
- Advanced Features: Tools with smart predictions, auto-labeling, and dynamic adjustment help reduce errors and speed up the process.
- Integration with Machine Learning (ML) Pipelines:
A good data labeling company ensures that its tools integrate seamlessly with your existing workflows. This includes the ability to export labeled data in formats compatible with machine learning models and cloud platforms.- Supported Cloud Platforms: Integration with AWS, Google Cloud, Azure, or other services makes data processing efficient and supports faster model training.
- Direct Integration: Tools that connect directly with your ML pipeline reduce the need for manual uploads or conversions, saving time.
- Compatibility with Popular Data Formats:
The tools must support common data formats to ensure flexibility and usability across different applications:- COCO: Ideal for object detection and segmentation.
- YOLO: Widely used for real-time object detection.
- Pascal VOC: Popular for classification and detection tasks.
Compatibility with these formats ensures that the labeled data fits seamlessly into your AI workflows.
Security and Compliance
Data security is a top priority, especially for industries like healthcare, finance, and government, where sensitive or personal information is often involved. A reliable data labeling company must prioritize privacy, comply with regulations, and protect your data from breaches.
- Adherence to Regulations:
A trustworthy company ensures compliance with global and industry-specific data protection laws, including:- GDPR (General Data Protection Regulation): Protects personal data in the EU.
- HIPAA (Health Insurance Portability and Accountability Act): Ensures confidentiality of healthcare data in the US.
- CCPA (California Consumer Privacy Act): Governs data privacy in California.
Adherence to these regulations ensures your data is handled responsibly and minimizes legal risks.
- Secure Data Transfer and Storage:
Companies should use robust security measures to protect your data during transfer and storage:- Encrypted Transfers: Tools like VPNs and end-to-end encryption protect data in transit.
- Secure Storage: Data is stored on protected servers with restricted access to prevent unauthorized breaches.
- Access Controls: Role-based access ensures that only authorized personnel can view or edit data.
Cost and Pricing Models
Understanding the pricing model of a data labeling company helps you manage your budget effectively while ensuring quality and reliability. Different pricing models cater to various project sizes and requirements.
- Common Pricing Structures:
- Pay-As-You-Go: You pay based on the volume of labeled data. This is ideal for variable or smaller projects.
- Subscription-Based: Fixed costs for ongoing services, suitable for companies needing long-term annotation.
- Usage-Based Pricing: Charges based on time or specific tasks completed. This model gives flexibility for specific workloads.
- Hidden Costs to Watch For:
Some companies may charge extra for services like additional reviews, complex annotations, or expedited delivery. Clarify all potential costs upfront to avoid surprises. - Balancing Cost with Quality:
While it’s tempting to choose the cheapest option, sacrificing quality can lead to errors in annotations, which can cost more in the long run. Invest in a company that offers transparent pricing and guarantees consistent, high-quality output.
Customer Support and Communication
Strong customer support and clear communication are essential for smooth project execution. A good data labeling company should provide responsive service and keep you updated throughout the project.
- Dedicated Project Managers:
Having a project manager as a single point of contact ensures that your project is well-coordinated. They can provide regular updates, address your concerns, and manage deadlines effectively. - Clear Communication Channels:
Companies should offer multiple ways to stay in touch, including:- Emails and Chat: For quick updates and minor queries.
- Video Calls: For detailed discussions and progress reviews.
- Project Management Tools: Platforms like Slack, Trello, or Asana to track progress in real-time.
- Real-Time Feedback Loops:
Companies that encourage ongoing feedback during the annotation process ensure that your input is incorporated quickly. This minimizes errors and keeps the project aligned with your goals.
Common Challenges in Choosing a Data Labeling Company
Selecting the right data labeling company can be challenging, as several factors can affect the success of your AI project. Here are some common issues that businesses face when partnering with a data labeling company:
Inconsistent Quality
One of the biggest challenges is ensuring consistent labeling quality. Poor labeling can severely impact the performance of AI models.
- What Happens with Poor Labeling?
Inconsistent or incorrect labels confuse AI models during training. For instance, if a self-driving car model is trained with mislabeled pedestrians or vehicles, it could lead to dangerous mistakes in real-world scenarios. - Why It Happens:
- Lack of clear guidelines for annotators.
