BDD100K: Database of driving videos for self driving cars AI
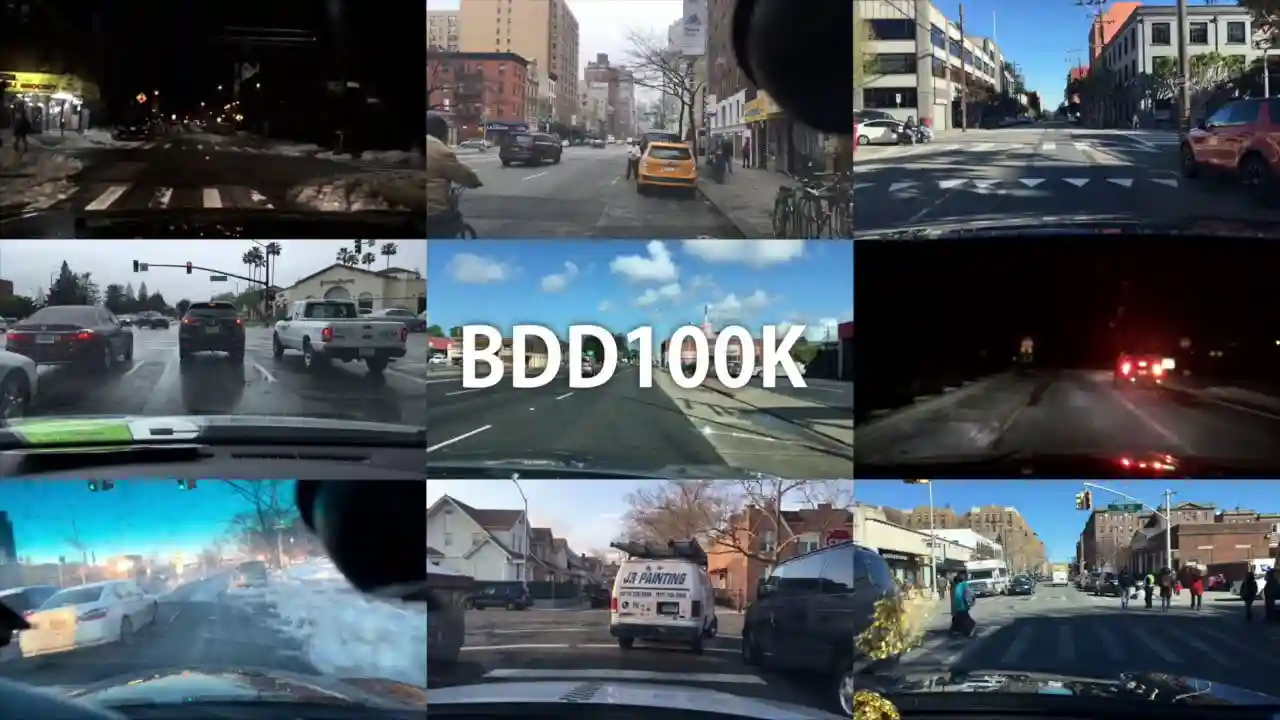
We understand that working on datasets is a tough task but finding correct datasets for your model to get the right results is at par level difficult. But you can make this task much easier by understanding the datasets. If you have a clear idea about the most appropriate option then, definitely, you can reach the optimum level without much effort. Here, we have provided detailed information about the BDD100K dataset. Read till the end to gain complete knowledge.
Table of Contents
- Introduction: BDD100K
- Why Has This Data Set Been Created?
- Some of the Related Research Papers
- How to Download It?
Introduction: BDD100K
The largest public driving video dataset, BDD100K is created by Fisher Yu, Haofeng Chen, Xin Wang, Wenqi Xian, Yingying Chen, Fangchen Liu, Vashisht Madhavan, and Trevor Darrell.
The Berkley DeepDrive 100K Dataset contains all of the instance segmentation, object recognition, driveable area, and lane marking information. It comprises more than 100 000 HD videos shot in a range of lighting conditions and seasons. Data on localization, timestamps, and IMU are included in the collection.
Data were gathered in four places, three of which were adjacent to one another (SF, Berkeley, Bay Area), with New York serving as the fourth.
