6 Best Data Curation Tools for Computer Vision in 2025
In 2025, top data curation tools for computer vision include Labellerr, Lightly AI, Scale Nucleus, Labelbox, Encord, and ClarifAI. These platforms offer features like automation, model-assisted labeling, and seamless integration.
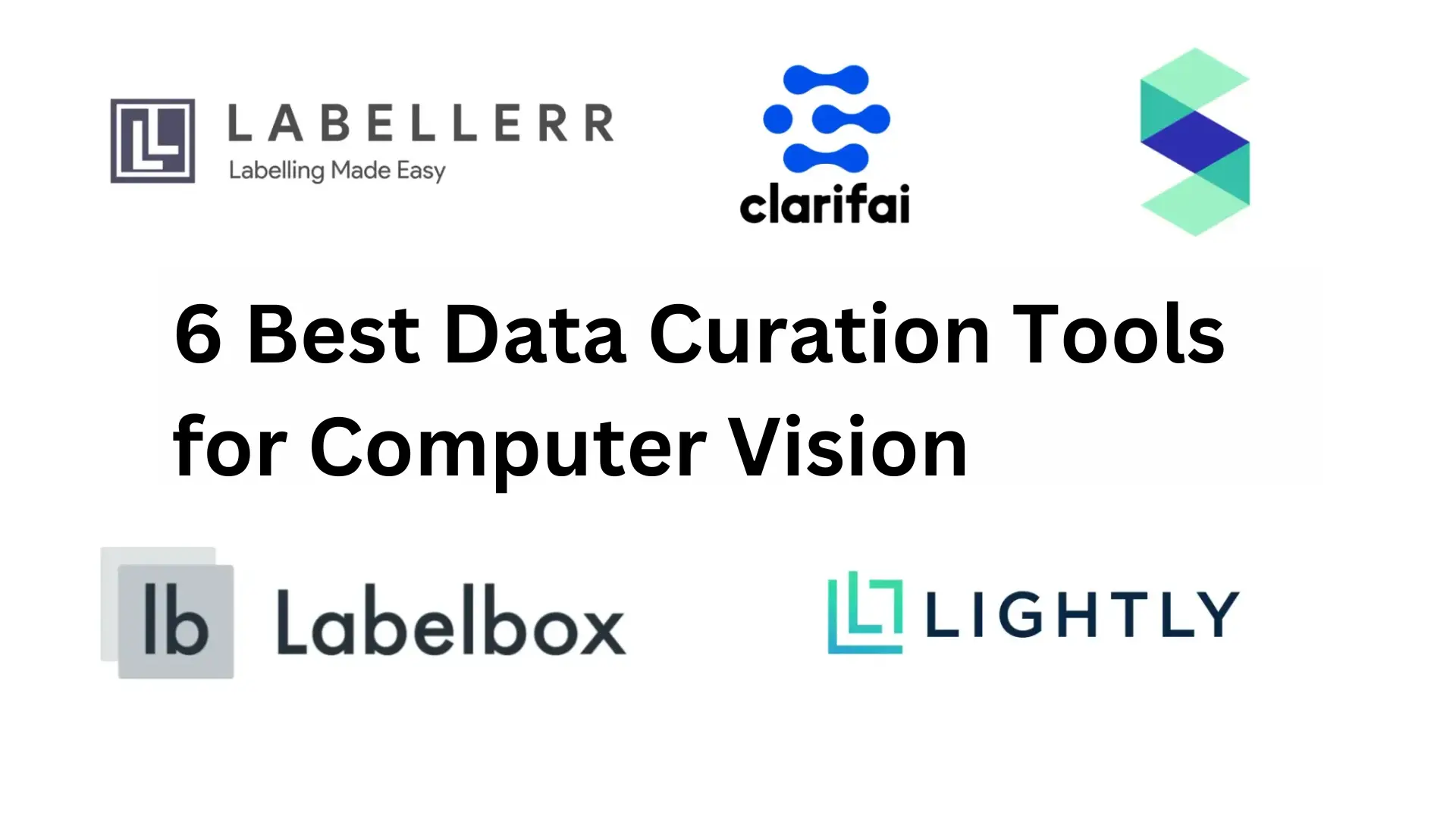
Data curation tools has the power to save AI-ML teams countless hours and make them efficient and more productive. In this article, we'll explore some of the top curation tool for year 2025.
This is what we'll cover-
Table of Contents
- What is Data Curation in Computer Vision?
- Why is Data Curation Important?
