Measure False Positive to Object Detected In One Click With Labellerr
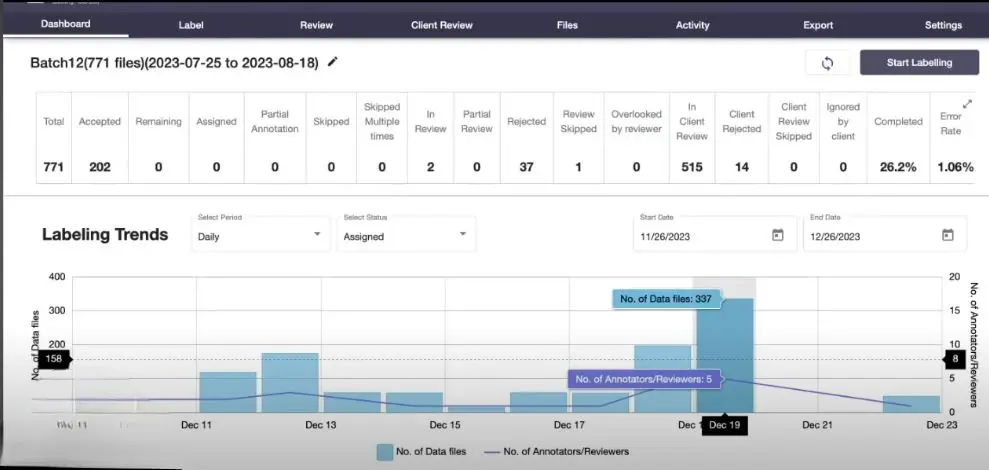
Picture yourself as a data analyst tasked with assessing the accuracy of annotations within a dataset. Analyzing false positives in overall dataset is very crucial for quality assurance.
Without Labellerr's latest feature update, your evaluation process is hindered by inaccurate error rate calculations that fail to provide a precise understanding of errors within individual files.
This lack of clarity could lead to misinterpretations and compromises in data quality assessment.
What problem does it solve?
In the absence of Labellerr's updated error rate calculation feature, data analysts face significant challenges in accurately assessing the quality of annotations within files.
Error rate is a critical metric in annotation datasets as it provides valuable insights into the accuracy and reliability of the annotations. In data annotation, where human annotators manually label objects or entities within the data, errors are inevitable.
Traditional error rate calculations based on total file counts are often unclear which causes misleading assessments and potentially overlooking critical issues.
These errors can range from mislabeling objects to inaccurate boundaries, and their presence can significantly impact downstream tasks such as machine learning model training or data analysis.
Advantages of using Error rate
Clear and Accurate Assessment: Labellerr's updated error rate calculation focuses on mistakes within individual files, providing customers with a clear and accurate view of the real error rate.
Improved Data Quality Evaluation: This adjustment ensures that customers can precisely evaluate the quality of annotations within each file, enabling them to identify and address errors more effectively.
Enhanced Decision Making: Labellerr's updated error rate calculation feature allows customers to make more informed decisions based on a comprehensive understanding of annotation accuracy.
Increased Transparency: The updated error rate calculation feature enhances transparency in data quality evaluation by providing customers with a clear and accurate representation of annotation errors within files.
Customizable Error Rate Analysis: Labellerr's error rate calculation feature offers flexibility for customers to customize error rate analysis based on their specific requirements.
How to see the error rate in Labellerr:
- Once all annotations have been completed, navigate to the Labellerr dashboard, where you'll find an overview of the error rate.
2. By clicking on the expand icon, you can access detailed information regarding the number of annotations where mistakes were made and annotations with remarks.
This feature allows organizations to set a minimum acceptable error rate percentage, which makes sure data quality standards are maintained.
Conclusion
In summary, Labellerr's updated error rate calculation feature revolutionizes data quality evaluation by providing customers with a clear and accurate view of annotation errors within individual files.
By focusing on mistakes at a granular level, customers can make more informed decisions, improve data quality, and drive better outcomes in their data annotation projects.
For a more hands-on tutorial, you can watch the following video on YouTube:
Frequently Asked Questions
Q1) What is error rate in the context of data annotation?
Error rate refers to the proportion of errors or inaccuracies present in annotated data compared to the total number of annotations. It provides a measure of the reliability and accuracy of the annotations within a dataset.
Q2) How does Labellerr calculate error rate?
Labellerr calculates the error rate by considering mistakes within individual files and comparing them to the total number of objects annotated within those files. This approach offers a more accurate assessment of annotation quality.
Q3) Why is the error rate important in data annotation projects?
The error rate is crucial for evaluating the quality of annotated datasets. It helps users understand the reliability of the annotations, identify areas for improvement in annotation processes, and make informed decisions regarding dataset usability and suitability for various tasks.
Book our demo with one of our product specialist
Book a Demo