10 Best Medical Image Annotation & Labeling Service Providers In 2024
Top image annotation services of 2024 streamline AI model training with precise data labeling, offering varied features and scalable options. This list explores providers like Labellerr, Scale AI, and Appen, known for accuracy, customization, and robust data security.
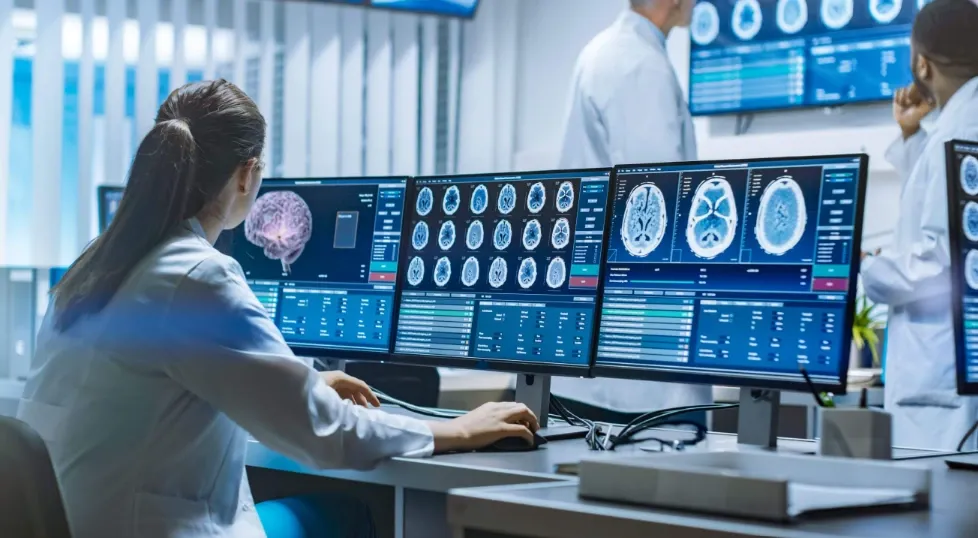
By providing specialised solutions for the advancement of healthcare, Medical Annotation Services has established an opportunity for itself in the medical picture annotation market. The business specialises in annotation services, which are critical for medical imaging algorithm training and for improving diagnosis and treatment planning.
The dedication of Medical Annotation Services to customisation is what makes it stand out; the team works closely with clients to comprehend their unique needs and makes sure that the annotated datasets produced are perfectly aligned with the complexities of their medical imaging projects. The service providers helps medical facilities and research groups use annotated data to build AI models that enhance patient outcomes. It emphasises precision and scalability in this process.
We'll explore the top 10 medical imaging annotation and labeling service providers, with a focus on easy-to-understand language. These companies play a crucial role in advancing healthcare by specializing in annotation services, vital for training medical imaging algorithms and enhancing the accuracy of diagnosis and treatment planning.
Table of Contents
- Labellerr
- Supervisely
- Labelbox
- Cogito Tech
- Anolytics
- Alegion
- Ango Hub (by iMerit)
- Basic AI
- V7
- Kili
- Conclusion
- Frequently Asked Questions
Top 10 Medical Image Annotation Service Providers
1. Labellerr
Labellerr has emerged as a trusted partner in healthcare innovation, offering comprehensive data annotation services that transform raw data into valuable insights. The platform plays a vital role in advancing personalized medicine by providing accurate and reliable annotation services tailored specifically to the healthcare industry.
Key Features of Labellerr in Medical Image Annotation
1. Precision in Medical Imaging: Labellerr excels in providing precise annotations for medical images, contributing to more accurate diagnosis and therapy planning. The platform's annotation services likely encompass tasks such as identifying and outlining structures in various medical imaging modalities, including MRI, CT scans, X-rays, and more.
2. Electronic Health Record (EHR) Analysis: Labellerr offers annotation services for Electronic Health Records (EHRs), streamlining the organization and annotation of patient data for quick access and analysis. The platform's capabilities in EHR analysis support improved data management and accessibility in healthcare settings.