In this dataset, there are only 10 object categories:
- bike
- bus
- car
- motor
- person
- rider
- traffic light
- traffic sign
- train
- truck
Instance segmentation categories:
- banner
- billboard
- lane divider
- parking sign
- pole
- pole group
- street light
- traffic cone
- traffic device
- traffic light
- traffic sign
- sign frame
- person
- rider
- bicycle
- bus
- car
- caravan
- motorcycle
- trailer
- train
- Truck
Drivable area categories:
- area/alternative
- area/drivable
Lane marking categories:
- lane/crosswalk
- lane/double other
- lane/double white
- lane/double yellow
- lane/road curb
- lane/single other
- lane/single white
- lane/single yellow
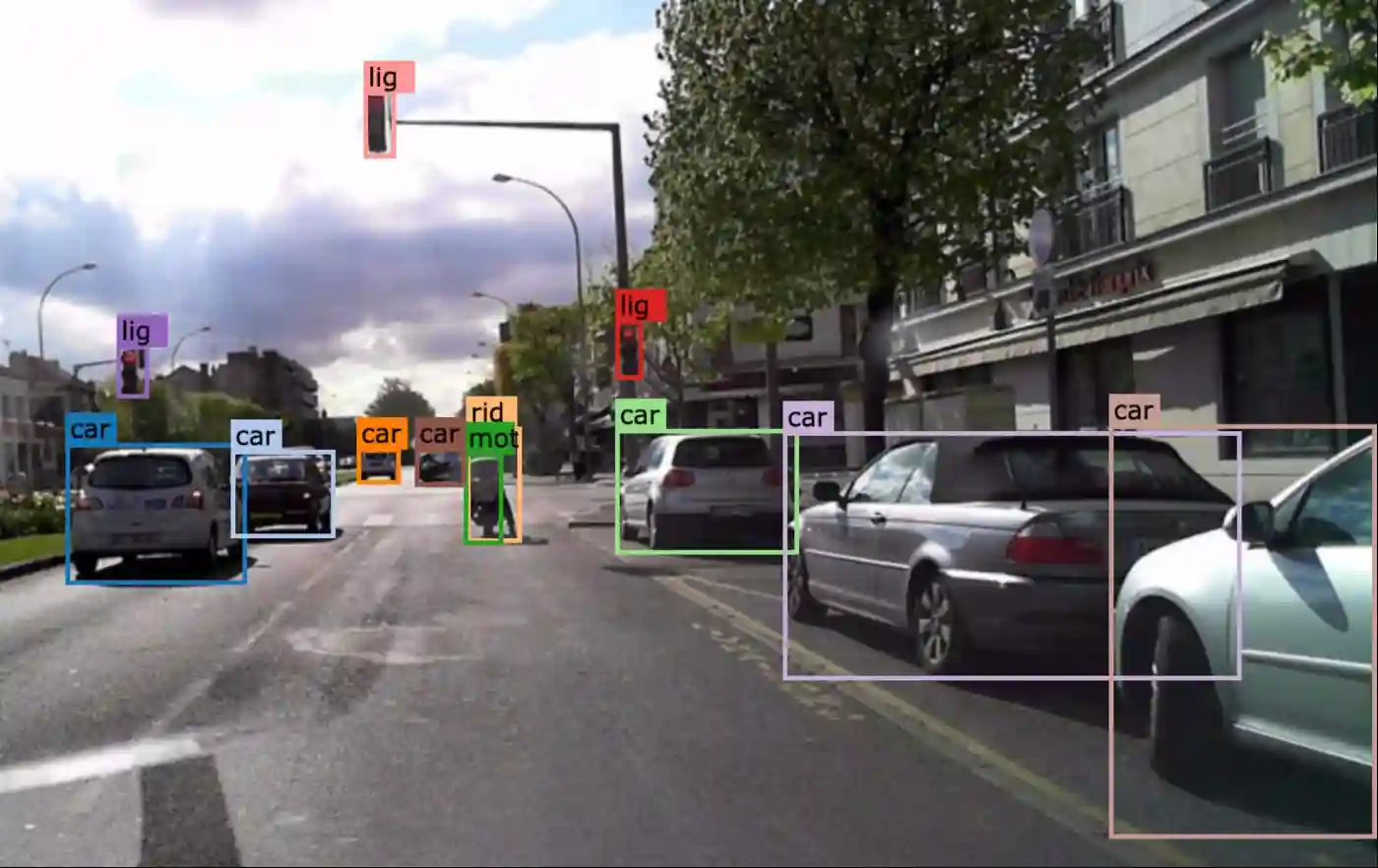
This data set contains 100K films and 10 tasks for assessing the fascinating advancements in image recognition algorithms for autonomous driving. Each video is in high resolution and 40 seconds long.
The collection, which contains over 100 million frames, reflects more than 1000 hours of traveling time.
The videos include trajectory data from the GPU and IMU. The dataset's geographic, ecological, and weather diversity is helpful for developing models that are less susceptible to being caught off guard by novel circumstances.
The perceptual difficulties are made more difficult by the complex ego-vehicle mobility and the changing external views.
The data set collection includes a variety of scenario types, including motorways, residential areas, and city streets.
The videos were also shot at distinct periods of the day and in a variety of weather situations.
