5 Best Speech-to-Text Annotation Tools in 2024
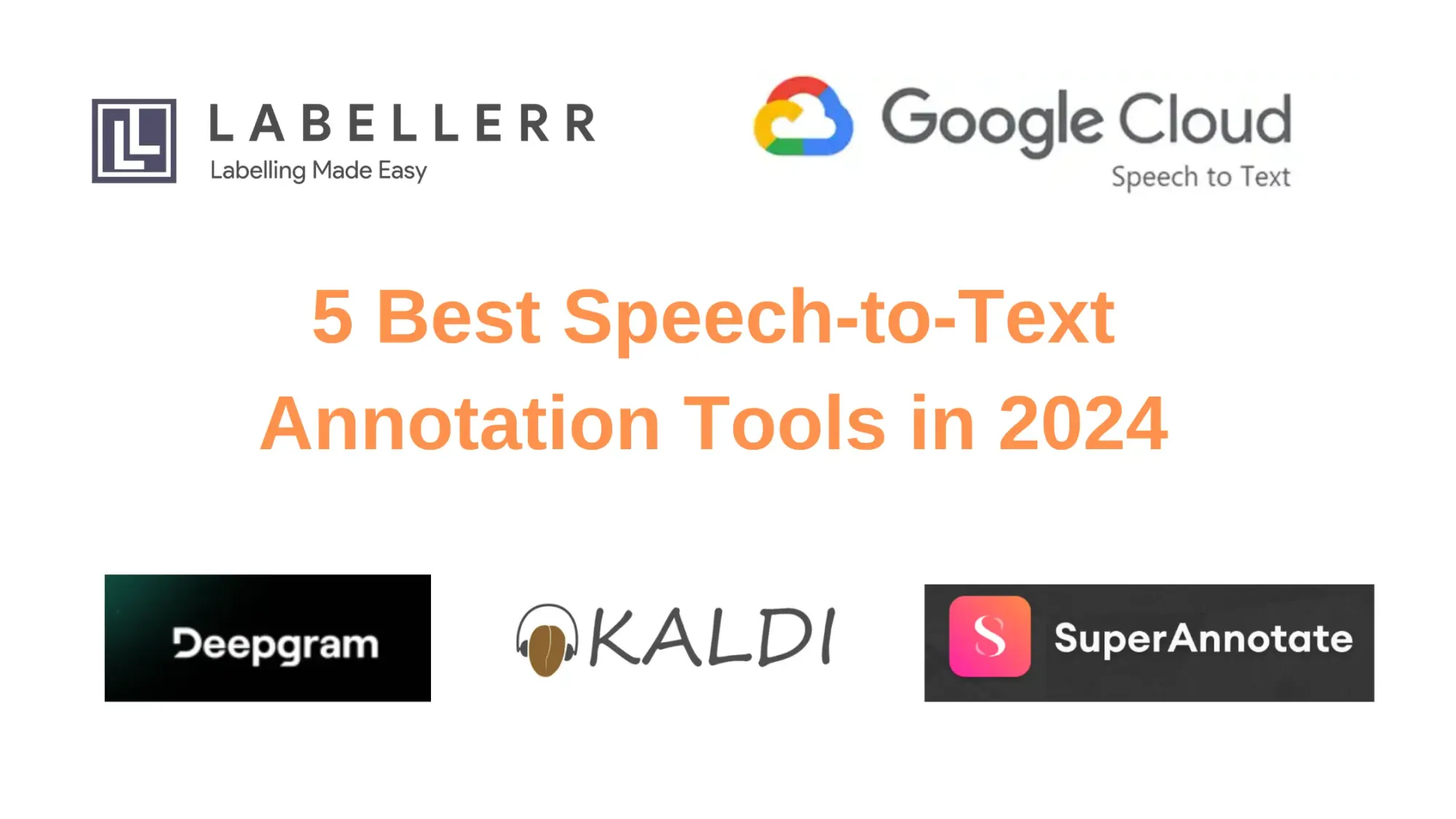
Speech-to-text annotation is important for understanding and analyzing audio data in fields like speech recognition, language processing, and sound analysis. This process involves labeling audio parts to help machines learn and find important information.
As the need for detailed audio analysis grows, so does the need for good speech-to-text annotation tools. In this article, we'll look at five advanced tools known for their features, ease of use, and ability to work with different audio formats.
Whether you're a linguist, researcher, podcaster, or AI fan, this list can help you find the right tool for your needs. Whether you want to make annotation easier, improve accuracy, or study sound closely, this article is your guide to the best speech-to-text annotation tools.
