What Are Large Language Models & Its Applications
Large language models (LLMs) are transformative AI tools trained on vast datasets to perform language-based tasks with human-like precision. From powering chatbots to aiding content generation and translation, LLMs redefine NLP applications with their advanced contextual understanding.
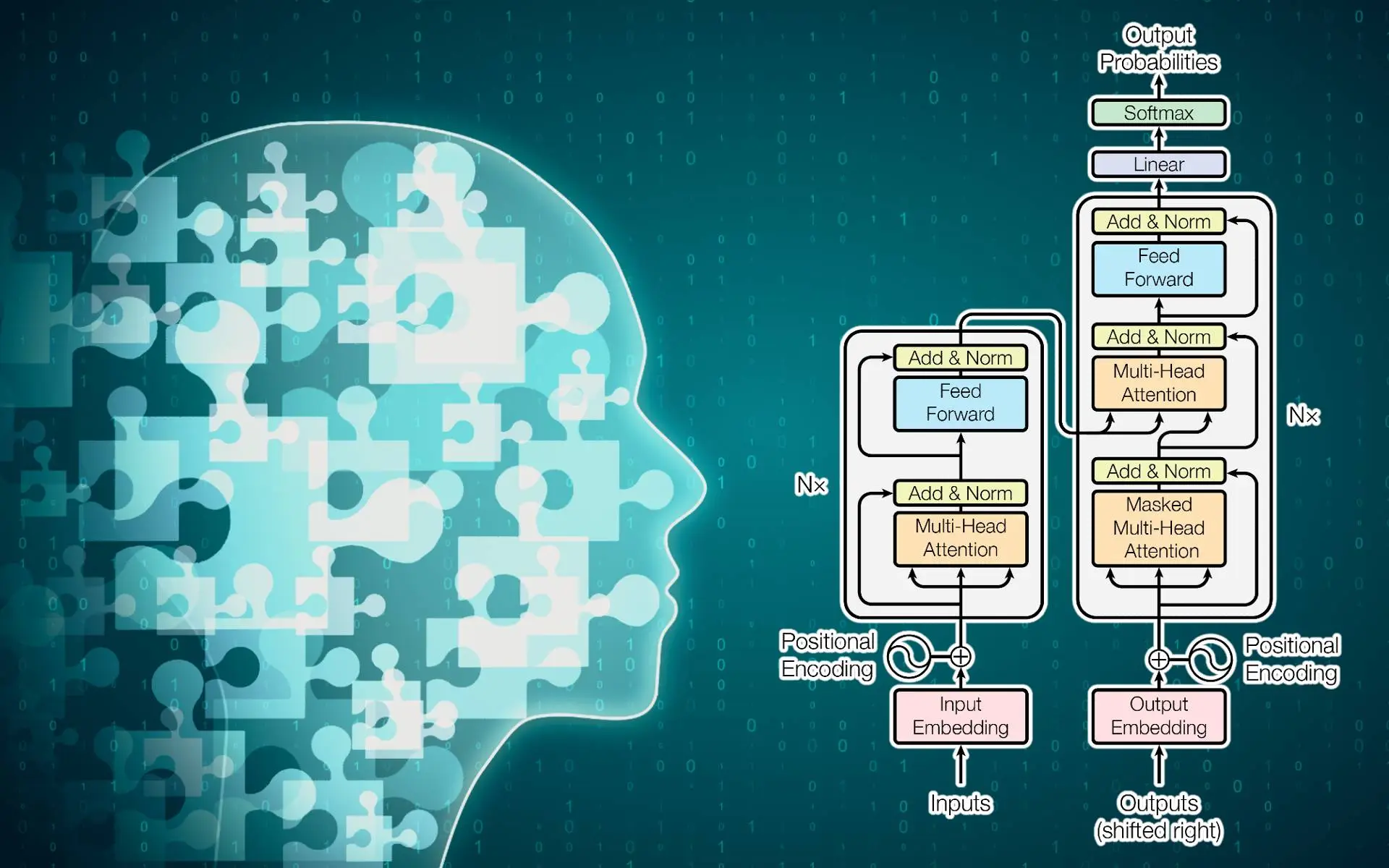
Imagine a world where machines can write essays, hold meaningful conversations, translate languages instantly, and even create poetry—just like humans. This isn’t science fiction; it’s the power of Large Language Models (LLMs).
In 2022, OpenAI’s GPT-3 astounded the tech world by generating text so human-like that distinguishing it from real writers became a challenge. Fast forward to 2024, GPT-4 processed over 1 trillion words, setting a new standard for AI language models. But this is just the beginning.
LLMs are revolutionizing Natural Language Processing (NLP) by excelling at tasks like:
- Generating human-like text for chatbots and content.
- Translating languages with near-native fluency.
- Summarizing complex documents in seconds.
