5 Best Chatbot Fine Tuning Tools in 2025
In 2025, top chatbot fine-tuning tools include Labellerr, Labelbox, Kili, Databricks Lakehouse, and Label Studio. These platforms offer personalized workflows, collaboration, and seamless integration with machine learning models to optimize chatbot performance.
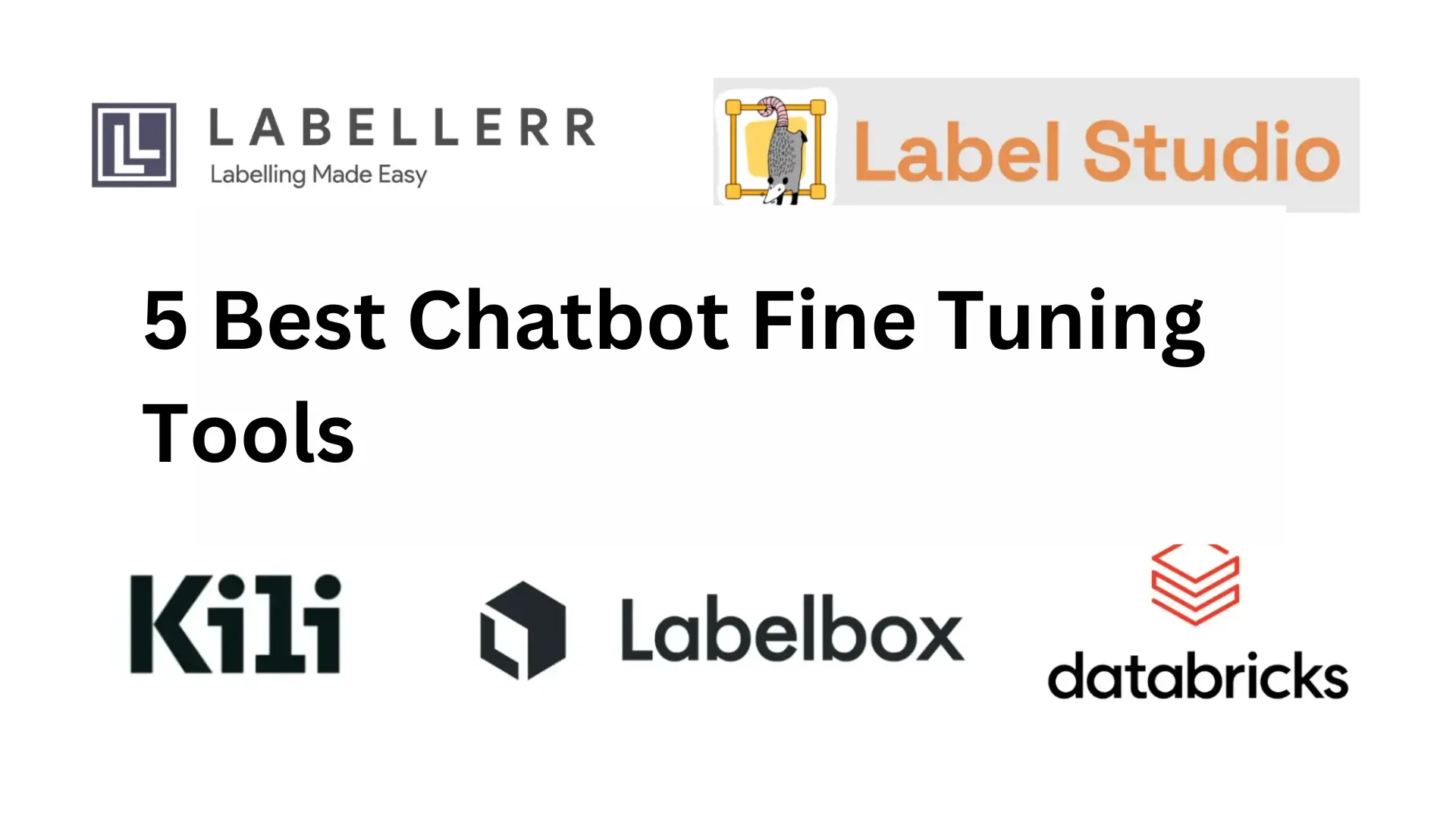
Refining Language Model Models (LLMs) for chatbots is a pivotal step in maximizing their effectiveness across diverse natural language processing tasks.
In this comprehensive exploration, we'll delve into five prominent tools – Labellerr, Kili, Label Studio, Databricks Lakehouse, and Labelbox – meticulously crafted to cater to the nuanced requirements of fine-tuning chatbots.