Table of Contents
Top 5 Speech-to-Text Annotation Tools
1. Labellerr
Labellerr is a specialized speech-to-text tool crafted for annotating and labeling audio data, particularly instrumental in training machine learning and AI models.
Here's what sets Labellerr apart:
1. User-Friendly Interface
Labellerr boasts an intuitive design, simplifying the audio data labeling process with drag-and-drop functionality for efficient annotation.
2. Format Versatility
Supporting a range of audio formats like MP3, WAV, and FLAC, Labellerr accommodates diverse audio types such as speech, music, and environmental sounds, crucial for various AI applications.
3. Annotation Features
Labellerr equips users with labeling tools to add metadata like timestamps, facilitating supervised machine learning by providing labeled data for model training.
4. Collaborative Functionality
With collaborative features, Labellerr enables multiple annotators or team members to work concurrently on projects, enhancing productivity and streamlining the annotation process.
5. Integration and Compatibility
Labellerr seamlessly integrates with other platforms and tools, ensuring smooth import/export of data and compatibility with different machine learning frameworks.
6. Version Control
Labellerr maintains a history of annotations, enabling versioning and tracking changes made to annotated audio segments over time.
7. Security Measures
Labellerr prioritizes data security, employing encryption, user access controls, and compliance with privacy regulations to safeguard sensitive audio data.
8. Scalability and Performance
Capable of efficiently handling large audio datasets, Labellerr maintains optimal performance even under heavy workloads.
Use Cases
1. Speech Recognition
Labellerr aids in labeling audio data to train speech recognition models, enhancing their accuracy in transcribing speech to text.
2. Sound Classification
It's instrumental in annotating audio samples for sound classification tasks, and identifying sounds like footsteps or car horns within datasets.
3. Sentiment Analysis
Labellerr facilitates the annotation of audio data for sentiment analysis, discerning emotions or tones in spoken language for applications like customer service or market research.
4. Training AI Models
Labellerr is a vital tool in training and refining AI models utilizing audio data, spanning applications in natural language processing, audio generation, and beyond.
Read one of their case study here.
2. SuperAnnotate
SuperAnnotate offers an advanced speech-to-text annotation platform, designed to deliver precise and high-quality annotations for various audio file formats.
Let's delve into its standout features:
1. Audio File Compatibility
Supporting a wide array of audio file types, SuperAnnotate ensures seamless usability across diverse audio sources.
2. Diverse Use Cases
Its capabilities extend across various applications, from speech recognition and speaker identification to sound event detection and audio classification.
3. Industry Reach
SuperAnnotate serves an extensive range of industries, including agriculture, healthcare, insurance, sports, robotics, autonomous driving, aerial imagery, NLP, security, and surveillance.
4. Quality Assurance
With built-in quality assurance features like collaboration systems, item and project status tracking, and detailed instructions, SuperAnnotate emphasizes the importance of accuracy and precision in annotations.
5. Innovative Solutions
SuperAnnotate offers tailored solutions for specific industries and use cases, including annotation software, annotation services via a global marketplace, and project and quality management tools.
6. Comprehensive Support
The platform covers a diverse range of data types, allowing users to generate high-quality training data across different formats.
It also provides access to a global marketplace of annotation service teams and tools for effective project management and annotator oversight.
Industry Applications
SuperAnnotate's speech-to-text annotation tool finds applications across numerous industries, enabling tasks in agriculture, healthcare, insurance, sports, robotics, autonomous driving, aerial imagery, NLP, security, and surveillance.
Its versatility and adaptability make it indispensable for a wide array of audio annotation needs within these sectors.
3. Google Cloud Speech-to-Text
Google Cloud Speech-to-Text is a speech-to-text annotation tool, primarily focused on transcribing spoken words into written text.
Its standout features include:
1. Application Programming Interface (API): Seamlessly integrates with various applications, simplifying the transcription of audio data.
2. Application Integration: Enables easy integration with existing applications, streamlining the transcription process for users.
3. Text Summarization: Offers text summarization capabilities, condensing lengthy documents into concise summaries, thus improving efficiency.
Pricing:
Free Trial: Provides a 60-minute free trial for transcription and analysis, coupled with $300 in free credits for new customers.
Paid Plans: Subscription plans are based on monthly usage.
User Feedback
Positive:
Users commend Google Cloud Speech-to-Text for its remarkable accuracy and versatility.