The global large language model market size was estimated at USD 4.35 billion in 2023 and is projected to grow at a CAGR of 35.9% from 2024 to 2030.
But what makes LLMs so transformative? How do they work, and why are they hailed as a cornerstone of modern AI innovation?
In this blog, we’ll unravel the fascinating world of LLMs, explore their groundbreaking applications, and tackle the challenges they present.
Let’s dive in and uncover the potential of large language models!
What are Large Language Models (LLMs)?
Using self-supervised or semi-supervised learning, large language models (LLMs), which are language models made up of neural networks with billions of parameters, are trained on massive amounts of unlabeled text.
LLMs are general-purpose models that excel at various tasks instead of being trained for a single job.
LLMs are used in generative AI chatbots like ChatGPT, Google Gemini, and Microsoft Copilot to produce responses that resemble those of human beings.
LLMs produce human-like responses to questions by combining deep learning and natural language generation algorithms with a large text library.
LLMs process language using a transformer neural network architecture, specifically designed for language tasks, and are trained on massive volumes of data.
What Is The Difference Between LLMs And Traditional Language Models?
Large Language Models (LLMs) are learned utilizing self-supervised or semi-supervised learning on enormous amounts of unlabeled text, unlike traditional language models, which are trained on labeled data.
LLMs are general-purpose models that perform well across a variety of applications, as opposed to being trained for a single job.
LLMs respond to human-like prompts by combining deep learning and natural language generation techniques with an extension text library.
LLMs are trained by cutting-edge machine learning algorithms to understand and analyze the text, unlike traditional language models pre-trained by academic institutions and major tech corporations.
LLMs are self-training, thus they get better the more input and usage they receive.
Applications of Large Language Models
Artificial intelligence (AI) and natural language processing (NLP) both have several uses for large language models (LLMs). Based on information from massive datasets, LLMs can recognize, condense, translate, forecast, and even produce human-like words and other content like photos and audio.
LLMs have displayed exceptional performance across a variety of NLP tasks, including text generation and completion, sentiment analysis, text classification, summarization, question answering, and language translation.
Based on a given prompt, LLMs can produce logical and contextually relevant language, providing new opportunities for creative writing, social media content, and other uses. LLMs can also be used in chatbots, virtual assistants, and other conversational AI applications.
LLMs are widely applicable for a variety of NLP activities and can be used as the basis for unique use cases. An LLM can be enhanced with further training to produce a model well-suited to an organization's unique requirements.
How Are LLMs Created? What Is Their Architecture?
LLMs are typically constructed utilizing deep learning techniques, specifically neural networks to process and learn from enormous volumes of data. At the fundamental layer, an LLM needs to be trained on a substantial amount of data, often measured in petabytes.
The training can proceed in several stages, typically with an unsupervised learning strategy. In that method, the model is trained on the data without the involvement of a human.
On the basis of data from substantial datasets, LLMs are able to recognize, condense, translate, forecast, and even produce human-like texts as well as other types of information like photos and audio.
You must have come across the fact that LLMs are largely dependent on deep learning techniques. What are those techniques? Let’s explore!
Large Language Models (LLMs) are built using advanced deep learning techniques, particularly neural networks, to process and learn from massive amounts of data efficiently.
Transformer models, recurrent neural networks (RNNs), and convolutional neural networks (CNNs) are some of the most popular deep-learning methods used to build LLMs.
Transformer models, like Google's BERT and OpenAI's GPT, have grown in popularity as a result of their capacity to process massive volumes of data and produce text of a high standard.
RNNs are frequently employed for sequence-to-sequence tasks like text summarization and language translation.
For tasks like text categorization and sentiment analysis, CNNs are frequently utilized. Depending on the objective and dataset, LLMs can also be created using a combination of these methods.
Some of the Popular Large Language Models (LLMs)
The models are trained on massive datasets, which makes them versatile and have found applications across industries. Below, we explore five prominent LLM models and their detailed applications.
1. GPT Models (Generative Pre-trained Transformers)
GPT models, developed by OpenAI, are among the most recognized LLMs. These models are trained using transformer architecture and are designed for natural language understanding and generation tasks.
Applications
- Content Creation: GPT models are used to generate articles, blogs, creative writing, and even poetry. Tools like ChatGPT exemplify their capability in assisting with marketing copy and social media content.
- Customer Support: Businesses deploy GPT-based chatbots to provide 24/7 customer support, answering FAQs, troubleshooting, and managing user interactions.
- Code Generation: With capabilities to understand programming languages, GPT models assist in writing and debugging code, often used by developers for rapid prototyping.
- Language Translation: GPT can be applied to real-time translation tasks, enhancing communication across different languages.
- Education and Tutoring: It serves as a virtual tutor, helping students with explanations, generating learning materials, and providing test preparations.