3. Telemedicine Support: Labellerr facilitates the annotation of data from distant patients, enabling remote monitoring and diagnosis in telemedicine applications. The platform likely supports the annotation of medical data, allowing healthcare professionals to make informed decisions based on annotated information from remote patients.
4. Precision Medicine with Genetic Data Annotation: Labellerr contributes to precision medicine by performing genetic data annotation, enabling the creation of individualized treatment strategies. The platform's services in genetic data annotation likely involve identifying and marking specific genetic features for personalized medical interventions.
5. Clinical Trials and Research: Labellerr simplifies the analysis of clinical trial data, supporting researchers and healthcare professionals in making informed decisions. The platform's annotation services likely aid in organizing and annotating data generated from clinical trials, ensuring accurate and reliable information for research purposes.
6. Healthcare IoT Data Annotation: Labellerr's annotation services extend to Healthcare IoT data, providing real-time analysis and individualized health insights. The platform likely supports the annotation of data from IoT devices in healthcare, contributing to the development of advanced AI models for health monitoring.
7. Building a Computer Vision Model in Healthcare: Labellerr outlines a simple three-step process for building a computer vision model in healthcare, emphasizing the identification of issues, gathering and labeling images for training, and continuous enhancement and scaling of the model.
8. Maximizing the Utility of Medical Images: Labellerr provides guidance on maximizing the utility of medical images, including the use of public datasets, integration of labeling and training processes, incorporation of augmentations, and prioritization of privacy and security.
Labellerr's medical image annotation service appears to be a comprehensive solution, leveraging cutting-edge AI technology to annotate and organize healthcare data effectively. The platform's commitment to precision, privacy, and competence positions it as a reliable partner in advancing AI applications in the healthcare sector.
2. Supervisely
Supervisely is a versatile data annotation platform that offers comprehensive solutions for medical image annotation. The platform supports a variety of annotation tasks, including segmentation and labeling, making it suitable for diverse medical imaging projects.
What sets Supervisely apart is its user-friendly interface, allowing both technical and non-technical users to efficiently annotate medical images. The platform also facilitates collaboration, enabling teams to work seamlessly on annotation projects. Supervisely's commitment to flexibility and ease of use positions it as a valuable tool for healthcare organizations aiming to annotate medical images for training machine learning models.
3. Labelbox
Labelbox is a well-known data annotation platform that caters to various industries, including healthcare. With a focus on scalability, Labelbox provides solutions for medical image annotation, supporting tasks such as object detection, segmentation, and classification. The platform's versatility allows users to create labeled datasets tailored to specific medical imaging applications. Labelbox also incorporates features for collaboration and quality control, ensuring the accuracy and consistency of annotated data.
Healthcare organizations leveraging Labelbox benefit from its robust infrastructure and the ability to streamline the annotation process for developing AI models in medical imaging.
Before selecting a medical image annotation service provider, it is essential to evaluate factors such as the platform's user interface, scalability, collaboration features, and adherence to data security and privacy standards.
Additionally, checking for recent customer reviews and staying informed about the latest developments in the industry will contribute to making well-informed decisions based on the specific needs of your medical imaging projects.
4. Cogito Tech
Cogito Tech is a well-known brand in the medical image annotation services industry, renowned for its proficiency in artificial intelligence and computer vision. The business provides all-inclusive medical image annotation tools that support the development of machine learning models for applications including organ segmentation, disease categorization, and tumour identification.
Cogito Tech is unique in that it is dedicated to offering accurate and efficient annotations of the highest calibre. An expert team of professionals with a thorough understanding of medical imaging intricacies handles the annotation process. Cogito Tech offers services in radiology and pathology, among other medical fields, which helps improve patient care and diagnostic precision.
5. Anolytics
Anolytics stands out as a leading medical image annotation service provider, offering a comprehensive suite of annotation solutions tailored for the healthcare industry. Specializing in tasks such as segmentation, classification, and object detection, Anolytics leverages advanced annotation techniques to enhance the accuracy and efficiency of machine learning models in medical imaging. The company employs a team of skilled annotators with expertise in radiology and pathology, ensuring a deep understanding of the nuances within medical images. Anolytics places a strong emphasis on quality control, employing rigorous validation processes to guarantee the precision of annotated datasets. Their commitment to regulatory compliance, including adherence to HIPAA standards, makes them a reliable choice for healthcare organizations seeking top-notch annotation services for the development of robust AI algorithms.