- 7 Points to Consider for Selecting a Data Curation Tool in Computer Vision
- Best Data Curation Tools
- Labellerr
- Lightly AI
- Scale Nucleus
- abelbox
- Encord
- ClarifAI
- Conclusion
- Frequently Asked Questions
What is Data Curation in Computer Vision?
Data curation in computer vision is all about taking good care of the pictures and videos that help machines learn.
You collect, clean, organize, check, and keep the data tidy so that it works well for teaching computers to see things.
Before, people just gave their labeling team lots of raw images to work with. But now, that's not enough.
With data curation, you make sure the data is really good quality and fits what the computer needs to learn.
This is important because if you don't do this right, the computer might make mistakes or not see things properly.
Think of it like baking a cake. You need the best ingredients, right?
Data curation helps you pick the best "ingredients" (or data) for teaching your computer vision models.
And just like you need a good recipe for a cake, you need the right tools for data curation.
These tools help you pick the right data, fix mistakes, and make sure your team can work together smoothly.
So, having good data curation tools is like having a special ingredient for baking the perfect cake - or in this case, training the perfect computer vision model!
Why is Data Curation Important?
Data curation has become incredibly important due to a shift in focus from adjusting with models to improving the quality of data.
In the past, engineers mainly focused on adjusting the model itself to enhance performance.
However, with the rise of a data-centric approach, attention has turned to systematically improving the quality of the data used to train these models.
This shift underscores the critical role of data curation in ensuring the success of machine learning projects.
The tools used for data curation directly impact the quality and performance of the models they train.
Therefore, it's crucial to choose the right data curation tools tailored to the specific needs of the project.
Effective data curation empowers machine learning teams in several key ways:
1. Selecting the right data
By enabling teams to pick the most relevant and valuable data samples, data curation ensures that the model learns from high-quality inputs.
Additionally, tools that allow querying based on metadata and manipulating distributions help create an ideal training dataset.
2. Integration into data pipelines
Quality data curation tools seamlessly fit into existing machine learning pipelines and storage systems, facilitating efficient workflow management.
3. Performing data-based model debugging
Data curation tools assist in identifying and addressing errors in the data, such as false positives and negatives, which can significantly impact model performance.
4. Visualizing data
Visualizing the dataset helps in identifying biases, outliers, and edge cases, which are crucial for understanding the limitations and potential areas for improvement in the model.
5. Managing datasets
Collaboration features ensure that team members can work together effectively, streamlining the data curation process and promoting better outcomes.
Data curation ensures that the data used to train machine learning models is of high quality, relevant, and well-suited to the task at hand.
This, in turn, enhances the performance, accuracy, and reliability of the models, ultimately leading to better outcomes in computer vision projects.
7 points to consider for Selecting a Data Curation Tool in Computer Vision
Considerations for selecting a data curation tool in computer vision include:
1. Data Prioritization
Ensure the tool can filter, sort, and select data effectively, handling large datasets and automating prioritization tasks if possible.
2. Visualizations
Look for customizable visualization options for better understanding and analysis of data, supporting various formats like tables, plots, and images.
3. Model-Assisted Insights
Seek features that enable debugging by visualizing model performance, using tools like confusion matrices or saliency maps to identify issues.
4. Modality Support
Ensure the tool can handle different data types such as images, videos, and medical imaging formats, with support for various annotation types.
5. Simple and Configurable UI
Opt for a tool with an intuitive interface accessible to both technical and non-technical users, supporting automated workflows and programmable integration.
6. Annotation Integration
Look for seamless annotation workflows, allowing for easy creation, editing, and management of labels and annotations.
7. Collaboration
Choose a tool that supports collaboration among multiple users, facilitating shared projects and real-time collaboration on datasets and annotations.
Best Data Curation Tools
1. Labellerr
Labellerr is an exceptional data curation tool that stands out for its remarkable efficiency and comprehensive features.
It offers a seamless experience for AI teams by providing high-quality labels at an incredible speed, making the process 99 times faster than traditional methods.
Labellerr's versatility extends to various data types, including images, videos, PDFs, text, and audio, ensuring that it meets the diverse needs of different projects without the hassle of switching between multiple tools.
One of Labellerr's key strengths lies in its advanced automation capabilities, which include prompt-based labeling, model-assisted labeling, and active learning-based labeling automation.
These features not only expedite the labeling process but also ensure accuracy and precision in the annotations.
Moreover, Labellerr's smart quality assurance mechanisms, powered by pre-trained models and ground truth validation, further enhance the reliability of the labeled data, giving ML teams full confidence in their datasets.
