5 Smart Waste Management Use Cases Using Vision AI
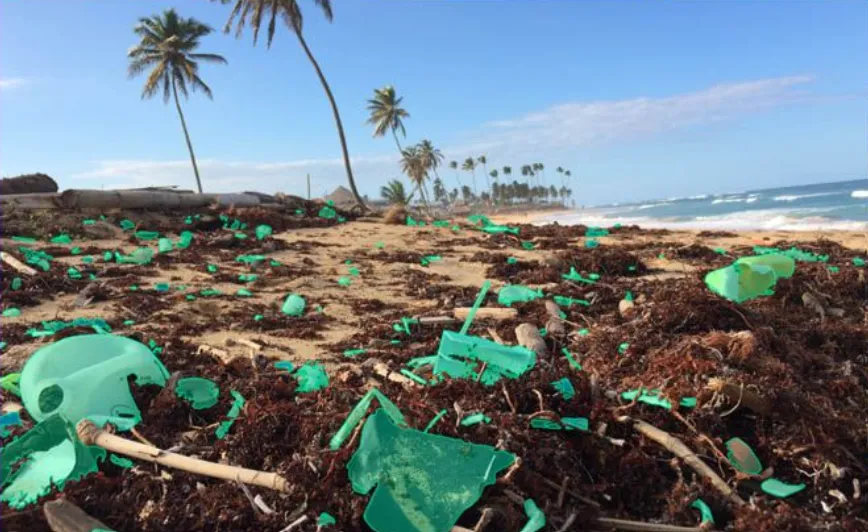
Waste management is an ever-escalating global challenge, with the World Bank predicting that annual waste production will soar to a staggering 3.4 billion tonnes within the next 30 years. As the volume of waste steadily climbs, waste management authorities and private businesses face mounting pressure to manage and process this waste efficiently.
Inadequate waste management not only jeopardizes the environment but also invites criticism from concerned citizens and consumers. To overcome these challenges, the waste management industry is increasingly turning to artificial intelligence (AI) and machine learning, empowered by computer vision. These technologies promise to make waste management safer, more cost-effective, and faster.
However, for AI in waste management to reach its full potential, it crucially depends on high-quality annotated training data. In this blog, we will delve into the four key challenges that AI is helping to address in waste management, and how data annotation tools are instrumental in achieving these goals.
Table of Contents
- Sorting Hazardous Waste
- Identifying Markings
- Automated Recycling
- Waste Spotting Drones
- Medical and Biohazard Waste Management
- Conclusion
- Frequently Asked Questions
Crucial Roles of Data Annotation in Waste Management
I. Sorting Hazardous Waste
Sorting hazardous waste, such as chemical and biohazardous materials, poses significant risks to human workers. Mishandling these materials can result in severe harm or even fatalities. To mitigate these dangers, AI-powered robots are being deployed to autonomously identify and safely remove harmful waste items, reducing human involvement.
However, ensuring the reliability and safety of these AI systems is paramount. This is where data annotation tools come into play. Data annotation tools offer advanced project management features that ensure tasks are assigned to the most proficient annotators.
Detailed performance metrics help maintain high annotation standards, which is particularly essential when safety is a top priority. These tools assist in perfecting the accuracy of annotated AI training images and videos, thereby enhancing the safety of waste sorting operations.
Automated Waste Sorting: Waste sorting facilities can benefit greatly from AI-driven automation. Object recognition AI models, in conjunction with robotic systems, can precisely and tirelessly sort through large volumes of waste. They can separate recyclables from non-recyclables, ensuring that valuable materials are recovered, reducing contamination, and increasing recycling rates.
Impact of Data Annotation in Sorting Hazardous waste
1. Data annotation ensures high-quality training data for AI-powered robots, enhancing their ability to safely identify and remove hazardous waste items.
2. It reduces the risk to human workers and minimizes the chances of accidents.
Method: Image and Video Annotation
1.Collect a diverse dataset of hazardous waste items, including images and videos.