- Inexperienced or untrained labeling teams.
- Over-reliance on manual processes without proper quality checks.
- Impact:
Poor labeling leads to inaccurate predictions, higher error rates, and increased costs as businesses must re-label the data or retrain models.
Hidden Costs
Hidden costs are another major concern when working with data labeling companies. These can strain budgets and create unexpected financial pressures.
- Common Hidden Costs:
- Extra charges for revising annotations after the first delivery.
- Higher fees for complex labeling tasks like 3D annotations or edge cases.
- Costs for expedited project timelines.
- Why It’s a Problem:
Businesses often choose a company based on initial low pricing but later discover these hidden costs. This disrupts budgeting and may force companies to compromise on quality. - Solution:
Look for companies with transparent pricing models that clearly define costs upfront, including revisions, complexity surcharges, or additional services.
Lack of Scalability
Not all data labeling companies can handle the demands of large or growing datasets, which can slow down projects.
- Why Scalability Matters:
AI projects often involve thousands or millions of data points. A company that lacks the infrastructure or resources to scale can create bottlenecks in your workflow. - Signs of Scalability Issues:
- Delayed delivery due to insufficient staff or tools.
- Inability to adapt to project changes, such as increased data volume.
- Impact:
A lack of scalability can lead to missed deadlines, increased costs, and lost opportunities to launch products on time. - Solution:
Choose a company with a proven ability to handle large-scale projects, including cloud-based solutions and flexible workflows.
Communication Barriers
Effective communication is crucial for successful collaboration. Poor communication can lead to delays, misunderstandings, and unsatisfactory results.
- Common Issues:
- Lack of regular updates on project progress.
- Vague or unclear responses to queries.
- Limited access to a dedicated point of contact.
- Impact:
Communication breakdowns can result in missed deadlines, low-quality outputs, and increased frustration for your team. - Solution:
Select a company that offers:- Dedicated Project Managers: A single point of contact ensures clear and efficient communication.
- Regular Updates: Frequent progress reports keep you informed.
- Collaboration Tools: Platforms like Trello, Slack, or Asana help streamline communication and feedback.
Questions to Ask Before Partnering with a Data Labeling Company
Before partnering with a data labeling company, it’s essential to ask the right questions to ensure they can meet your project’s requirements. Here are the key questions to ask and why they matter:
What is your process for ensuring quality and consistency?
Quality and consistency are critical for the success of your AI model. This question helps you understand how the company maintains high standards in their labeling process.
- What to Look For:
- Use of quality assurance mechanisms like confidence scoring, inter-annotator agreement, and anomaly detection.
- A clear review process to double-check annotations and resolve errors.
- Experienced annotators trained in handling complex datasets.
- Why It Matters:
Inconsistent labeling can lead to poor model performance, requiring costly rework and delays. A company with robust quality assurance processes ensures accurate and reliable annotations.
Can you handle projects of my size and complexity?
AI projects often involve large datasets or specific labeling requirements. This question ensures the company can scale to your needs and handle complex tasks.
- What to Look For:
- Ability to process large datasets quickly without compromising quality.
- Experience with various data types, such as images, videos, text, and audio.
- Flexibility to adapt to changes in project scope or timelines.
- Why It Matters:
A company that cannot scale effectively or manage complex requirements may cause delays or deliver subpar results. Ensure they have the infrastructure and expertise to match your project’s demands.
What industries or data types do you specialize in?
Every industry has unique data labeling requirements. This question helps you evaluate the company’s expertise in your specific domain.
- What to Look For:
- Experience in industries like healthcare, retail, autonomous vehicles, or agriculture.
- Familiarity with domain-specific data challenges, such as labeling medical images or segmenting road scenes for autonomous vehicles.
- Why It Matters:
A company with industry expertise understands your challenges and can deliver tailored solutions. This reduces the need for extensive rework and ensures the labeled data meets your project goals.
How do you ensure data security and compliance?
Data privacy and security are essential, especially when working with sensitive information like medical records or financial data. This question helps you assess the company’s commitment to protecting your data.