6. Alegion
Alegion is a comprehensive data annotation platform that extends its services to the healthcare sector, providing medical image annotation solutions. The platform is designed to address the unique needs of medical imaging projects, offering tools and workflows for tasks like tumor identification, organ segmentation, and disease classification.
Alegion stands out for its flexibility and scalability, allowing healthcare organizations to manage and scale their image annotation projects efficiently. Moreover, the platform emphasizes quality control mechanisms to ensure the accuracy and reliability of annotated datasets, crucial for training machine learning models in the medical domain. Alegion's approach makes it a valuable partner for institutions seeking a robust and customizable solution for medical image annotation.
7. Ango Hub (by iMerit)
Ango Hub, by iMerit, stands out as a robust medical image annotation platform catering to the intricate needs of healthcare professionals and researchers. Positioned as an expert annotation platform, Ango Hub provides a tailored interface for medical experts, facilitating the meticulous labeling and marking of features within diverse medical imaging modalities such as MRI, CT, Cellular, Dermo, Ultrasound, and X-Ray data. The platform's annotation tools are likely designed to streamline the annotation process, offering precision and efficiency in creating annotated datasets.
Ango Hub is expected to encompass pathology-specific annotation tools, addressing the unique requirements of various medical specialties. This specialization ensures that medical professionals can accurately annotate datasets, laying the foundation for the development of artificial intelligence models that excel in diagnosing specific conditions or interpreting distinct anatomical structures. The platform's emphasis on pathology-specific tools underscores its commitment to delivering tailored solutions in the dynamic landscape of medical imaging.
8. Basic AI
BasicAI's comprehensive image and video annotation services, equipped with precise bounding box annotation, polygon and poly-line annotation, key point annotation, skeleton annotation, segmentation, and sensor fusion annotation, are versatile and adaptable to diverse domains. In the context of medical image annotation, these services could be instrumental in advancing healthcare technologies and research.
Medical Image Annotation Capabilities:
(i) Bounding Box Annotation: Ideal for medical object detection and localization tasks, such as identifying and tracking specific structures in radiological images.
(ii) Polygon and Poly-line Annotation: Essential for outlining anatomical structures in medical imaging, contributing to applications like semantic segmentation and accurate organ delineation.
(iii) Key Point Annotation: Supports the identification of anatomical landmarks, enabling applications like pose estimation for surgical procedures or research in biomechanics.
(iv) Skeleton Annotation: Crucial for mapping interconnected skeletons, which can be valuable in analyzing complex poses in medical scenarios, aiding in fields like biomechanics or physical therapy.
(v) Segmentation: Critical for pixel-level masks in medical images, facilitating precise delineation of tissues or abnormalities for improved diagnostic accuracy.
(vi) Sensor Fusion Annotation: Relevant for integrating data from different medical imaging sensors, offering a comprehensive view for applications like 3D reconstruction or treatment planning.
9. V7
V7 stands out as a powerful AI training data platform that specializes in image annotation services, catering to the diverse needs of computer vision model development. The platform supports annotation for various types of data, including images, videos, and volumetric series, offering a comprehensive solution for a range of applications.
The platform's versatility extends to medical imaging, allowing users to annotate medical file formats such as DICOM and NIfTI. V7's support for instance segmentation, automatic label interpolations in videos, and object detection and tracking in videos with instance IDs underscores its adaptability to diverse use cases.
One of V7's notable features is its pixel-perfect automatic data labeling, allowing users to achieve accurate annotations swiftly. The platform provides an intuitive dataset management system, enabling users to handle and organize their annotated data seamlessly. With a one-click model training option, V7 emphasizes simplicity in deploying trained models for computer vision applications.
The advanced auto-labeling capabilities of V7 significantly contribute to reducing data annotation time by an impressive 90%. This efficiency is complemented by team collaboration tools, fostering a collaborative environment for annotators and other stakeholders. V7 supports multiple annotation types, including bounding boxes, polygons, key points, and auto-segmentation tools, all powered by machine learning models.