Another standout feature of Labellerr is its seamless integration with MLOps, enabling effortless collaboration and integration with various AI development environments such as GCP Vertex AI, AWS Sagemaker, and custom setups.
This ensures that ML workflows are streamlined and efficient, from data preparation to model deployment.
Labellerr's dedication to customer satisfaction is evident through its round-the-clock support, ensuring that teams receive timely assistance and guidance whenever needed.
Additionally, the platform's advanced analytics and project management capabilities empower users with valuable insights into their data, facilitating informed decision-making and optimizing project workflows.
Here are the pros and benefits of Labellerr:
1. High-quality labels at Lightning Speed
Labellerr delivers top-notch labels with exceptional accuracy, ensuring reliable data for training AI models.
The platform accelerates the labeling process by up to 99 times faster than traditional methods, saving significant time and resources.
2. Versatile Data Support
Supports various data types including images, videos, PDFs, text, and audio, catering to the diverse needs of different projects.
3. Advanced Automation
Utilizes prompt-based labeling, model-assisted labeling, and active learning-based labeling automation for swift and precise annotations.
Automation features streamline the labeling workflow, reducing manual effort and minimizing human error.
4. Smart Quality Assurance
Employs pre-trained models and ground truth validation for smart quality assurance, ensuring the accuracy and reliability of labeled data.
Automated QA processes enhance data integrity and minimize the need for manual verification.
5. Seamless MLOps Integration
Integrates seamlessly with MLOps, facilitating collaboration and integration with popular AI development environments such as GCP Vertex AI and AWS Sagemaker.
Enables smooth workflow from data preparation to model deployment, enhancing efficiency and productivity.
6. Advanced Analytics and Project Management
Provides comprehensive project management tools and advanced analytics for insightful data visualization and decision-making.
Empowers users with valuable insights into their data, optimizing project workflows and maximizing efficiency.
7. 24/7 Customer Support
Offers round-the-clock technical support, ensuring prompt assistance and guidance for crucial projects.
Ensures customer satisfaction and seamless user experience with fast response times and expert assistance.
Overall, Labellerr emerges as a top-tier data curation tool that not only accelerates the labeling process but also enhances the overall efficiency, accuracy, and cost-effectiveness of ML projects.
With its powerful features, intuitive interface, and unwavering commitment to quality, Labellerr sets a new standard for data annotation platforms.
Labellerr's strengths lie in its ability to deliver high-quality labeled data swiftly and efficiently, supported by advanced automation, smart QA, seamless integration, and robust customer support.
2. Lightly.AI
Lightly.ai is a tool for organizing lots of pictures so computers can understand them better.
It helps with computer vision, which means teaching computers to see and recognize things in images.
Here's how it works:
Imagine you have a huge collection of pictures, maybe millions of them.
Lightly.ai helps sort through all these pictures to find ones that are similar to each other.
This makes it easier to train computer programs to understand and recognize different things in pictures.
Lightly.ai can learn on its own. It doesn't always need someone to tell it what everything is in the pictures.
And guess what? You can even use Lightly.ai on your computer systems if you want.
This means you can keep all your pictures and data safe and private, which is important, especially for big companies or sensitive projects.
Pros:
1. It helps pick out the most important pictures to train computers.
2. It can handle lots of pictures, even millions!
3. You can use it on your computers, so your data stays safe.
4. It doesn't always need labels on pictures to work well.
Cons:
1. It's more about organizing the data than fixing problems with the computer models themselves.
Overall, Lightly.ai is a handy tool for anyone working with lots of pictures, especially if they want to keep their data secure and organized.
3. Scale Nucleus
Scale Nucleus is like a hub for teams working on teaching computers to understand things in images.
It came out in 2020 and is made by a company called Scale.
This tool lets teams work together easily, sending pictures to be labeled either by Scale's workers or using the built-in editor.
Right now, it can only handle visual data.
But it's got some good features like searching for specific details in the data, finding and fixing mistakes in labels, and connecting with other software through its API (although it can't be used on your own computer systems just yet).
Pros:
1. It connects directly with Scale's labeling team, so you can get your data labeled quickly.
2. You can see predictions and labels to spot where your computer model might be making mistakes.
3. It helps you find similar pictures in your data easily.
Cons:
1. It's not great for handling really big sets of unlabeled data.
2. Once you start using it, it's kinda hard to switch to a different tool.
3. Picking out data has to be done manually through the user interface.
Scale Nucleus is part of a whole set of tools from Scale AI that help with making AI models better.
It's especially good for teams who want a simple way to organize their picture data and get help with labeling.