2. BERT (Bidirectional Encoder Representations from Transformers)
BERT, developed by Google, is a bidirectional model focused on understanding the context of a word based on its surroundings. It is widely used for tasks requiring language comprehension rather than generation.
Applications
- Search Engine Optimization (SEO): Google integrates BERT to improve search result relevance by better understanding user queries.
- Question Answering Systems: BERT powers applications that provide accurate answers to user queries, like knowledge-based retrieval systems.
- Sentiment Analysis: Businesses use BERT to analyze customer reviews and feedback, helping them gauge public opinion about their products or services.
- Document Summarization: BERT extracts key points from lengthy documents, saving time and improving information processing.
- Healthcare Data Analysis: It helps healthcare professionals analyze unstructured data like medical records and research articles.
3. T5 (Text-to-Text Transfer Transformer)
T5, created by Google, reframes all NLP tasks into a text-to-text format. Its flexible architecture allows it to handle diverse tasks effectively.
Applications
- Text Summarization: T5 is used for condensing lengthy reports, articles, or papers into concise summaries, useful in business and academia.
- Question Generation: This model generates questions based on given content, aiding in education and training material creation.
- Language Translation: It excels in multilingual translations, making it valuable for global communication and e-commerce platforms.
- Chatbot Development: T5’s generative capabilities support conversational AI in creating interactive and intelligent chatbots.
- Code Documentation: Developers use T5 to generate descriptions and documentation for codebases automatically.
4. LLaMA (Large Language Model Meta AI)
Developed by Meta, LLaMA focuses on efficiency by being smaller in size compared to models like GPT, while maintaining competitive performance.
Applications
- Academic Research: LLaMA supports researchers in summarizing papers, generating hypotheses, and organizing literature reviews.
- Low-Resource Language Support: It provides NLP capabilities for underrepresented languages, contributing to inclusivity in AI tools.
- Knowledge Base Management: Businesses use LLaMA to organize and retrieve knowledge from vast corporate datasets.
- Interactive Virtual Assistants: It powers digital assistants, offering enhanced conversational capabilities.
- Creative Writing Tools: Writers leverage LLaMA for idea generation, drafting, and brainstorming sessions.
5. BLOOM (BigScience Large Open-Science Open-Access Multilingual Language Model)
BLOOM is a multilingual language model designed to handle over 40 languages and dialects. It is an open-access project emphasizing inclusivity and collaboration.
Applications
- Multilingual Content Creation: BLOOM generates content in multiple languages, aiding global businesses in reaching diverse audiences.
- Cultural Preservation: It supports lesser-known languages, contributing to their digital preservation and accessibility.
- Legal Document Processing: BLOOM automates the summarization and analysis of multilingual legal documents.
- Global Education Platforms: The model enables the creation of multilingual educational resources for a broader reach.
- Community-Driven AI Applications: Its open-access nature allows developers worldwide to create custom NLP tools tailored to local needs.
Conclusion
Large language models (LLMs), a ground-breaking advancement in artificial intelligence, can fundamentally alter how we interact with language.
LLMs are already being used in a variety of applications, from chatbots and virtual assistants to language translation and content production, thanks to their capacity to produce text that resembles human speech and carry out a number of language-related tasks.
But just like any new technology, LLMs also pose important moral and societal issues that must be resolved. It is essential that, as we seek to expand the capabilities of LLMs, we also consider their broader implications and fight to build a more responsible and equitable future for this ground-breaking technology.
Read our latest article on Llama 3 and its application.
FAQs
Q 1: What are Large Language Models (LLMs)?
Machine learning models called Large Language Models (LLMs) are trained on a huge amount of data to learn how to represent language. To comprehend the context and meaning of language, these models take advantage of cutting-edge natural language processing techniques, such as the self-attention mechanism.
Q 2: What is the self-attention mechanism used in LLMs?
The self-attention mechanism is a crucial component of LLMs that enables the model to give various portions of the input text varying weights based on how relevant they are to the job at hand. LLMs better understand the context and meaning of the input text because of this approach.
Q 3: What are some use cases for LLMs?
LLMs can be used for a variety of tasks involving natural language processing, including sentiment analysis, question answering, text production, and text summarization. They are utilized more frequently in programs like chatbots, virtual assistants, and content production software.
Q 4: What is fine-tuning in the context of LLMs?
During the fine-tuning procedure, a pre-trained LLM is further trained in a particular task or area. This enhances the model's performance by allowing it to better adapt to the particulars of the task or domain.
Q 5: What are some challenges associated with building and training LLMs?
High-quality data, powerful computers, and specialized machine-learning knowledge are all necessary for developing and training LLMs. Due to these difficulties, questions have been raised regarding the use of LLMs, their potential for prejudice, and their environmental and ethical implications.
Book our demo with one of our product specialist
Book a Demo