2. Use data annotation tools to label and annotate these items, highlighting hazardous components.
3. Utilize advanced features of data annotation tools for task assignment and quality control.
4. Continuously review and refine annotations to improve accuracy.
5. Create a labeled dataset for training AI models.
II. Identifying Markings
Many packaging materials and waste items bear markings that convey information about their content and proper handling. AI models equipped with computer vision capabilities can efficiently process this visual information, enabling the streamlined sorting of waste products. AI excels at identifying small, predictable visual cues, surpassing human capabilities in speed and precision.
Data annotation tools further expedite the process of annotating images and videos. They incorporate features like linear interpolation, which automatically tracks objects and markings across frames of video data. This accelerates the annotation process, ensuring that AI systems can efficiently identify and categorize waste items based on their markings.
Recycling Optimization: Waste materials often have markings or labels that provide information about their re-cyclability or appropriate processing methods. AI models trained on annotated data can recognize these markings quickly and accurately. This helps streamline the recycling process by automatically sorting materials into appropriate categories, reducing the amount of waste sent to landfills.
Impact of Data Annotation in Identifying Markings
- Data annotation enables AI models to recognize markings on waste items, facilitating efficient sorting and handling.
- It enhances the speed and precision of the sorting process.
Method: Image Annotation with Linear Interpolation
- Gather a diverse dataset of waste items with markings.
- Utilize data annotation tools to annotate the images, highlighting and categorizing markings.
- Apply linear interpolation to track markings across video frames, if applicable.
- Review and validate annotations to ensure accuracy.
- Create a labeled dataset for AI model training.
III. Automated Recycling
Recycling facilities are under constant pressure to manage increasing volumes of waste efficiently. AI, particularly with its object recognition capabilities, can automate and expedite the sorting process. However, the success of object recognition systems hinges on access to large quantities of precisely annotated training data.
Data annotation tools support AI developers in generating metadata, including hierarchical structures. This metadata is vital for training AI systems to accurately and effectively categorize recyclable materials. By simplifying the annotation process and ensuring data quality, these tools contribute to the advancement of recycling automation, making the recycling process more efficient and sustainable.
Impact of Data Annotation in Automated Recycling
- Data annotation helps create metadata and hierarchical structures for AI systems, improving the categorization of recyclable materials.
- It streamlines the recycling process, reducing waste sent to landfills.
Method: Image Annotation with Metadata
- Gather a large dataset of recyclable materials.
- Annotate the dataset, including metadata about the recyclability of each item.
- Utilize data annotation tools to ensure consistency and quality in annotations.
- Create hierarchical structures to categorize recyclables.
- Develop a comprehensive dataset for training AI models.
IV. Waste Spotting Drones
Landfill zones can span vast areas, making them challenging to navigate and survey. To address this issue, many waste management organizations employ aerial drones equipped with computer vision systems. These drones can accurately identify specific types of waste, such as plastic debris floating on the ocean's surface.
This technology is invaluable for efficiently locating and addressing waste-related issues. Video annotation plays a crucial role in drone-based AI applications. Data annotation tools simplify this process by enabling multiple annotators to collaborate on a single piece of footage simultaneously. Once their work is complete, the annotated sections can be seamlessly merged, creating comprehensive datasets for AI training.
This collaborative annotation process enhances the accuracy and effectiveness of waste-spotting drones.
Landfill Monitoring: Drones equipped with AI systems can conduct aerial surveillance of landfill sites. These AI systems can automatically detect and locate waste within the landfill, providing real-time data to site operators. This information can be used to optimize waste disposal and space utilization in landfills, leading to better management practices.
Ocean Plastic Detection: Drones can also be employed to monitor and detect plastic waste in the oceans. AI algorithms can analyze the images and videos captured by drones to identify floating debris, enabling more efficient cleanup efforts and raising awareness about the extent of plastic pollution in our oceans.
Impact of Data Annotation on Waste Spotting Drones
- Data annotation enhances the accuracy and effectiveness of waste spotting drones by creating comprehensive datasets for AI training.