They appreciate its scalability and reliability, noting its ability to handle varying workloads without compromising quality, catering to businesses of all sizes.
Negative:
Some users have reported difficulties with Indian accents, though no significant issues have been widely reported.
4. Kaldi
Kaldi is an open-source speech-to-text annotation tool, licensed under the Apache License and freely available to users.
Originating from a workshop at Johns Hopkins University in 2009, its primary aim was to achieve high-quality speech recognition for new languages and domains at minimal development costs.
Released on May 14, 2011, Kaldi quickly gained recognition for its user-friendly interface.
Written primarily in C++, it's predominantly used for research in acoustic modeling.
Key Features:
1. Comprehensive Support
Kaldi supports full covariance structures and Gaussian mixture modules, including advanced techniques like MMI (Maximum Mutual Information) and boosted MMI.
2. Integration with FSTs
It seamlessly integrates with Finite State Transducers (FSTs) through the OpenFst toolkit, enhancing its functionality.
3. Language Model Conversion
Kaldi provides tools for converting Language Models (LMs) from the standard ARPA format to FSTs, ensuring compatibility.
4. Matrix Library
It utilizes a matrix library wrapping standard Basic Linear Algebra Subroutines (BLAS) and Linear Algebra Package (LAPACK) routines, supporting general linear algebra.
5. Extensible Design
Kaldi features an extensible design with space for discriminative training, allowing for further customization and optimization.
6. Deep Neural Networks
It offers comprehensive recipes and deep neural networks for improved performance and accuracy.
7. Adaptation Techniques
Kaldi implements techniques like Maximum Likelihood Linear Regression (MLLR) for model-space adaptation and feature-space MLLR for feature-space adaptation, enhancing adaptability.
Kaldi's versatility, robust feature set, and support for various adaptation techniques make it a valuable tool in speech-to-text annotation.
5. Deepgram
Deepgram is a robust speech-to-text annotation tool, renowned for its precise transcription of spoken language into written text, even across varying accents.
Its capabilities include the swift processing of large volumes of audio data, making it particularly well-suited for businesses needing transcription services for calls, meetings, or customer interactions.
The company is dedicated to advancing human language understanding.
Key Features
1. Transcription Speed
Deepgram can transcribe live or pre-recorded audio almost in real time, ensuring rapid results.
2. Multi-lingual Support
With comprehension and transcription abilities in over 30 languages, Deepgram caters to diverse linguistic requirements.
3. Accuracy
It delivers transcriptions with over 90% accuracy, ensuring reliability in text conversion.
Pricing
Free Plan: Offers a Pay As You Go option with a $200 credit.
Growth Plan: Priced between $4,000 and $10,000 annually.
Enterprise Plan: Pricing is available upon request. Interested users can contact the Deepgram team for detailed information.
User Feedback
Positive
Users praise Deepgram for its essential role in projects requiring accurate real-time transcription, such as providing translations during events.
Its ease of integration, configuration, and multilingual support are highlighted as significant advantages contributing to project success.
Negative
Some users express a desire for Deepgram to include real-time transcription capabilities for video calls using their API.
Nevertheless, Deepgram is widely regarded as a reliable choice for speech-to-text annotation needs.
Read our other listicles:
1. 6 Best Automatic Speech Recognition (ASR) Tools in 2024
2.7 Best Text Annotation & Labeling Tools In 2024
Conclusion
In this blog, we've explored five advanced speech-to-text annotation tools tailored for diverse needs, from Labellerr's user-friendly interface to Deepgram's swift transcription capabilities.
These tools empower users to streamline annotation processes, enhance accuracy, and extract insights from audio data efficiently.
Whether you're a researcher, podcaster, or AI enthusiast, these tools offer invaluable resources for improving speech recognition, sound classification, sentiment analysis, and training AI models.
With their versatility and robust features, they stand ready to unlock the potential of audio data in various fields, advancing human language understanding in today's digital landscape.
Frequently Asked Questions
1. How to choose text-to-speech annotation tools in NLP projects?
Choosing TTS annotation tools for NLP projects involves considering factors such as the specific annotation tasks required, the complexity of the data, the ease of integration with existing workflows, the availability of pre-trained models, the tool's scalability, and the level of support and documentation provided.
Additionally, evaluating the tool's accuracy, versatility, and cost-effectiveness is essential to ensure it aligns with project requirements and goals.
Conducting thorough research, testing multiple options, and seeking feedback from team members can help in making an informed decision.
Book our demo with one of our product specialist
Book a Demo