8 Benefits of Using Image Labeling Tools
Image labeling tools enhance AI model training by providing accurate, scalable annotations. They save time through automation features like AI-assisted labeling, reduce manual effort, and handle large datasets efficiently, making them essential for large-scale AI projects
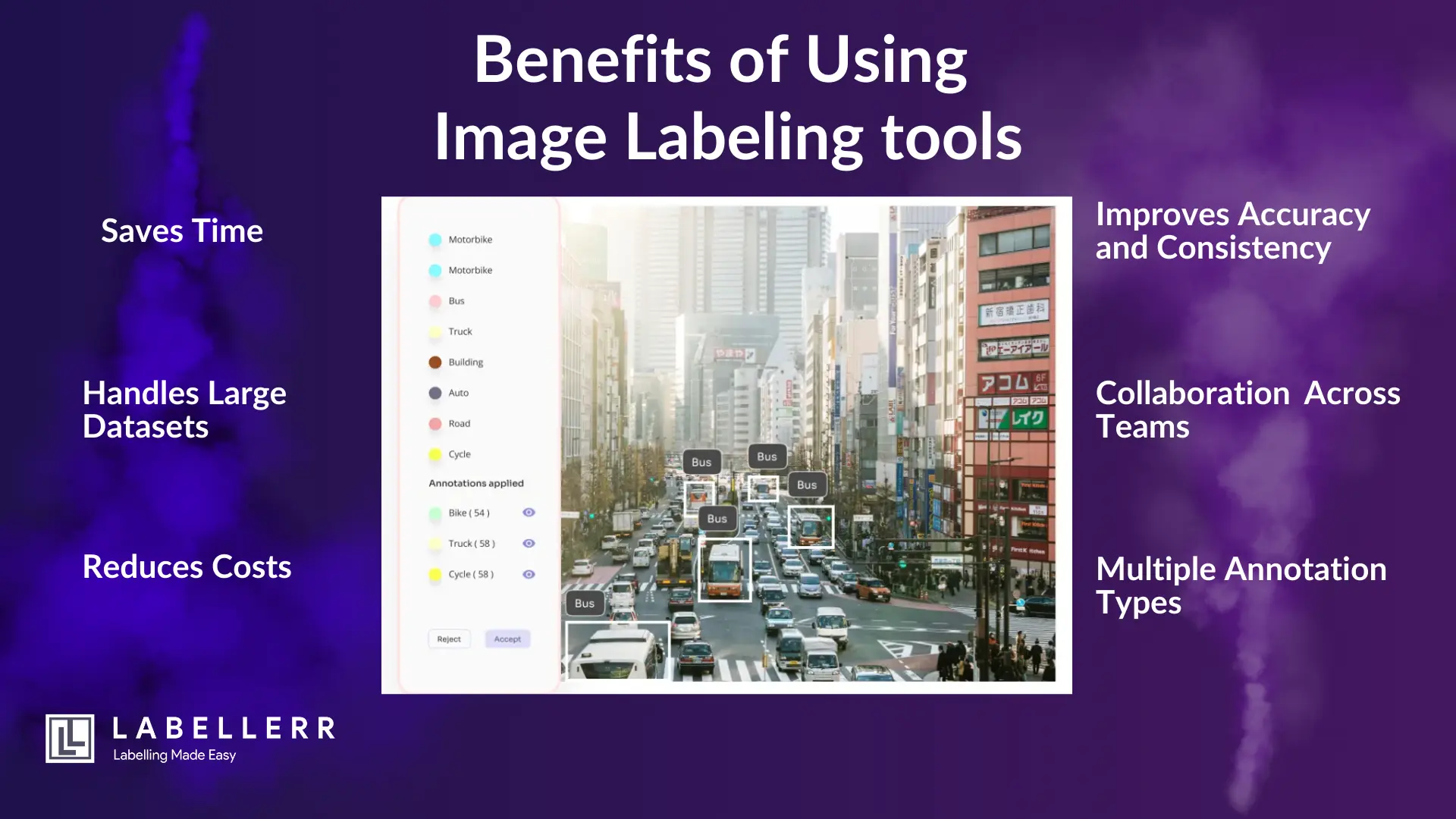
Data preparation, including labeling, accounts for up to 80% of the time spent on AI and machine learning projects. These tools play a critical role in creating reliable datasets, which are the foundation of every machine learning model.
However, manual data annotation comes with its own set of challenges. It is time-consuming, requiring hours of effort to label large datasets. It is also expensive, as manual annotation needs a large workforce, increasing costs.
Additionally, scaling manual processes to handle millions of images is difficult, which slows down AI development and delays project timelines.
Image labeling tools solve these challenges by automating and enhancing the annotation process.
They save time, reduce costs, and ensure consistent and accurate labeling. By addressing the limitations of manual annotation, these tools make it easier for teams to create high-quality datasets and improve the performance of their AI models.