There are three sets of videos: a training set (70K), a validation set (10K), and a testing set (20K). Each movie has a frame annotated at the 10-second mark for image tasks, and the complete sequence is utilized for tracking tasks.
Why has this data set been created?
The data set was created to offer a sizable, diverse driving video collection with in-depth annotations that can highlight the difficulties in comprehending street scenes.
The data set is collected through movies, crowdsourcing, supported by Nexar 2, and a large number of drivers to produce good diversity.
The file additionally includes GPS/IMU recordings to retain the driving trajectories in addition to high resolution (720p) and high frame rate (30fps) photos.
We have collected 100K driving movies (each lasting 40 seconds) from more than 50K journeys, spanning the New York City, San Francisco Bay Area, and some other places.
Some of the related research papers
Here are some related research papers that you can utilize for your research.
1. Ortiz Castelló, V., Salvador Igual, I., del Tejo Catalá, O., & Perez-Cortes, J. C. (2020). High-Profile VRU Detection on Resource-Constrained Hardware Using YOLOv3/v4 on BDD100K. Journal of imaging, 6(12), 142.
In this paper, they have explained the significant use of object detection is the identification of vulnerable road users (VRUs) with the goal of reducing accidents in advanced systems for driver assistance and facilitating the creation of autonomous cars. For these reasons, the well-known YOLOv3 net and the new YOLOv4 one are evaluated by training them on a large, recent on-road image dataset (BDD100K), for both VRU and full on-road classes, with a significant improvement in terms of recognition quality when compared to their authors' MS-COCO-trained generic correspondent models, but at a negligible cost in forward pass time.
This study compares the performance of Yolo-v4 and the deconvolutional single shot multibox detector (DSSD), two popular real-time object identification techniques, in a realistic driving situation. Each neural network is first trained using the 80-class COCO dataset, then it is then fine-tuned using the BDD100k dataset. The True/False Positive Results, Precision-Recall Curve, Average Precision @ Intersection of Union 50, and Average Precision Intersection of Union 75 outcomes are used to compare the detection results.
3. V2X-Sim: Multi-Agent Collaborative Perception Dataset and Benchmark for Autonomous Driving -By Yiming Li, Dekun Ma, Ziyan An, Zixun Wang, Yiqi Zhong, Siheng Chen, Chen Feng
This research paper explains how Vehicles and numerous other things in the surrounding environment can work together due to vehicle-to-everything (V2X) communication systems, which could significantly enhance the autonomous driving perception system. V2X-Sim, a thorough simulated multi-agent perception dataset for V2X-aided autonomous driving, was developed to close this gap. In order to enable collaborative perception, V2X-Sim offers "multi-agent" sensor records from the roadside unit (RSU) and several cars, as well as multi-modality sensor streams and a variety of ground truths to support different perception tasks.
4. Multi-task Learning for Visual Perception in Automated Driving-By Sumanth Chennupati
That talks about Modern advances in deep learning and computer vision along with top-notch detectors like cameras and LiDARs, have powered sophisticated visual perception technologies. The lack of sufficient processing capacity to create real-time applications is the key hurdle for these technologies. The trade-off between efficiency and run-time optimization is frequently caused by this bottleneck and has emphasized these bottlenecks.
5. Towards Recognition as a Regularizer in Autonomous Driving-By Aseem Behl
Have mentioned various strategies for utilizing semantic cues from cutting-edge recognition techniques to enhance the efficiency of other tasks necessary to address autonomous driving. The performance of identification as a regularizer in 2 main autonomous driving issues, namely scene flow estimations and end-to-end learned autonomous driving, is thus examined in this thesis.
How to download it?
You can access the BDD100K data and annotations at https://bdd-data.berkeley.edu/. Once you've accepted the BDD100K license, all you have to do is sign in there and download the files using your browser. A list of download links with names that relate to the subcategories on this page can be found on the downloading site. In this package, there are 10,000 photos used for panoptic, instance, and semantic segmentation. Not all of the images on this page have associated videos due to some structural problems. There is a lot of overlap, but it's not a fraction of the 100K photos.
You can also take a quick preview of the dataset on Labellerr's platform.
- To facilitate downloading, the 100K videos were divided into 100 parts.
- The frames from the videos' tenth second make up the visuals in this package. With the entire video collection, the train, verification, and testing set are divided in the same way. They are employed for lane marking, driving areas, and object detection. (Size 5.3GB)
- Road object detection annotations were released in JSON format in 2018. The downloadable JSON files also contain the video properties, such as scene, time of day, and weather. We updated the detection annotation in 2020 and made them available in the list as Detection 2020 Labels. It is advised that you make use of the new labeling. For use in comparison with previous findings, this recognition annotation set is retained.
Book our demo with one of our product specialist
Book a Demo