Whether you're a passionate machine learning enthusiast or a seasoned professional seeking to elevate your chatbot's performance, this in-depth guide offers a thorough analysis of the unique features, advantages, and tailored approaches of these cutting-edge tools.
Join us on this enlightening journey to unlock the full potential of chatbot optimization with these state-of-the-art fine-tuning solutions.
Table of Contents
- Top Chatbot Fine-Tuning Tools
- Labellerr
- Labelbox
- Databricks Lakehouse
- Kili
- Label Studio
- Conclusion
- Frequently Asked Questions
Top Chatbot Fine Tuning Tools
1. Labellerr
Labellerr as a chabot fine-tuning tool emerges as an innovative platform meticulously crafted to aid machine learning teams in seamlessly configuring the fine-tuning process for their chatbot models.
With its adaptable and personalized workflow, ML teams can significantly accelerate the preparation of top-tier training data for fine-tuning chatbot models in just a few hours.
This tool boasts many features designed to streamline the fine-tuning journey, making it an intuitive, efficient, and swift solution.
Key Features
1. Personalized Workflow Setup
Labellerr facilitates the creation of tailored annotation tasks, ensuring alignment with the unique requirements of chatbot fine-tuning, such as intent classification, entity recognition, and dialogue coherence assessment.
2. Versatility Across Data Formats
The ChatBot Fine-Tuning Tool supports various data formats, including text, images, audio, and video.
This versatility proves invaluable for chatbots engaged in multi-modal interactions that involve diverse data types.
3. Collaborative Annotation for Enhanced Productivity
Labellerr's collaborative annotation capabilities, particularly in its Enterprise version, enable multiple annotators to collaborate on the same dataset.
This collaborative feature simplifies the annotation process, distributes the workload effectively, and ensures uniformity in annotations.
4. Quality Control Measures
The ChatBot Fine-Tuning Tool incorporates quality control features such as annotation history tracking and disagreement analysis to ensure good annotation accuracy.
These tools play a pivotal role in upholding the precision and quality of annotations, which are paramount for the success of chatbot fine-tuning endeavors.
5. Seamless Integration with ML Models
Labellerr seamlessly integrates with machine learning models, enabling active learning workflows.
This entails harnessing chatbot models for pre-annotation, followed by human refinement, thereby enhancing the efficiency of the annotation process and augmenting fine-tuning outcomes.
The ChatBot Fine-Tuning Tool by Labellerr positions itself as an all-encompassing platform empowering machine learning teams to efficiently prepare top-quality datasets for fine-tuning chatbot models.
With its user-centric design and emphasis on customization and collaboration, Labellerr offers a valuable solution for unlocking the full potential of chatbots in the field of natural language processing and conversational AI.
2. Labelbox
Labelbox's ChatBot Fine-Tuning Tool offers a comprehensive solution tailored to streamline the fine-tuning process of ChatBot models.
These models, powered by deep learning techniques, play a crucial role in various natural language processing (NLP) applications such as AI-driven voice assistants, chatbots, translation services, and sentiment analysis.
Key Features and Workflow
1. Tailored Ontology Configuration
Define a customized classification ontology aligned with your specific ChatBot use case, ensuring the model is finely tuned to comprehend and generate content relevant to your domain.
2. Project Setup and Annotation
Create projects within Labelbox, aligning with the defined ontology for classifying data intended for ChatBot fine-tuning.
Utilize Labelbox's annotation capabilities to efficiently and accurately label training data.
3. Iterative Model Refinement
Use an iterative model to swiftly fine-tune ChatBot models.
Labelbox facilitates performance diagnosis, high-impact data identification, data labeling, and subsequent model iteration for refined fine-tuning.
4. Seamless Google Colab Integration
Streamline the process with Google Colab Notebook integration, allowing for the direct importation of necessary packages, including OpenAI and Labelbox, within the notebook.
API keys ensure a seamless connection to instances.
5. Adaptive Training Data Generation
Guide users in generating training data based on the defined ontology, which is crucial for adapting ChatBot models to specific use cases and capturing relevant nuances.
6. Cloud-Agnostic Platform
Labelbox's cloud-agnostic platform ensures compatibility with diverse model training environments and cloud service providers (CSPs), seamlessly connecting to the model training environment for enhanced flexibility and accessibility.
Advantages
1. Time and Cost Efficiency
Leveraging foundational models like GPT-3 significantly saves time and costs compared to training models from scratch.