- What to Look For:
- Compliance with regulations like GDPR, HIPAA, or CCPA.
- Secure data transfer methods, such as encrypted file sharing.
- Storage practices that protect data from unauthorized access or breaches.
- Why It Matters:
Partnering with a company that doesn’t prioritize security can put your data and reputation at risk. Ensure they have robust policies and practices in place to safeguard your information.
What is your pricing structure, and are there any hidden costs?
Understanding the pricing structure upfront helps you budget effectively and avoid unexpected expenses.
- What to Look For:
- Transparent pricing models, such as pay-as-you-go, subscription-based, or usage-based options.
- Clarification on additional costs for complex annotations, revisions, or expedited timelines.
- A detailed cost breakdown for different services.
- Why It Matters:
Hidden costs can strain your budget and lead to financial surprises mid-project. A clear pricing structure ensures you get the most value without compromising quality.
Case Studies
Case studies provide real-world examples of how data labeling companies like Labellerr help businesses overcome challenges and achieve their goals. Let’s explore a successful partnership between Labellerr and Oishii, focusing on their vertical farming use case.
Case Study: Advancing Vertical Farming with AI
Oishii, a leader in vertical farming, specializes in growing strawberries using advanced techniques in controlled environments.
To improve the efficiency of their harvesting process, Oishii developed an AI-powered autonomous robot capable of detecting the ripeness of strawberries and identifying the precise stem-cutting points.
However, for their AI model to perform accurately, Oishii needed high-quality annotated data. This involved labeling thousands of strawberry images, including segments for berries, stems, and calyx, in diverse lighting conditions and growth stages.
Challenges Faced by Oishii
- Data Complexity:
Oishii’s project required precise segmentation of strawberries, stems, and calyx. Variations in ripeness, overlapping objects, and inconsistent lighting made labeling complex. - Volume of Data:
The project involved thousands of images, requiring detailed annotations for each strawberry in the dataset. Managing such a large dataset manually was impractical. - Accuracy Requirements:
The AI model relied on extremely accurate annotations to ensure the robot could make precise cuts without damaging the fruit or plant. Errors in labeling could lead to inefficient harvesting or wastage.
How Labellerr Helped?
Labellerr addressed Oishii’s challenges by providing a tailored solution that combined advanced technology with efficient workflows:
- Customized Annotation Tools:
- Labellerr used a combination of bounding boxes, polygons, and key points to label strawberries, stems, and calyx with precision.
- The platform’s AI-assisted features pre-labeled images, allowing annotators to focus on fine-tuning complex segments.
- AI-Assisted Annotation:
- Automation reduced the time required for repetitive tasks.
- Confidence scoring and anomaly detection ensured consistent and accurate annotations across the dataset.
- Efficient Workflow Integration:
- Labellerr integrated seamlessly with Oishii’s existing AI pipelines, allowing easy data upload and export in formats compatible with their machine learning model.
- The platform supported collaboration between Oishii’s internal teams and external annotators, ensuring quality and consistency.
Results and Impact
- Improved Annotation Efficiency:
- Labellerr’s tools helped reduce annotation time by 70% compared to manual methods.
- Tasks that previously took weeks were completed in days, allowing Oishii to accelerate their model training.
- Enhanced Model Performance:
- Accurate annotations enabled Oishii’s robot to detect ripeness levels and cutting points with high precision.
- The robot successfully avoided damaging unripe berries or plants, improving harvest quality.
- Scalability for Future Use Cases:
- Labellerr’s platform prepared Oishii to handle larger datasets for other crops or advanced robotic applications.
- The scalable annotation solution ensured that Oishii could expand their AI initiatives without delays.
Case Study: Revolutionizing Waste Segmentation
Spare-it is a company dedicated to sustainability, focusing on waste management solutions to help organizations reduce their environmental impact.
They developed an AI-powered waste segmentation model to automate the identification and categorization of waste types. This model aims to improve waste sorting processes and reduce landfill waste by enhancing recycling efficiency.
To train their AI model, Spare-it needed high-quality labeled data. This involved annotating images of waste with multiple categories, such as plastics, metals, paper, and organic materials. The complexity of these tasks required precise and efficient data labeling.