- It enables real-time identification and location of waste in large areas.
Method: Video Annotation with Collaboration
- Capture video footage from waste spotting drones.
- Use data annotation tools to allow multiple annotators to collaborate on the same footage.
- Annotate specific types of waste and their locations.
- Merge annotated sections to create a complete dataset.
- Ensure consistency and accuracy in the annotations for effective drone-based waste spotting.
V. Medical and Biohazard Waste Management
AI for Biohazard Waste Handling: AI-powered systems, when trained with video annotation data, can play a critical role in handling medical and biohazard waste. They can identify and categorize different types of biohazard waste, ensuring proper disposal procedures are followed. This reduces the risk to human workers who would otherwise be exposed to dangerous materials.
Impact of Data Annotation in Medical and Biohazard Waste
- Data annotation helps AI systems in identifying and categorizing biohazard waste, reducing the risk to human workers.
Method: Video Annotation for Biohazard Waste
- Collect video data showing various types of medical and biohazard waste.
- Annotate the video frames to identify and categorize different waste types.
- Ensure that annotations include information about proper disposal procedures.
- Verify the quality and accuracy of annotations.
- Create a labeled dataset for training AI systems in biohazard waste handling.
LabelGPT for Faster Data Annotation in Waste Management
In the dynamic field of AI applications for waste management, we are thrilled to introduce LabelGPT, a revolutionary prompt-based labeling tool that has the potential to revolutionize data annotation for waste management use cases.
Initially designed for broader applications, LabelGPT's capabilities can be harnessed effectively to address the unique challenges in waste management. Here's how it can be a game-changer:
Import Waste Management Data: Seamlessly connect your waste management-related images and videos from various sources, including waste sorting facilities, recycling plants, landfill sites, and drone footage, to the LabelGPT platform.
Specify Labeling Requirements: Provide clear text prompts that outline the elements within the waste management content that require labeling. Choose from various labeling types, such as hazardous waste identification, recycling material categorization, and landfill monitoring.
Effortless Review: In a matter of minutes, LabelGPT will generate accurately labeled waste management content and provide confidence scores. These scores ensure the precision and reliability of the annotations, crucial for safety and efficiency. You can easily review and validate the labels.
LabelGPT offers a powerful solution for enhancing the accuracy and speed of AI applications in waste management, contributing to safer and more effective waste handling and recycling processes.
Conclusion
The integration of AI and machine learning into waste management holds immense promise for addressing the escalating global waste crisis. Data annotation tools, such as LabelGPT, are indispensable in supporting these innovations by ensuring the precision and reliability of AI systems.
By enhancing waste sorting safety, optimizing the identification of markings, automating recycling processes, and facilitating efficient waste spotting using drones, data annotation tools are pivotal in making waste management safer, more cost-effective, and environmentally sustainable.
As waste generation continues to surge, the synergy between AI and data annotation tools offers a promising solution to this age-old challenge, revolutionizing the way we manage and reduce waste for a more sustainable future.
Frequently Asked Questions
1. Can artificial intelligence improve waste management systems for smart cities?
The integration of artificial intelligence with chemical analysis enhances waste pyrolysis, refines estimates of carbon emissions, and optimizes energy conversion processes. Furthermore, we elaborate on how AI can enhance efficiency and lower expenses within waste management systems designed for smart cities.
2. How AI based waste management works?
Artificial intelligence has the potential to revolutionize various aspects of waste management, spanning from garbage collection and transportation to central oversight and efficient surveillance. Consequently, AI-powered smart solutions can replace conventional methods of waste management and manual monitoring. Surprisingly, there have been limited in-depth assessments of AI-driven waste management systems.
3. How can AI improve waste management?
Artificial intelligence can play a crucial role in tracking the advancement of individual tasks within the waste management process and detecting possible points of congestion. Through the identification of these bottlenecks, AI can contribute to optimizing the efficiency of waste management operations. This optimization is facilitated through Machine Vision, which automates waste recognition and sorting.
Book our demo with one of our product specialist
Book a Demo