2. Reinforcement Learning from Human Preferences (RLHP)
Incorporate RLHP framework to enhance ChatBot model performance significantly.
3. Iterative Improvement
Empower users with an iterative workflow to continuously fine-tune ChatBot models based on real-world data priorities, ensuring ongoing improvement and adaptability.
4. Performance Evaluation
Evaluate model performance, identify weaknesses, and iteratively improve by feeding relevant data back into the fine-tuning process.
5. Catalog Integration for Data Prioritization
Utilize Labelbox Catalog features to prioritize data impacting the next training iteration, enhancing the model's ability to address edge cases effectively.
Labelbox's ChatBot Fine-Tuning Tool offers flexibility, efficiency, and iterative improvement, providing a structured approach for optimizing ChatBot models across diverse NLP applications.
The integration with Google Colab Notebook and the cloud-agnostic platform further enhances the user experience, making it an invaluable resource for machine learning teams fine-tuning ChatBot models for specific use cases.
3. Databricks Lakehouse
Databricks Lakehouse presents itself as a comprehensive platform designed to streamline the fine-tuning process of Chatbots, prioritizing distributed training and real-time serving capabilities.
Key Features
1. Ray AIR Integration
Leveraging Ray AIR Runtime for distributed fine-tuning of ChatBot models, allowing efficient scaling across multiple nodes.
Integration with Spark data frames and Hugging Face facilitates seamless data loading.
2. Hyperparameter Tuning with RayTune
Utilizes RayTune for model hyperparameter tuning, ensuring optimal performance tailored to specific use cases through distributed fine-tuning.
3. MLFlow Integration for Model Tracking
Integrates with MLFlow for comprehensive model version tracking and logging, utilizing MLFlow's Transformer Flavor for standardized model storage and checkpointing.
4. Real-Time Model Endpoints
Enables deployment of fine-tuned ChatBot models with real-time serving endpoints on Databricks, supporting both CPU and GPU serving options, with upcoming features for optimized serving of large models.
5. Efficient Batch Scoring with Ray
Demonstrates efficient batch scoring using Ray BatchPredictor, ideal for distributing scoring tasks across instances equipped with GPUs.
6. Low-Latency Model Serving Endpoints
Introduces Databricks Model Serving Endpoints, offering low-latency and managed services for ChatBot model deployments, with GPU serving options and upcoming features for optimized serving of large models.
Advantages
1. Unified Framework
Ray AIR serves as a unifying framework, seamlessly orchestrating various tools such as Spark, Hugging Face, and MLFlow for cohesive ChatBot fine-tuning workflows.
2. Distributed Scalability
Leverages distributed computing capabilities for both training and inference, ensuring efficient scaling of ChatBot models across clusters.
3. Flexible Model Tuning
Provides flexibility in model hyperparameter tuning through RayTune, enabling adaptation of models to specific performance requirements.
4. MLFlow for Model Management
MLFlow integration facilitates comprehensive model versioning, tracking, and logging, enhancing overall model management capabilities.
5. Real-Time Serving
Real-time serving endpoints facilitate immediate deployment of fine-tuned ChatBot models for use in applications, reducing latency and improving user experience.
6. Support for Large Models
Addresses challenges related to GPU memory constraints, offering solutions and recommendations for optimizing resources to accommodate large ChatBot models effectively.
Databricks Lakehouse stands out as a versatile ChatBot fine-tuning platform, offering a range of features and advantages tailored to meet the evolving needs of machine learning teams in optimizing ChatBot models for various applications.
4. Kili
Kili's ChatBot Fine-Tuning Tool is a robust and intuitive platform designed for both individuals and enterprises aiming to optimize the performance of their ChatBot models.
Key Features and Advantages
1. Customizable Evaluation Criteria
Define tailored evaluation criteria such as adherence to instructions, creativity, reasoning, and factuality for effective fine-tuning.
2. Comprehensive Data Labeling
Handle a variety of tasks including classification, ranking, transcription, and dialogue utterances, ensuring high-quality annotations.
3. Advanced Quality Assurance Workflows
Set up advanced QA workflows, implement QA scripts, and detect errors in machine learning datasets to ensure top-notch data annotation.
4. Efficient Feedback Conversion
Overcome noise and information scarcity in user feedback through an advanced filtering system, efficiently converting insights into actionable training data.
5. Seamless Integration with Leading ChatBot Models
Enjoy native integration with a Copilot LLM-powered system for annotation and plug-and-play integrations with market-leading models like GPT, streamlining the fine-tuning process.