Challenges Faced by Spare-it
- Complex Data Requirements:
Spare-it’s project involved classifying waste into detailed categories with subtle visual differences. For example, distinguishing between recyclable and non-recyclable plastics required highly accurate annotations. - Volume of Images:
The project required labeling thousands of waste images from diverse environments, including office spaces, public bins, and industrial sites. Managing this large volume manually would have been time-consuming and error-prone. - Accuracy for AI Performance:
High-quality annotations were critical to ensure the AI model could accurately identify and categorize waste, even in cluttered or poorly lit images. Errors in labeling could lead to incorrect sorting and reduced model performance.
How Labellerr Helped?
Labellerr helped Spare-it by providing a tailored solution to meet specific requirements, ensuring efficiency and accuracy at every stage of the labeling process:
- Advanced Annotation Tools:
- Labellerr used a combination of bounding boxes, semantic segmentation, and polygons to accurately annotate waste items in images.
- The tools supported detailed categorization, allowing annotators to label even subtle differences between waste types.
- AI-Assisted Labeling:
- Labellerr’s AI-assisted features automated repetitive tasks like drawing bounding boxes and suggested pre-labels for review.
- These features significantly reduced manual effort and sped up the annotation process.
- Quality Assurance Mechanisms:
- Labellerr implemented quality control techniques like confidence scoring and anomaly detection to ensure consistent and accurate annotations.
- Multiple reviewers validated the annotations to eliminate errors and improve dataset quality.
- Integration with Spare-it’s Workflow:
- Labellerr seamlessly integrated with Spare-it’s AI pipeline, allowing easy uploading, processing, and exporting of annotated data in formats compatible with their machine learning model.
Results and Impact
- Improved Annotation Efficiency:
- Labellerr reduced the annotation time by 65%, enabling Spare-it to complete their project ahead of schedule.
- Automation tools helped Spare-it’s team focus on refining their AI model instead of spending excessive time on data labeling.
- Enhanced Model Performance:
- The high-quality labeled data provided by Labellerr allowed Spare-it’s AI model to achieve high accuracy in waste categorization.
- The model performed reliably in real-world scenarios, improving waste sorting processes and reducing errors.
- Scalability for Future Projects:
- Labellerr’s platform enabled Spare-it to handle additional datasets with ease, preparing them for expansion into new waste management applications.
- The scalable solution supported Spare-it’s mission to drive sustainability through technology.
Case Study: Efficiency With AI In Sustainable Aquaculture
Aquaculture, the farming of aquatic organisms, plays a vital role in food production worldwide. However, it faces challenges like waste management, water quality monitoring, and overfeeding, which impact sustainability and profitability.
A forward-thinking startup aimed to address these issues by developing an AI-powered system to monitor fish health, detect anomalies, and optimize feeding strategies in aquaculture farms.
To build their AI model, the startup needed high-quality annotated datasets, particularly for fish behavior and water quality analysis. The data included underwater videos and images, which required detailed labeling to train the AI for object detection and pattern recognition.
Challenges Faced by the Startup
- Complex Data Requirements:
- Annotating underwater images of fish behavior involved challenges like motion blur, varying light conditions, and overlapping fish.
- Labeling specific behaviors, such as feeding, stress signs, and group movement patterns, required high precision.
- Volume of Data:
- The project involved annotating thousands of video frames and images from multiple aquaculture farms.
- Managing this large dataset manually would have been time-intensive and error-prone.
- Accuracy for AI Model Training:
- The AI model relied on accurate and consistent annotations to detect subtle changes in fish behavior and water quality.
- Poor labeling could lead to incorrect predictions, reducing the system’s effectiveness in improving aquaculture sustainability.
How Labellerr Helped?
Labellerr helped aquaculture by providing a comprehensive solution tailored to the unique needs of this project:
- Custom Annotation Features:
- Labellerr’s platform supported detailed labeling with tools like bounding boxes, polygons, and semantic segmentation to annotate fish, their movements, and environmental factors in underwater images.
- These features allowed annotators to differentiate between healthy and stressed fish, detect group behaviors, and monitor water clarity.