10. Kili
Kili's versatile data annotation platform could be well-suited for applications in the healthcare industry, including medical image annotation. Kili Technology supports multiple annotation types, encompassing text, image, video, and audio. This diversity implies that the platform can cater to the unique requirements of medical image annotation, which often involves intricate labeling of structures in various medical imaging modalities such as MRI, CT scans, X-rays, and more.
The platform's focus on simplifying data-centric AI workflows is particularly valuable for medical machine learning (ML) and DataOps teams. In healthcare, where the quality and accuracy of annotated data are critical for the success of AI projects, Kili's capabilities may streamline the process of creating and managing annotation projects. This could be especially relevant in medical research, diagnostic AI, and treatment planning.
In the context of medical image annotation, Kili could be utilized to annotate and manage datasets for tasks such as medical image segmentation, object detection, and anatomical structure labeling.
Kili offers a free tier for individuals, which could be beneficial for researchers or small teams in the medical field working on AI projects. For larger healthcare enterprises with more extensive data annotation needs, corporate/enterprise plans may provide scalability and additional features, ensuring flexibility for healthcare organizations of varying sizes.
Kili Technology emphasizes that the foundation of better AI is excellent data. In the medical domain, where precision and accuracy are paramount, Kili's commitment to high-quality data annotation aligns with the requirements for developing reliable and effective AI models in healthcare applications.
Conclusion
The demand for medical imagine annotation services is always changing, which makes it crucial for using AI to improve healthcare. Labellerr, Supervisely, Labelbox, Cogito Tech, Anolytics, Alegion, Ango Hub, Basic AI, V7, and Kili are among the top 10 providers; each having particular advantages. These platforms are essential for improving diagnosis and treatment planning accuracy. They are advancing precision medical imaging, assisting with electronic health record analysis, or supporting precision medicine. These providers' dedication to precision, scalability, and customization makes them valuable partners for healthcare organizations looking to use annotated data for ground-breaking research, better patient outcomes, and creative AI solutions, especially as the demand for AI in healthcare continues to rise.
Read our other listicles:
1. Top 10 Best Video Annotation & Labeling service providers in 2024
2.Top 10 Best Image Annotation & Labeling Service providers in 2024
Frequently Asked Questions
1. What are the use cases for medical annotations & labeling?
Medical annotations and labeling have diverse use cases in healthcare, including precise identification and delineation of anatomical structures in medical images for applications such as diagnostic imaging, surgical planning, and treatment monitoring. Annotations play a crucial role in training machine learning models for tasks like pathology detection, organ segmentation, and disease classification, thereby enhancing the accuracy and efficiency of automated diagnostic systems.
Additionally, annotations are integral to medical research, clinical trials, and the development of AI-driven solutions for personalized medicine, contributing to advancements in healthcare through data-driven insights and improved patient care.
2. How to improve data quality in medical annotation?
Improving data quality in medical annotation involves implementing rigorous quality control measures, ensuring consistency in annotations through standardized guidelines, and fostering continuous feedback loops between annotators and domain experts. Regular training sessions for annotators on medical nuances and using advanced annotation tools contribute to enhanced accuracy.
Leveraging automated validation checks and incorporating a multi-annotator approach for consensus validation further strengthens the reliability of annotated datasets. Prioritizing data privacy and security measures, adhering to industry standards, and continuously updating annotation processes based on evolving medical knowledge are essential steps in ensuring and improving the overall quality of medical annotations.
3. What are medical image annotation tools?
Medical image annotation tools are specialized software applications designed for labeling and marking anatomical structures, abnormalities, or other clinically relevant features in medical images. These tools facilitate the creation of annotated datasets essential for training and validating machine learning models in healthcare applications.
Medical image annotation tools vary in functionalities, offering features such as bounding box annotation, segmentation, key point identification, and anatomical structure labeling. They play a crucial role in tasks like medical image analysis, diagnostics, and research by providing accurate and standardized annotations for improved model accuracy and clinical decision support.
Book our demo with one of our product specialist
Book a Demo