Table of Contents
8 Benefits of Using Image Labeling Tools
1. Saves Time with Automation
These tools automate repetitive tasks like drawing bounding boxes or segmenting objects in images. This automation significantly reduces the time needed for manual annotation.
For example, AI-assisted labeling can cut annotation time by up to 70%, allowing teams to complete projects much faster. Faster annotation not only saves time but also speeds up AI development, enabling teams to meet tight deadlines and launch products sooner.
2. Improves Accuracy and Consistency
They come with features like AI-assisted labeling, confidence scoring, and quality checks that ensure precise and consistent annotations.
These tools also use anomaly detection to flag inconsistent labels, improving the overall quality of the data. Consistent and accurate data is crucial for AI models, as poor-quality annotations can lead to errors in model predictions.
By maintaining high-quality annotations, these tools help ensure that the AI model performs reliably across various tasks.
3. Reduces Costs
Automation provided by them reduces the need for large manual annotation teams, which lowers labor costs.
This cost saving allows businesses to allocate their budgets to other critical areas like model optimization or deployment.
Studies show that using AI-assisted labeling can cut annotation costs by nearly half compared to manual methods. This makes them especially valuable for startups and smaller organizations with limited budgets.
4. Handles Large Datasets Efficiently
They are built to manage datasets with thousands or even millions of images.
Many tools are cloud-based, making it easy to scale without requiring additional infrastructure.
For instance, a retail company labeling millions of product images for recommendation systems can efficiently annotate large datasets using AI-assisted labeling tools.
This scalability is essential for projects that deal with growing datasets or require continuous data updates.
5. Offers Multiple Annotation Types
Modern labeling tools support various annotation types, including:
- Bounding Boxes for object detection.
- Polygons for annotating irregular shapes like rooftops in satellite imagery.
- Semantic Segmentation for labeling every pixel in an image.
- Key Points for marking specific features like facial landmarks or joint positions.
This flexibility allows users to choose the most suitable annotation type for their specific AI use case.
For example, bounding boxes are ideal for simple object detection, while polygons are better for outlining complex or irregular shapes.
With AI-assisted labeling, these tasks become more efficient and less prone to human error.
6. Ensures Collaboration Across Teams
These tools have built-in collaboration features that allow multiple annotators and reviewers to work together seamlessly.
Real-time feedback and updates improve the efficiency and quality of annotations. For example, annotation teams working on autonomous vehicle datasets can collaborate to ensure consistent guidelines are followed across all images.
This collaborative approach, powered by AI-assisted labeling,AI-assisted labeling reduces errors and helps complete projects faster.
7. Provides Seamless Integration with ML Pipelines
They integrate easily with machine learning workflows, supporting popular formats like COCO, YOLO, and Pascal VOC.
This integration reduces friction in transferring labeled data to training pipelines, saving time and effort.
For example, labeled data can be exported directly to cloud platforms like AWS or Google Drive, allowing for smooth data processing and model training using AI-assisted labeling.
8. Future-Proofing with AI-Assisted Features
Advanced tools use AI to adapt to new challenges, such as labeling rare or ambiguous cases.
For example, tools like LabelGPT can auto-label images based on text prompts, reducing the need for human input.
These tools evolve to handle complex datasets, ensuring long-term usability. As AI applications become more sophisticated, AI-assisted labeling tools will remain valuable in addressing emerging annotation needs.
Conclusion
These tools save time by automating repetitive tasks, reduce costs by minimizing the need for large annotation teams, and improve accuracy with features like AI-assisted labeling and quality checks.
These tools make it easier to handle large datasets, ensure consistent annotations, and streamline the entire AI development process.
Looking to speed up your data annotation process?
Contact Labellerr today to explore how we can help automate your workflows and deliver high-quality annotations for your AI projects!
FAQs
1. What are image labeling tools, and why are they important?
Image labeling tools are software platforms that help annotate images by adding labels or tags to objects, regions, or features within the images.
These annotations are essential for training AI and machine learning models to recognize patterns, detect objects, or make predictions.
They improve efficiency, ensure accuracy, and enable scalability for projects requiring large amounts of annotated data.
2. How do these tools save time compared to manual annotation?
They use automation features like AI-assisted labeling, pre-labeling, and smart suggestions to speed up the annotation process.
For example, they can automatically generate bounding boxes, polygons, or masks, reducing the manual effort required. Studies show that these tools can cut annotation time by up to 70%, enabling teams to complete projects faster.
3. Can these tools handle large datasets efficiently?
Yes, most modern tools are designed to handle large datasets with thousands or even millions of images.
Many are cloud-based, which allows for easy scalability without needing additional infrastructure. These tools also support batch uploads, automated workflows, and seamless integration with machine learning pipelines, making them ideal for large-scale projects.
Book our demo with one of our product specialist
Book a Demo