- AI-Assisted Annotation Tools:
- Labellerr’s AI-powered pre-labeling features automated repetitive tasks, such as identifying fish in frames, significantly speeding up the annotation process.
- Confidence scoring highlighted annotations that needed review, ensuring consistent quality across the dataset.
- Efficient Workflow and Quality Assurance:
- Labellerr implemented a two-tier review process to validate annotations, reducing errors and maintaining high accuracy.
- The platform’s integration capabilities allowed seamless export of labeled data in formats compatible with the startup’s machine learning pipeline.
Results and Impact
- Streamlined Annotation Process:
- Labellerr reduced the annotation time by 60%, enabling the startup to complete their dataset preparation ahead of schedule.
- This efficiency allowed the team to focus more on refining their AI model and deployment.
- Improved AI Model Accuracy:
- The high-quality labeled data provided by Labellerr significantly enhanced the model’s ability to detect subtle fish behaviors and environmental changes.
- The AI system helped aquaculture farmers optimize feeding, monitor fish health, and reduce waste, improving both sustainability and profitability.
- Scalable Solution for Future Growth:
- Labellerr’s scalable platform prepared the startup to handle larger datasets as they expanded their operations to new farms and regions.
- The flexibility of the annotation tools allowed them to adapt to new use cases, such as monitoring different fish species or environmental parameters.
The case studies of Labellerr’s partnerships with Oishii, Spare-it, and the sustainable aquaculture startup highlight how tailored data labeling solutions drive innovation across diverse industries.
These examples demonstrate the critical role that high-quality annotations play in building reliable AI models and achieving transformative results.
Across these partnerships, Labellerr proved to be a trusted partner by providing efficient workflows, scalable solutions, and exceptional accuracy.
By reducing annotation time, improving model performance, and enabling seamless integration, Labellerr empowered these companies to achieve their goals and make a meaningful impact in their industries.
Whether it's revolutionizing farming, waste management, or aquaculture, Labellerr’s innovative tools and expertise showcase how advanced data labeling can drive success in AI projects.
These case studies reinforce the importance of choosing a reliable data labeling company to overcome challenges and unlock the full potential of AI solutions.
How Labellerr Stands Out as a Data Labeling Company
Labellerr is not just another data labeling company; it is a comprehensive solution designed to meet the unique challenges of modern AI projects.
From advanced technology to exceptional customer support, Labellerr offers everything you need to ensure your data labeling process is efficient, accurate, and scalable. Here's what makes Labellerr stand out:
1. Advanced Features
Labellerr’s platform is built with cutting-edge technology to simplify and enhance the annotation process.
- AI-Assisted Annotation:
Labellerr uses AI to automate repetitive tasks like drawing bounding boxes, segmenting objects, or labeling points. Pre-labeling features allow annotators to review and refine instead of starting from scratch, saving time and effort. - Quality Assurance:
Ensuring accurate annotations is a priority. Labellerr employs techniques like:- Confidence Scoring: Highlights uncertain annotations for review.
- Anomaly Detection: Flags inconsistencies in labeling.
- Inter-Annotator Agreement: Ensures consistency across teams.
- Seamless Integration:
Labellerr integrates with popular machine learning pipelines and cloud platforms like AWS, Google Cloud, and Azure. It supports multiple data formats, such as COCO, YOLO, and Pascal VOC, making it easy to export labeled datasets for immediate use.
These advanced features help streamline the entire workflow, enabling faster project timelines and higher-quality results.
2. Scalability
Labellerr is designed to handle projects of any size, making it a perfect partner for startups and enterprises alike.
- Managing Large Datasets:
Labellerr’s platform efficiently processes thousands or even millions of data points without compromising quality. Whether it’s images, videos, text, or audio, the platform scales effortlessly to meet your needs. - Industry Adaptability:
Labellerr has experience working across industries such as healthcare, retail, autonomous vehicles, agriculture, and waste management. Its versatile tools and tailored solutions ensure that domain-specific challenges are addressed effectively. - Future-Ready Solutions:
Labellerr prepares businesses to handle growing datasets and evolving AI requirements, ensuring they remain competitive as their projects expand.
3. Customer Support
Labellerr prioritizes customer satisfaction by providing dedicated support throughout the project.