6. Access to Expert Annotators
Benefit from access to qualified annotators with industry-specific expertise, ensuring high-quality standards and swift delivery of labeled datasets.
7. Positive User Testimonials
Users praise Kili's user-friendly interface and efficient tools for data labeling and ChatBot fine-tuning, highlighting its effectiveness and ease of use.
Kili's ChatBot Fine-Tuning Tool offers a comprehensive solution, addressing the complexities of the fine-tuning process with its user-friendly interface, customizable features, and access to expert annotators.
5. Label Studio
Label Studio's ChatBot Fine-Tuning Tool is a versatile and robust data annotation platform specifically crafted to streamline the process of optimizing ChatBot models.
This tool serves a crucial role in refining ChatBot models by offering a suite of features tailored to the intricacies of model optimization.
Key Features and Advantages
1. Custom Annotation Tasks
Tailor annotation tasks to the specific requirements of ChatBot fine-tuning, covering tasks such as intent classification, named entity recognition, and semantic text similarity.
2. Support for Multiple Data Formats
Accommodate diverse data types including text, images, audio, and video, catering to the multi-modal nature of ChatBot tasks.
3. Collaborative Annotation
Facilitate teamwork with collaborative annotation capabilities, allowing multiple annotators to work efficiently on the same dataset, ensuring consistency and accuracy.
4. Quality Control
Ensure annotation precision with quality control features including annotation history tracking and disagreement analysis, crucial for maintaining dataset quality.
5. Integration with ML Models
Seamlessly integrate with machine learning models for active learning workflows, leveraging ChatBot models for pre-annotation followed by human correction, enhancing efficiency, and improving fine-tuning outcomes.
Label Studio's ChatBot Fine-Tuning Tool empowers users to efficiently prepare high-quality datasets tailored for ChatBot model optimization.
Through customized annotation tasks, versatile data format support, collaborative features, robust quality control, and seamless integration with machine learning models, Label Studio enables users to unlock the full potential of their ChatBot models.
Conclusion
Fine-tuning ChatBot models is essential for optimizing their performance across various natural language processing tasks.
The tools discussed in this guide – Labellerr, Labelbox, Databricks Lakehouse, Kili, and Label Studio – offer unique features and approaches to streamline the fine-tuning process.
Whether you're a machine learning enthusiast or a professional seeking to enhance your ChatBot's capabilities, these platforms provide invaluable resources.
By leveraging their user-friendly interfaces, customizable workflows, and advanced features, you can unlock the full potential of ChatBots in the evolving landscape of conversational AI.
Frequently Asked Questions
1. What is a chatbot fine-tuning process?
The chatbot fine-tuning process involves iteratively enhancing the performance of a chatbot model through data collection, preprocessing, initial training, and subsequent fine-tuning iterations.
This iterative refinement includes adjusting model parameters, architecture, and training data based on performance evaluation and user feedback.
The process aims to improve various aspects such as response accuracy, conversational flow, and domain adaptation.
Continuously evaluating the model's performance, incorporating user feedback, and deploying the refined model into production ensure a seamless conversational experience over time.
2. How can chatbots be built using large language models?
Chatbots can be built using large language models by leveraging their vast pre-trained knowledge and natural language understanding capabilities.
Large language models, such as GPT (Generative Pre-trained Transformer) models, are trained on massive amounts of text data, allowing them to understand and generate human-like responses to user queries.
To build a chatbot, developers fine-tune the pre-trained language model on specific conversational datasets relevant to the chatbot's domain or use case.
This fine-tuning process adapts the model to understand the nuances of conversational language and generate contextually appropriate responses.
Additionally, developers can integrate the fine-tuned language model into chatbot frameworks or platforms, enabling users to interact with the chatbot through various channels such as messaging apps, websites, or voice assistants.

Simplify Your Data Annotation Workflow With Proven Strategies
Download the Free Guide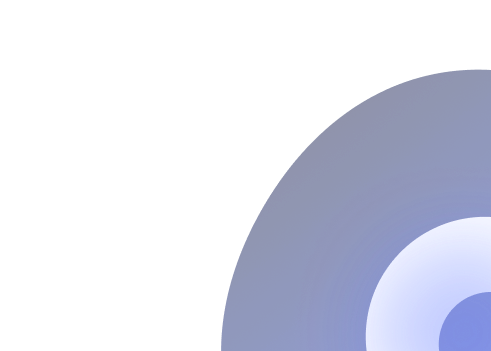