4. Labelbox
Labelbox is useful for teams working on teaching computers to understand things in images.
It focuses on a part of the process called the "training data iteration loop".
It helps with three main things: putting labels on pictures, checking how well your computer model is doing, and deciding which pictures are most important based on that.
One thing about Labelbox is that it's really good for teams working together, especially if they're not all in the same place.
It makes it easy for everyone to work on projects together, even if they're far away from each other.
Pros:
1. It connects directly with Labelbox's labeling tool, so you can label your data right there.
2. You can use something called "embeddings" to group similar pictures together, which is super handy.
Cons:
1. You have to pick out the data you want to work with manually using the interface.
Overall, Labelbox is great for teams who want to work together on labeling lots of pictures and making sure their computer models are doing a good job.
5. Encord Active
Encord Active is a data curation tool for ML engineers who work with computer vision models.
It's all about making models better by finding out where they might be going wrong and picking the best data to label next.
Plus, it helps save time and money on labeling while giving a deeper understanding of how your models work.
Pros:
1. It's open source, meaning anyone can use it for free, and it works on any computer system.
2. It's packed with tools to measure how good your data is and figure out what needs fixing.
3. You can even make your own tools to fit your specific needs, like focusing on certain types of images or data.
Some highlights of what it can do:
1. It comes with lots of metrics to help understand your data better.
2. You can customize metrics to fit your specific needs.
3. It has its own labeling tool built right in.
4. It's smart enough to find similar images for you based on machine-learning tricks.
5. It works great for healthcare, especially with medical images.
Who's it best for?
Anyone who wants to make their data better for training computer vision models.
Whether you're a big company with lots of experience or just starting out, Encord Active could be a handy addition to your toolbox.
Plus, since it's free and open source, it's a great option for anyone looking to save some cash.
6. ClarifAI
Clarifai is a data curation tool for teams who want to understand things in images, videos, and even text.
It offers lots of features like making custom models, adding tags automatically, searching for specific things in images, and drawing on pictures.
Pros:
1. It's easy to use for teams who are just starting out with machine learning.
2. You can work with all kinds of data, not just images.
3. It has many tools for different jobs like a big toolbox.
4. It's terrific if you're into robotics or making cars that can drive themselves.
Cons:
1. It's not meant for developers who want to build their tools. It's more like a finished product that you can use as it is.
Overall, if you're new to machine learning and want some help getting started, or if you're working on robots or self-driving cars, Clarifai could be a good fit for you. Just keep in mind that it's not open source.
Conclusion
Data curation plays a vital role in the field of computer vision by ensuring that the images and videos used to teach machines are of high quality and relevance.
By collecting, cleaning, organizing, and checking data, teams can provide the best "ingredients" for training computer vision models.
This approach has become increasingly important as attention shifts from adjusting models to improving data quality.
With the right data curation tools, teams can select the most relevant data, integrate it into their pipelines, debug models based on data insights, visualize datasets, and manage collaboration effectively.
Ultimately, effective data curation leads to better outcomes in computer vision projects, enhancing model performance, accuracy, and reliability.
Read our other listicles:
1. 5 Best Speech-to-Text Annotation Tools in 2025
2. 7 Best Audio Annotation Labeling Tools In 2025
Frequently Asked Questions
1. What makes a good data curation tool?
An effective data curation tool must have the capability to manage various data formats, including images, videos, DICOM, and geo.tiff, while also supporting a wide range of annotation formats such as bounding boxes, segmentation, polylines, and key points.
Additionally, it should cater to both technical and non-technical users who are involved in the curation process.
2. Why is data curation important?
Data curation is crucial because it ensures that the data used to train machine learning models is of high quality, relevant, and well-suited to the task at hand.
By collecting, cleaning, organizing, and checking data, data curation enhances the performance, accuracy, and reliability of machine learning models, ultimately leading to better outcomes in various fields such as computer vision, natural language processing, and data analysis.

Simplify Your Data Annotation Workflow With Proven Strategies
.png)
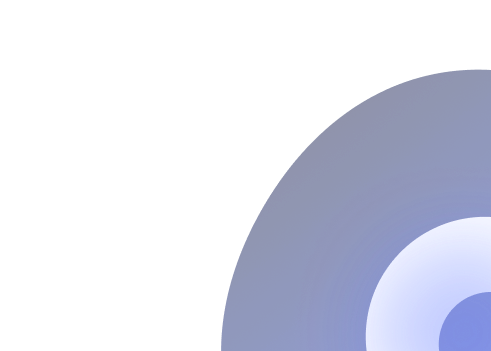