- Dedicated Project Managers:
Each project is assigned a dedicated manager who ensures smooth communication, manages timelines, and provides regular updates. This one-on-one support keeps your project on track and aligned with your goals. - Real-Time Updates:
Labellerr’s platform provides real-time feedback and progress tracking. This transparency allows teams to monitor the annotation process and make adjustments as needed. - Collaboration Features:
The platform supports collaboration between annotators, reviewers, and project managers, ensuring that feedback loops are efficient and productive.
4. Success Stories
Labellerr’s proven track record speaks for itself. The platform has helped startups and enterprises across various industries achieve their goals efficiently:
- Oishii:
Labellerr reduced annotation time by 70% for a strawberry segmentation project, enabling precise harvesting by autonomous robots. The solution improved efficiency and reduced waste, showcasing Labellerr’s ability to handle complex agricultural use cases. - Spare-it:
For a waste segmentation project, Labellerr’s tools streamlined the annotation of waste images, reducing time and improving the AI model’s accuracy. This partnership empowered Spare-it to develop a sustainable solution for waste management. - Sustainable Aquaculture Startup:
Labellerr enabled precise annotation of underwater images for monitoring fish behavior and optimizing feeding strategies. The solution improved model performance and helped the startup promote sustainable aquaculture practices.
Labellerr stands out as a reliable partner for AI projects by combining advanced features, scalability, exceptional support, and a track record of success.
Whether you’re a startup looking to accelerate your AI development or an enterprise aiming to scale your operations, Labellerr offers the tools and expertise to meet your goals efficiently.
Labellerr isn’t just about labeling data; it’s about empowering your AI vision with high-quality annotations, faster timelines, and scalable solutions that pave the way for success.
Conclusion
Choosing the right data labeling company is a crucial step in ensuring the success of your AI projects. High-quality labeled data directly impacts the accuracy and performance of AI models, making it essential to partner with a company that meets your specific needs.
When evaluating a data labeling company, focus on key factors such as:
- Quality Assurance: Ensure the company has robust mechanisms like confidence scoring, anomaly detection, and inter-annotator agreement to maintain consistent and accurate annotations.
- Scalability and Flexibility: Choose a partner that can handle large datasets and adapt to the changing needs of your project.
- Industry Expertise: Look for a company experienced in your domain, whether it’s healthcare, autonomous vehicles, retail, or any other industry.
- Advanced Technology: The use of AI-assisted tools, seamless ML pipeline integration, and compatibility with common data formats ensure efficiency and ease of use.
- Security and Compliance: Ensure the company follows strict data security practices and complies with regulations like GDPR, HIPAA, or CCPA.
- Transparent Pricing: Select a partner with a clear pricing structure that aligns with your budget.
- Strong Communication: A reliable partner should offer dedicated project managers, real-time updates, and collaborative tools to keep your project on track.
Labellerr is a trusted partner that checks all these boxes. With advanced annotation features, scalable solutions, and a strong track record across multiple industries, Labellerr has consistently delivered exceptional results. Whether you’re a startup or an established enterprise, Labellerr’s tailored solutions and expertise can accelerate your AI project timelines, reduce costs, and ensure high-quality labeled data.
By partnering with Labellerr, you gain more than just a data labeling service—you gain a team committed to supporting your AI vision and helping you achieve success in a competitive market.
Ready to simplify and accelerate your AI development? Book-a-demo Labellerr today and transform your data annotation process into a seamless and efficient experience.
FAQs
Why is choosing the right data labeling company important?
The right company ensures accurate and consistent annotations, which directly improve the performance of your AI models. A reliable partner can save time, reduce costs, and help scale your projects efficiently.
What should I prioritize when selecting a data labeling partner?
Focus on quality assurance, scalability, domain expertise, advanced technology, security compliance, transparent pricing, and strong communication.
How can Labellerr help improve my data annotation workflows?
Labellerr offers AI-assisted annotation, quality assurance mechanisms, seamless integration with ML pipelines, and scalable solutions. These features streamline workflows, reduce annotation time, and enhance data accuracy for better AI model performance.
Book our demo with one of our product specialist
Book a Demo